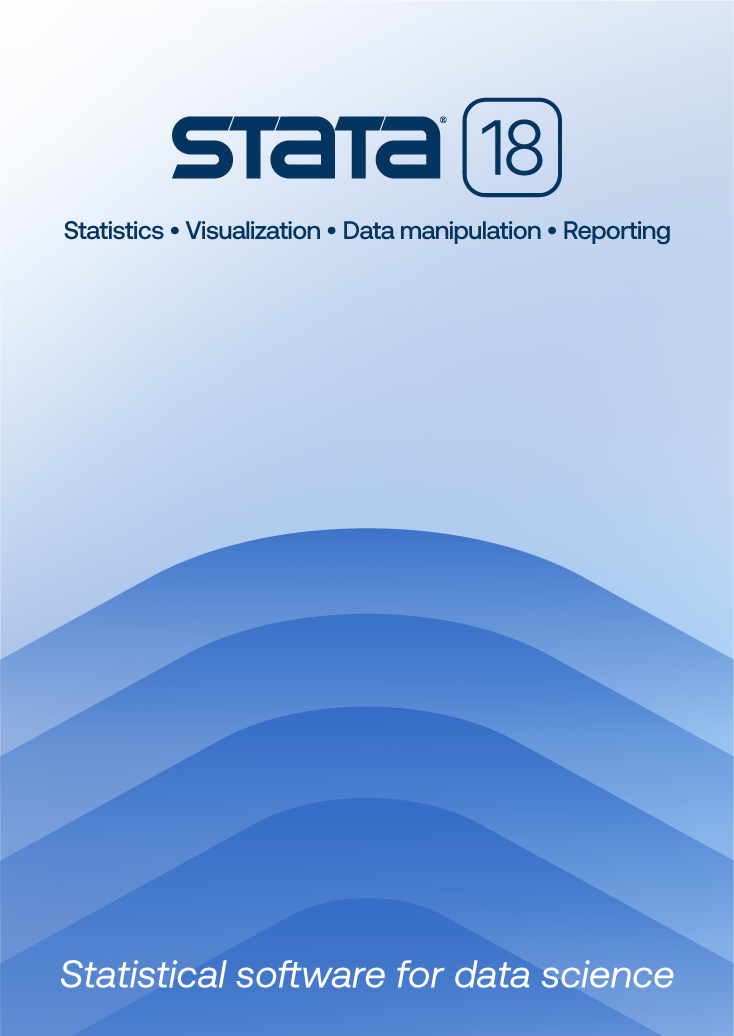
10:15–10:45 | resultssets in resultsframes in Stata 16-plus
Abstract:
A resultsset is a Stata dataset created as output by a Stata
command.
It may be listed and saved in a disk file or written over
an existing dataset in memory and (in Stata Versions 16 or
higher) written to a data frame (or resultsframe) in the
memory, without damaging any existing data frames. Commands
creating resultssets include parmest,
parmby, xcontract, xcollapse,
descsave, xsvmat, and xdir. Commands useful
for processing resultsframes include xframeappend,
fraddinby, and invdesc. I survey the ways in
which resultsset processing has been changed by
resultsframes.
Additional information:
Roger Newson
King's College London
|
|||
10:45–11:05 | A suite of Stata programs for analyzing simulation studies
Abstract:
Simulation studies are used in a variety of disciplines to
evaluate the properties of statistical methods.
Simulation studies involve creating data by random sampling,
typically from known probability distributions, with the aim of
assessing the robustness and accuracy of new statistical
techniques by comparing them with some known truth. I introduce
the siman suite for the analysis of simulation results.
siman is a set of Stata programs that offers data manipulation,
analysis, and graphics to process, explore, and visualize the
results of simulation studies.
siman expects a sensibly structured dataset of simulation study estimates, with input variables being in ‘long’ or ‘wide’ format, string, or numeric. The estimates data can be reshaped by siman reshape to enable data exploration. The key commands include siman analyse to estimate and tabulate performance; graphs to explore the estimates data (siman scatter, siman swarm, siman zipplot, siman blandaltman, siman comparemethodsscatter); and a variety of graphs to visualize the performance measures (siman nestloop, siman lollyplot, siman trellis) in the form of scatterplots, swarm plots, zip plots, Bland–Altman plots, nested-loop plots, lollyplots, and trellis graphs (Morris, White, and Crowther 2019). References:Morris, T. P., I. R. White, and M. J. Crowther. 2019. Using simulation studies to evaluate statistical methods. Statistics in Medicine 38: 2074–2102.
