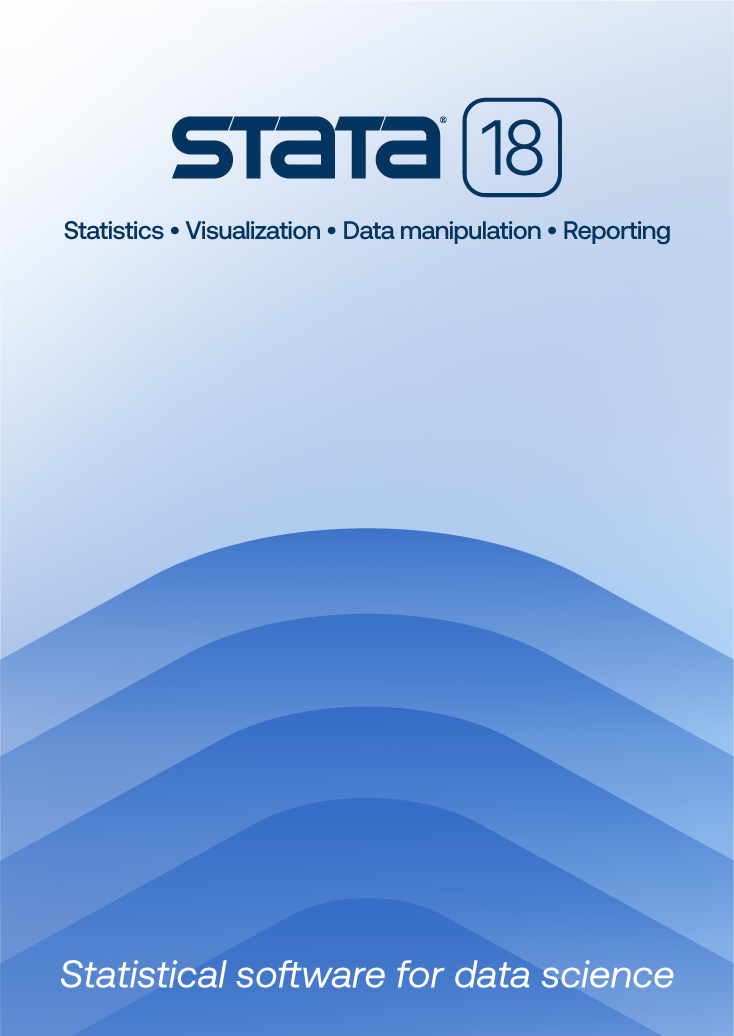
Last updated: 21 September 2009
Karolinska Institutet
Department of Medical Epidemiology and Biostatistics
Wargentine Lecture Hall
Nobels väg 12A
SE-171 77 Stockholm
Sweden
Multiple imputation is a popular simulation-based method for handling missing data. It replaces missing values with multiple sets of simulated values from an imputation model, applies primary analyses of interest to each imputed dataset, and obtains parameter estimates adjusted for missing-data uncertainty.
Stata 11's mi command for multiple-imputation analysis performs imputation, data management, and estimation. mi impute provides five univariate and two multivariate imputation methods. mi estimate combines the estimation and pooling steps of the multiple-imputation procedure into one easy step. mi also provides an extensive ability to manage multiple-imputed data.
The presentation will cover all aspects of using Stata 11's mi command to perform multiple-imputation analysis from imputation to data management to estimation.
Background: Underreporting is a common problem in dietary surveys and is particularly problematic for the obese. Underreporting in association with obesity may be further exacerbated by the assumption of standard portion sizes and by the assumption that missing data indicates that food is not eaten. Multiple imputation of missing data has been shown to be superior to single imputation assuming zero consumption or other plausible values. Use of portion size pictures may also reduce bias by capturing more individual variation associated with obesity. This study describes how multiple imputation as well as the use of a self-reported generalized portion size measure can improve the agreement between reported energy intake and expenditure and reduce obesity-related bias.
Method: InterGene is a population-based survey in which 1380 men and 1511 women completed a validated food frequency questionnaire (FFQ) with a supplementary 9-level scale describing portion size, based on photographs of a typical meal. Energy intake (EI) calculations were based on 92 food frequencies together with age- and sex-specific standard servings. Participants also underwent body composition measurement and reported on their physical activity levels, making it possible to estimate usual energy expenditure (EE).
Results: Obese participants had higher energy expenditure and reported higher portion sizes, but not higher energy intake than the non-obese, assuming zero intake for missing frequencies as well as standard portions. The amount of missing data was similar among normal, overweight, and obese participants.
The gaps between EE and EI were significantly smaller based on the imputed data and even more reduced when adjusting for portion size propensity. The improved agreement is not simply a result of an overall increase of EI, but also on individual level. In all three BMI categories the correlation coefficient between EE and EI tended to increase after imputation and adjustment for proportion size propensity. However, there is still no significant upward trend in energy intake by the BMI category even if the improvement is more obvious in the overweight and obese groups.
Conclusions: Missing data imputation and portion size propensity can significantly improve energy estimates from self reported FFQ. However, both methods cannot fully correct for the large underreporting in overweight and obese people. In addition, future work will examine whether we can use these adjustment procedures to obtain more valid values at the nutrient level.
Yvonne Åberg, Metrika Consulting and Stockholm UniversityNicola Orsini, Karolinska Institutet
Paul Dickman, Karolinska Institutet
Metrika Consulting, the official distributor of Stata in the Nordic and Baltic regions, and the Karolinska Institutet.