The third Swiss Stata Conference took place virtually on 19 November 2020.
Proceedings
10:30–11:00 | randregret: A command for fitting random regret minimization models using Stata
Abstract:
In this presentation, we describe the randregret command, which
implements a variety of random regret minimization (RRM) models. The
command allows the user to apply the classic RRM model (Chorus 2010),
the generalized RRM model (Chorus 2014), and also the mu-RRM and pure
RRM models (Van Cranenburgh, Guevara, and Chorus 2015).
We illustrate
the usage of the randregret command using stated choice data on
route preferences. The command offers robust and cluster standard-error
correction using analytical expressions of the score functions. It also
offers likelihood ratio tests, which can be used to assess the relevance
of a given model specification. Finally, predicted probabilities from
each model can be easily computed using the randregretpred
postestimation command.
References: Chorus, C. G. 2010. A new model of random regret minimization. European Journal of Transport and Infrastructure Research 10(2). Chorus, C. G. 2014. A generalized random regret minimization model. Transportation Research Part B: Methodological 68: 224–238. Van Cranenburgh, S., C. A. Guevara, and C. G. Chorus. 2015. New insights on random regret minimization models. Transportation Research Part A: Policy and Practice 74: 91–109.
Contributors:
Michel Meulders
Martina Vandebroek
KU Leuven
Additional information: Álvaro A. Gutiérrez Vargas
KU Leuven
|
11:00–11:30 | How to assess the fit of multilevel logit models with Stata? A project in progress
Abstract:
Stata 16 includes the multilevel model for binary (melogit) and
ordinal logits (meologit). Unfortunately, except for the global Wald
test of the estimated fixed effects, both models do not provide any fit measure to
assess its practical significance. Therefore, I developed an ado-file to
calculate McFadden's and McKelvey and Zavoina's pseudo-R². It estimates the
intraclass correlation (ICC) of the dependent variable for the actual
sample to assess the maximum of the contextual effect, too. Since the
early 90s, a lot of Monte Carlo simulation studies (Hagle and Mitchell
1992; Veall and Zimmermann 1992, 1993, 1994); Windmeijer 1995; DeMaris
2002) proved that McKelvey and Zavoina's pseudo-R² is the best one to assess
the fit of binary and ordinal logit models. My ado-file calculates this
fit measure in two complementary ways: for the fixed effects
only and for the fixed and random effects together. The
estimation of McFadden's pseudo-R² uses two different zero models:
the random-intercept-only model (RIOM) knowing the contextual
units and the fixed-intercept-only model (FIOM) ignoring the
contextual units completely. For each of them, it calculates the global
likelihood-ratio χ² test statistic 1 whether all fixed effects or all
fixed and random effects are zero in the population. It performs two
global likelihood-ratio χ² tests for all fixed effects and all fixed
and random effects using a probability weight for each level. An
empirical study of drug consumption in European countries demonstrates
the usefulness of my fit_meologit_2lev.ado or fit_meologit_3lev.ado
files for multilevel binary and ordinal logit models.
Additional information: Wolfgang Langer
Martin Luther Universität Halle-Wittenberg
|
11:30–12:00 | xtbreak: estimating and testing break points in panel data in Stata
Abstract:
I present the new community-contributed command xtbreak, which allows
the empirical analysis of multiple structural changes in panel data. The
panels may be strongly cross-sectionally dependent, where
cross-sectional dependence takes the form of a multifactor structure in
the errors. The number of break points and their dates are treated as unknown.
The command includes the following two hypothesis tests. First,
a test of the null hypothesis of no breaks against a user-specified
number of breaks. Second, a test of the null hypothesis of k numbers of
breaks against the alternative of k+1 number of breaks. Once the number
of breaks has been specified, the command allows the estimation of the
break dates and the construction of confidence intervals for these
dates. The syntax and options of xtbreak are explained. Examples
for the estimation of the breakpoints and testing those are given.
Additional information: Jan Ditzen
Heriot Watt University
|
1:00–1:30 | acreg: Arbitrary correlation regression
Abstract:
In this presentation, we present acreg, a new Stata command that
implements the standard error correction proposed in Colella et al.
(2019). This command computes regression coefficients' standard errors,
accounting for dependence across arbitrary correlation structures in a
flexible way. Arbitrary here refers to the way units are correlated with
each other: we impose no restrictions so that our approach can be used
with a wide range of data. In particular, this command allows the
estimation of OLS and 2SLS coefficient correcting standard errors across
units in three environments: in a spatial setting using objects'
coordinates or distance between units, in a network setting starting
from the adjacency matrix, and in a multi-way cluster framework taking
multiple clustering variables as input. This command suits both
cross-sectional and panel databases. Distance and time cutoffs can be
specified by the user and linear decay in time and space are also
optional.
Contributors:
Rafael Lalive
Seyhun Orcan Sakalli
Mathias Thoenig
Université de Lausanne
Additional information: Fabrizio Colella
Université de Lausanne
|
1:30–2:00 | A new implementation of relative distribution methods in Stata
Abstract:
A relative distribution is defined as the distribution of the relative
ranks that the outcomes of one distribution take on in another
distribution. An example is the relative positions that women's wages
take on in the distribution of men's wages. In this presentation, I will
discuss a new implementation of relative distribution methods in Stata.
The new
command called reldistcan be used to estimate and plot
the relative density function (relative PDF), a histogram of the relative
distribution, or the relative distribution function (relative CDF), or
to estimate polarization indices and descriptive statistics of the
relative data. The command also supports decomposition of the relative
distribution by adjusting differences in location, scale, and shape or
differences in covariate distributions (using reweighting). Standard
errors are estimated based on influence functions and support complex
survey data.