Additional information:
Ella Marley-Zagar
University College London
|
|||
11:05–11:35 | Cook’s distance measures for panel-data models
Abstract:
Influential observations in regression analysis are data points
whose deletion has a large impact on the estimated coefficients.
The usual diagnostics for assessing the influence of each
data point are designed for least-squares regression and
independent observations and are not appropriate when estimating
panel-data models.
The purpose of this presentation is to describe a new command, cooksd2, that extends the traditional Cook’s (1977) distance measure to determine the influence of each data point when applying the fixed-, random-, and between-effects regression estimators. The approach is based on the framework developed by Christensen, Pearson, and Johnson (1992) and also reports the influence of an entire subject or group of data points following the methods described by Banerjee and Frees (1997). References:Cook, R. D. (1977). Detection of influential observation in linear regression. Technometrics 19: 15–18. Banerjee, M., and E. W. Frees. (1997). Influence diagnostics for linear longitudinal models. Journal of the American Statistical Association 92: 999 1005. Christensen, R., L. M. Pearson, and W. Johnson. 1992. Case-deletion diagnostics for mixed models. Technometrics 34: 38–45.
Additional information:
David Vincent
David Vincent Economics
|
|||
11:35–12:35 | Bayesian multilevel modeling
Abstract:
In multilevel or hierarchical data, which include longitudinal,
cross-sectional, and repeated-measures data, observations belong
to different groups.
Groups may represent different levels of hierarchy, such as
hospitals, doctors nested within hospitals, and patients nested
within doctors nested within hospitals. Multilevel models
incorporate group-specific effects in the regression model and
assume that they vary randomly across groups according to some a
priori distribution, commonly a normal distribution. This
assumption makes multilevel models natural candidates for
Bayesian analysis. Bayesian multilevel models additionally
assume that other model parameters such as regression
coefficients and variance components—variances of
group-specific effects—are also random.
In this presentation, I will discuss some of the advantages of Bayesian multilevel modeling over the classical frequentist estimation. I will cover some basic random-intercept and random-coefficients modeling using the bayes: mixed command. I will then demonstrate more advanced model fitting by using the new-in-Stata-17 multilevel syntax of the bayesmh command, including multivariate and nonlinear multilevel models.
Additional information:
Yulia Marchenko
StataCorp
|
|||
1:40–2:00 | Bias-corrected estimation of linear dynamic panel-data models
Abstract:
In the presence of unobserved group-specific heterogeneity, the
conventional fixed-effects and random-effects estimators for
linear panel-data models are biased when the model contains a
lagged dependent variable and the number of time periods is
small.
I present a computationally simple bias-corrected estimator with
attractive finite-sample properties, which is implemented in the
new xtdpdbc Stata package. The estimator relies neither
on instrumental variables nor on specific assumptions about the
initial observations. Because it is a method of moments
estimator, standard errors are readily available from asymptotic
theory. Higher-order lags of the dependent variable can be
accommodated as well. A useful test for the correct model
specification is the Arellano–Bond test for residual 3
autocorrelation. The random-effects versus fixed-effects
assumption can be tested using a Hansen overidentification test
or a generalized Hausman test. The user can also specify a
hybrid model, in which only a subset of the exogenous regressors
satisfies a random-effects assumption.
Contributor:
Jörg Breitung
University of Cologne
Additional information:
Sebastian Kripfganz
University of Cologne
|
|||
2:00–2:30 | Impact of proximity to gas production activity on birth outcomes across the US
Abstract:
Despite mounting evidence on the health effects of natural gas
development (NGD), including hydraulic fracturing
(“fracking”), existing research has been constrained to
high-producing states, limiting generalizability.
