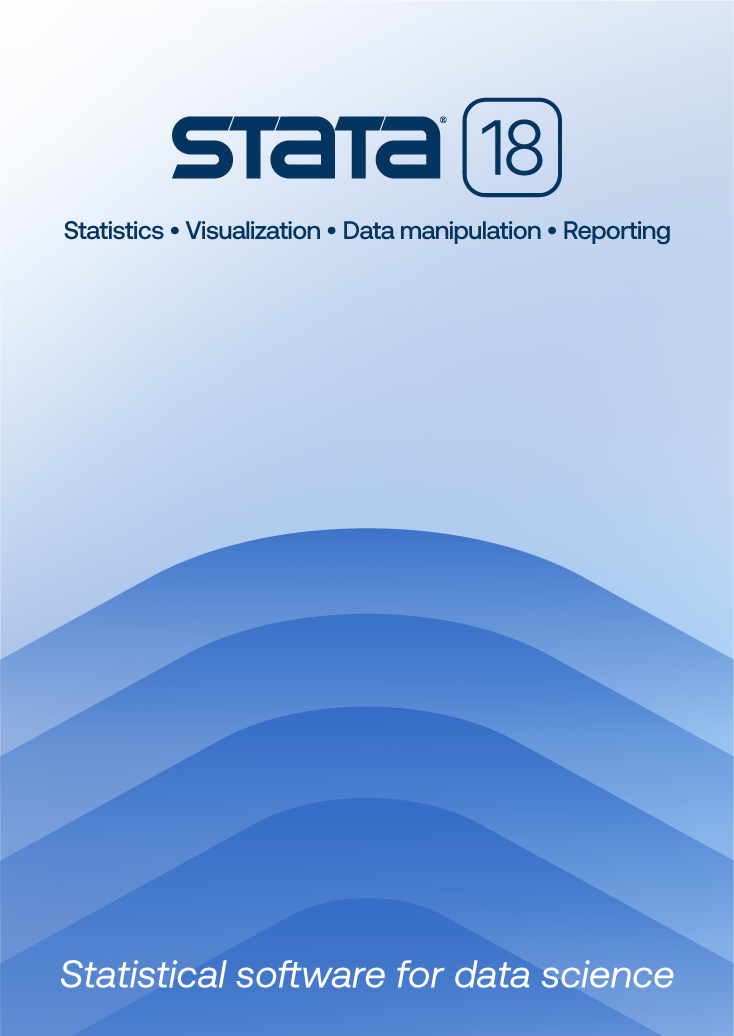
Stata provides statistical solutions developed by StataCorp, and it provides programming tools for those who want to develop their own solutions. There are two Stata programming languages: ado, which is easy to use, and Mata, which performs numerical heavy lifting. And Stata is integrated with Python.
New Mata class LinearProgram() solves linear programs. It uses Mehrotra's (1992) interior-point method, which is faster for large problems than the traditional simplex method.
Here's a linear program that we will solve:
maximize 5*x1 + 3*x2 (objective function) subject to -x1 + 11*x2 = 33 (equality constraints) 0.5*x1 - x2 <= -3 (inequality constraints) 2*x1 + 14*x2 <= 60 2*x1 + x2 <= 14.5 x1 - 0.4*x2 <= 5 and x1 >= 0 (bounds) x2 >= 0
The mathematical solution to this toy problem is
x1 = 0 x2 = 3 objective function = 5*0 + 3*3 = 9
Let's use LinearProgram() to solve it. To do that, you
A program to fit the above model might read:
real vector solveproblem() { class LinearProgram scalar lp real scalar value_of_objective_function real vector x // We do not bother to declare the other variables // used in this program. We have -mata strict off-. // Mata will declare the undeclared variables // automatically. // ---------------------------------------------------- // coefficients coefs = ( 5 , 3) // equality constraints eq_lhs = (-1 , 11) ; eq_rhs = 33 // inequality constraints ineq1_lhs = ( 0.5, -1) ; ineq1_rhs = -3 ineq2_lhs = ( 2 , 14) ; ineq2_rhs = 60 ineq3_lhs = ( 2 , 1) ; ineq3_rhs = 14.5 ineq4_lhs = ( 1 ,-.4) ; ineq4_rhs = 5 // bounds lower = (0, 0) upper = (., .) // ---------------------------------------------------- // combine inequality // constraints ineq_lhs = (ineq1_lhs \ ineq2_lhs \ ineq3_lhs \ ineq4_lhs ) ineq_rhs = (ineq1_rhs \ ineq2_rhs \ ineq3_rhs \ ineq4_rhs ) // ---------------------------------------------------- // set class lp.setCoefficients(coefs) lp.setEquality( eq_lhs, eq_rhs) lp.setInequality(ineq_lhs, ineq_rhs) lp.setBounds(lower, upper) // ---------------------------------------------------- // solve linear program value_of_objective_function = lp.optimize() x = lp.parameters() // ---------------------------------------------------- // done // Return solution as vector (x, value_of_objective_function) return((x, value_of_objective_function)) }
Running this program results in
x1 = 9.895354557e-11 x2 = 3.0000000000264 objective_function = 9.000000000574
x1 and x2 are accurate to 11 digits, and the objective, to 10. If we needed more accuracy, you could reset lp.setTol(). Its default is 1e-8.
Mehrotra, S. 1992. On the implementation of a primal-dual interior point method. SIAM Journal on Optimization 2: 575–601.
Learn more about Stata's Mata programming features.
Read more about linear programming in the Mata Reference Manual; see [M-5] LinearProgram().