Additional information: Ben Jann
Universität Bern
|
2:00–2:30 | Quantile and distribution regression in Stata: Algorithms, pointwise and functional inference
Abstract:
This presentation and the related commands offer fast estimation and
inference procedures for the linear quantile and distribution regression
models. First, qrprocess and drprocess implement new algorithms
that are much quicker than the built-in Stata commands, especially when a
large number of regressions or bootstrap replications must be
estimated. Second, the commands provide analytical estimates of the
variance–covariance matrix of the coefficients for several regressions
allowing for weights, clustering, and stratification. Third, in addition
to traditional pointwise confidence intervals, these commands also
provide functional confidence bands and tests of functional hypotheses.
Fourth, predict—called after either qrprocess or
drprocess—can
generate monotone estimates of the conditional quantile and distribution
functions obtained by rearrangement. Fifth, plotprocess conveniently
plots the estimated coefficients with their confidence intervals and
uniform bands.
Additional information: Blaise Melly
Universität Bern
|
3:00–3:30 | arhomme: A Stata implementation of the Arrellano/Bonhomme (2017) estimator for quantile regression with selection correction
Abstract:
Despite constituting a major theoretical breakthrough, the quantile
selection model of Arrellano and Bonhomme (2017, Econometrica, Vol. 85,
pp. 1–28) based on copulas has not found its way into many empirical
applications. We introduce the command arhomme, which implements
different variants of the estimator along with standard errors based on
subsampling. We illustrate the command by replicating the empirical
application in the original article and a related application in
Arrellano and Bonhomme (2017, Handbook of Quantile Regression, Ch. 13).
Contributor:
Pascal Erhardt
University of Tübingen
Additional information: Martin Biewen
University of Tübingen
|
3:30–4:00 | Conditional likelihood models for distributional regression analysis
Abstract:
This presentation illustrates the use of conditional likelihood models for
"distributional regression" analysis. Conditional likelihood models
impose specific parametric functional forms for an outcome variable of
interest—for example, normality, log-normality, Gamma distribution,
or more elaborate shapes—but allow the shape parameters to
vary with
covariates; for example, assuming normality and allowing both the mean
and the standard deviation to vary with a vector of explanatory
variables. Drawing on examples from income distribution analyses, I show
how such models are fit in Stata, relying on a range of community-contributed
commands, and how predictions and counterfactual simulations
for both the conditional and the marginalized (unconditional)
distributions can be easily obtained from the fitted models. I also
discuss the pros and cons of such conditional likelihood models in
comparisons with quantile regression or distribution regression
approaches.
Additional information: Phillippe Van Kerm
Universität von Luxemburg
|
4:00–4:30 | Econodistplot: distribution box plots, economist-style
Abstract:
I present a new Stata package called conodistplot, which produces
simple box plots showing median and interquantile ranges defaulting to
the 10% and 90% percentiles. These plots have been introduced by The
Economist
(www.economist.com/united-states/2019/06/29/willtransparent-pricing-make-americas-health-care-cheaper)
and slightly adapted by Bob Rudis for the R platform (cinc.rud.is/w
eb/packages/ggeconodist). The
package presented here reproduces the look of Rudis' graphs, with the
motivation of creating an easily legible and visually appealing data
summary.
Additional information: Alex Gamma
Universität Zürich
|
5:00–6:00 | Introduction to Bayesian Statistics Using Stata
Abstract:
Bayesian analysis has become a popular tool for many statistical
applications. Yet many data analysts have little training in the theory
of Bayesian analysis and software used to fit Bayesian models. This presentation
will provide an intuitive introduction to the concepts of Bayesian
analysis and will demonstrate how to fit Bayesian models using Stata.
No
prior knowledge of Bayesian analysis is necessary. Some specific topics
I will cover are the relationship between likelihood functions, prior
distributions, and posterior distributions; Markov chain Monte Carlo
(MCMC) using the Metropolis—Hastings algorithm; and how to use Stata's
bayes prefix to fit Bayesian models.
Additional information: Gustavo Sánchez
StataCorp
|
6:00–6:30 |
Open panel discussion with Stata developers
StataCorp
|
Scientific committee
Ben Jann Universität Bern |
Blaise Melly Universität Bern |
Logistics organizer
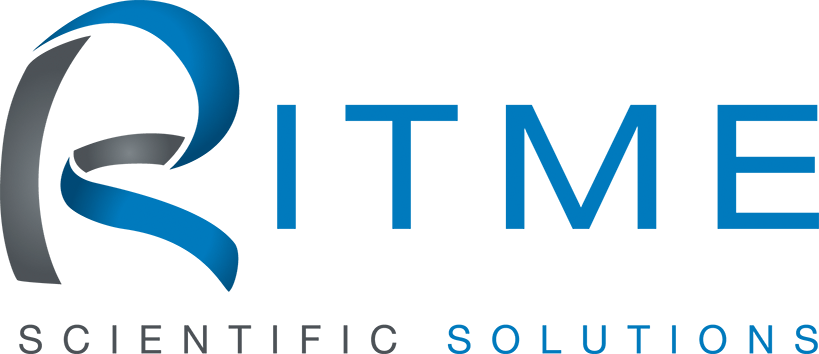
The logistics organizer for the 2020 Swiss Stata Conference is Universität Bern and Ritme, scientific solutions, the official distributor of Stata in Belgium and Switzerland.
View the proceedings of previous Stata Conferences and Users Group meetings.