We examined the impacts of prenatal exposure to NGD production
activity in all gas-producing US states on birth outcomes
overall and by race/ethnicity. Mata routines were developed to
link 185,376 NGD production facilities in 28 U.S. states and their
distance-weighted monthly output with county population
centroids via geocoding. These data were then merged with
2005–2018 county-level microdata natality files on 33,849,409
singleton births from 1,984 counties in 28 states, using
nine-month county-level averages of NGD production by both
conventional and unconventional production methods, based on
month/year of birth.
Linear regression models were fit to examine the impact of prenatal exposure to NGD production activity on birthweight and gestational age, while logistic regression models were used for the dichotomous outcomes of low birthweight (LBW), preterm birth, and small for gestational age (SGA). Overall, prenatal exposure to NGD production activity increased adverse birth outcomes. We found that a 10% increase in NGD production in a county decreased mean birthweight by 1.48 grams. A significant interaction by race/ethnicity revealed that a 10% increase in NGD production decreased birthweight for infants born to Black women by 10.19 grams and Asian women by 2.76 grams, with no significant reductions in birthweight for infants born to women from other racial/ethnic groups. Although effect sizes were small, results were highly consistent. NGD production decreases infant birthweight, particularly for those born to minoritized mothers.
Contributors:
Hailee Schuele
Philip J. Landrigan
Summer Sherburne Hawkins
Boston College
Additional information:
Christopher F. Baum
Boston College
|
|||
2:30–3:00 | Estimating compulsory schooling impacts on labor market outcomes in Mexico
Abstract:
This study estimates the impacts on labor market outcomes of
the 1993 compulsory schooling reform in Mexico.
A well-known problem in this analysis is the endogeneity between
schooling and labor market outcomes due to unobservable
characteristics that could jointly determine them. There is also
heterogeneity in the empirical evidence of the effectiveness of
such schooling policies among developing and developed countries,
perhaps because of the different contexts and identification
strategies used. Some studies use instrumental-variables (IV)
and difference-in-differences (D-i-D) methods to tackle
endogeneity issues. Most analyses use a regression discontinuity
design (RDD) approach with different order polynomials of the
year of birth (for example, cubic or quartic order), whereas few
studies use birth month for more accurate and robust
estimates because it allows more schooling variation within a year.
The impact of the Mexican policy is analyzed in this study through a fuzzy RDD approach with the use of Stata for the period 2009 to 2017. It addresses endogeneity by exploiting the age cohort discontinuities in birth month, for more robust estimation, as an exogenous source of education variation. Fuzzy RDD then compares schooling and labor market outcomes among the birth cohorts exposed with those not exposed to the reform. The fuzziness accounts for the imperfect compliance by using the random assignment of the exposure to the policy. Stata allows plotting discontinuity graphs between cohorts as well as the McCrary test to validate the use of this methodology. It also facilitates parametric and nonparametric analyses. The empirical evidence suggests that the 1993 compulsory schooling law, although raising average school attendance, was an insufficient policy to impact labor market outcomes in Mexico. The analysis contributes to the limited literature on the returns to compulsory schooling that uses a rigorous RDD methodology in developed and developing countries.
Additional information:
Erendira Leon Bravo
University of Westminster
|
|||
3:30–4:00 | Bias-adjusted three-step latent class analysis using R and the gsem command in Stata
Abstract:
In this presentation, we will describe a means to perform bias-adjusted
latent class analysis using three-step methodology.
This method is often performed using MPLUS, LATENT GOLD, or
specific functions in Stata. Here we will describe a novel means
to perform this analysis using the poLCA package in R to perform
the first two steps and the gsem command in Stata to
perform the third step. This methodology is applied to a case
study involving performing causal analysis by integrating
inverse probability of treatment weights into the methodology.
We will also demonstrate how to obtain estimates of the average
causal effect of exposure on a latent class using the
margins command with robust standard errors. Our aim is
to broaden awareness of three-step latent class methods and
causal analysis and offer means to perform this methodology for
users of R, for which there currently is little software
available.
Contributor:
Bianca de Stavola
UCL
Additional information:
Daniel Tompsett
UCL
|
|||
4:00–4:30 | Distributed lag nonlinear models (DLNMs) in Stata
Abstract:
The distributed lag nonlinear models (DLNMs) represent a
modeling framework to flexibly describe associations showing
potentially nonlinear and delayed effects in time-series data.
This methodology rests on the definition of a crossbasis, a
bidimensional functional space combining two sets of basis
functions that specify the relationships in the dimensions of
predictor and lags, respectively. DLNMs have been widely used in
environmental epidemiology to investigate the short-term
associations between environmental exposures, such as weather
variables or air pollution, and health outcomes, such as
mortality counts or disease-specific hospital admissions. We
implemented the DLNMs framework in Stata through the crossbasis
command to generate the basis variables that can be fit in a
broad range of regression models. In addition, the postestimation
commands crossbgraph and crossbslices
allow interpreting the results, emphasizing graphical
representation, after the regression model fit. We present an
overview of the capabilities of these new community-contributed
commands and describe the practical steps to fit and interpret
DLNMs with an example of real data to represent the relationship
between temperature and mortality in London during the period
2002–2006.
Contributors:
Ben Armstrong
Antonio Gasparrini
Spanish Research Council (CSIC) and LSHTM
Additional information:
Aurelio Tobias
Spanish Research Council (CSIC) and LSHTM
|
|||
4:30–5:15 | Advanced data visualizations with Stata: Part III
Abstract:
The presentation will showcase recent developments in complex
data visualizations with Stata.
These include various types of polar plots, for example, spider
plots, sunburst charts, circular bar graphs, and various
visualizations with spatial data, including bivariate maps,
gridded waffle charts, and map clippings. Updates for several
Stata packages, including joyplot, bimap,
streamplot, and clipgeo, will be presented, and
suggestions for improving Stata’s graph capabilities will be
discussed.
Additional information:
Asjad Naqvi
Austrian Institute for Economic Research (WIFO), International Institute for Applied Systems Analysis (IIASA), and Vienna University of Economics and Business (WI)
|
|||
9:10–9:40 | Grinding axes: Axis scales, labels, and ticks
Abstract:
This is a roundup of not quite utterly obvious tips and tricks
for graph axes, using both official and community-contributed
commands.
Ever needed a logarithmic scale but found default labels
undesirable?
Community-contributed commands mentioned will include mylabels, myticks, nicelabels, niceloglabels, qplot, and transplot.
Additional information:
Nick Cox
Durham University
|
|||
9:40–10:00 | Exchangeably weighted bootstrap schemes
Abstract:
The exchangeably weighted bootstrap is one of the many variants
of bootstrap resampling schemes.
Rather than directly drawing observations with replacement from
the data, weighted bootstrap schemes generate vectors of
replication weights to form bootstrap replications. Various ways
to generate the replication weights can be adopted, and some
choices bring practical computational advantages. This
presentation demonstrates how easily such schemes can be
implemented and where they are particularly useful. It also
introduces the exbsample command, which facilitates their
implementation.
Additional information:
Philippe Van Kerm
LISER and University of Luxembourg
|
|||
10:00–10:30 | Improving fitting and predictions for flexible parametric survival models
Abstract:
Flexible parametric survival models have been available in Stata
since 2000 with Patrick Royston’s stpm command.
I developed stpm2 in 2008, which added various extensions.
However, the command is old and does not take advantage of some
of the features Stata has added over the years. I will introduce
stpm3, which has been completely rewritten and adds a
number of useful features, including
Additional information:
Paul Lambert
University of Leicester and Karolinska Institutet
|
|||
11:00–11:30 | sttex: A new dynamic document command for Stata and LaTeX
Abstract:
In this presentation, I will introduce a new command for
processing a dynamic LaTeX document in Stata, for example, a
document containing both LaTeX paragraphs and Stata code.
A key feature of the new command is that it tracks changes in
the Stata code and executes the code only when needed, allowing
for an efficient workflow. The command is useful for creating
automated statistical reports, writing articles with data
analysis, preparing slides for a methods course or a conference
talk, or even writing a complete textbook with examples of
applications.
Additional information:
Ben Jann
University of Bern
|
|||
11:30–12:30 | Custom estimation tables
Abstract:
This presentation illustrates how to construct custom tables from one or more estimation commands.
I demonstrate how to add custom labels for significant coefficients and make targeted style
edits to cells in the table using the following commands:
Additional information:
Jeff Pitblado
StataCorp
|
|||
1:30–2:00 | The impact of a government pay reform in Mexico on the public sector wage gap
Abstract:
The 2018 federal pay reform on the remuneration of public
servants in Mexico is used to exploit its impacts on the
public–private sector wage gap across the unconditional
wage distribution in a developing country context.
This policy uses both payment cuts and freezes for public sector
workers.
Using cross-sectional data from 2017 to 2019, both the mean and unconditional quantile (UQ) regression models within a difference-in-differences (DID) framework are fit. Stata allows the use of UQ regressions based on the recentred influence function (RIF) to center the IF around the statistic of interest (for example, the population mean ‘µ’, 10 E[Y]) and not zero (for example, reweighting the observations) for generating the RIF quantiles. The RIF average effects are interpreted at different quantiles of the unconditional wage distribution (for example, the 5th or 95th percentiles or other intermediate quantiles). Then the DID approach implemented through Stata provides the effects of the reform before and after the policy intervention. It also deals with the endogeneity of employment selection by accounting for the differences in the unobservable effects of the public–private employment sector selection pretreatment. Posttreatment, such unobservables are differenced out to mitigate the concerns about potential selection bias. Robustness checks are also executed with Stata, such as cohort fixed effects with pseudopanel dataset, a two-step model within a Heckman framework, the Hansen J-statistic to test orthogonality, an IV-based model, an individual-level fixed-effects (FE) model with a panel dataset, and a placebo in-time test. Although there is some evidence that public sector employees anticipated the introduction of the policy, it reduced the public sector pay gap strongly among the lower-paid workers of the unconditional pay distribution. The UQ effects of this policy change on the public–private sectoral wage gap contribute to the limited literature for both developed and developing countries.
Contributor:
Barry Reilly
University of Sussez
Additional information:
Erendira Leon Bravo
University of Westminster
|
|||
2:00–2:30 | Illuminating the factor and dependence structure in large panel models
Abstract:
In panel models, a precise understanding about the number of
common factors and dependence across the cross-sectional
dimension is key for any applied work.
This presentation will give an overview about how to estimate
the number of common factors and how to test for cross-sectional
dependence. It does so by presenting two community-contributed
commands: xtnumfac and xtcd2. xtnumfac
implements 10 different methods to estimate the number of
factors, among them the popular methods by Bai and Ng (2002) and
Ahn and Horenstein (2013). The degree of cross-section dependence
is investigated using xtcd2. xtcd2 implements
three different tests for cross-section dependence based on
Pesaran (2015), Juodis and Reese (2021), and Pesaran and Xie (2021).
The presentation includes a review of the theory, a discussion
of the commands, and empirical examples.
Additional information:
Jan Ditzen
Free University of Bozen-Bolzano
|
|||
2:30–3:00 | mixrandregret: A command for fitting mixed random regret minimization models using Stata
Abstract:
This presentation describes the mixrandregret command,
which extends the randregret command
(Gutiérrez-Vargas, Meulders and Vandebroek. 2021.
The Stata Journal 21: 626–658), incorporating
random coefficients for random regret minimization (RRM) models.
The command can fit a mixed version of the classic RRM model
introduced in Chorus (European Journal of Transport and
Infrastructure Research. 2010. 10: 181–196). It allows
the user to specify a combination of fixed and random
coefficients. In addition, the users can specify normal and
log-normal distributions for the random coefficients using the
commands’ options. Finally, the models are fit using
simulated maximum-likelihood procedures using numerical
integration to simulate the models’ choice probabilities.
Contributors:
Ziyue Zhu
Martina Vandebroek
KU Leuven
Additional information:
Álvaro A. Gutiérrez-Vargas
KU Leuven
|
|||
3:30–4:30 | Open panel discussion with Stata developers
Contribute to the Stata community by sharing your feedback with StataCorp's developers. From feature improvements to bug fixes and new ways to analyze data, we want to hear how Stata can be made better for our users.
|
Tim Morris MRC, Clinical Trials Unit, UCL |
Rachael Hughes University of Bristol |
The logistics organizer for the 2022 UK Stata Conference is Timberlake Consultants, the Stata distributor to the United Kingdom and Ireland, France, Spain, Portugal, the Middle East and North Africa, Brazil, and Poland.
View the proceedings of previous Stata Conferences and Users Group meetings.