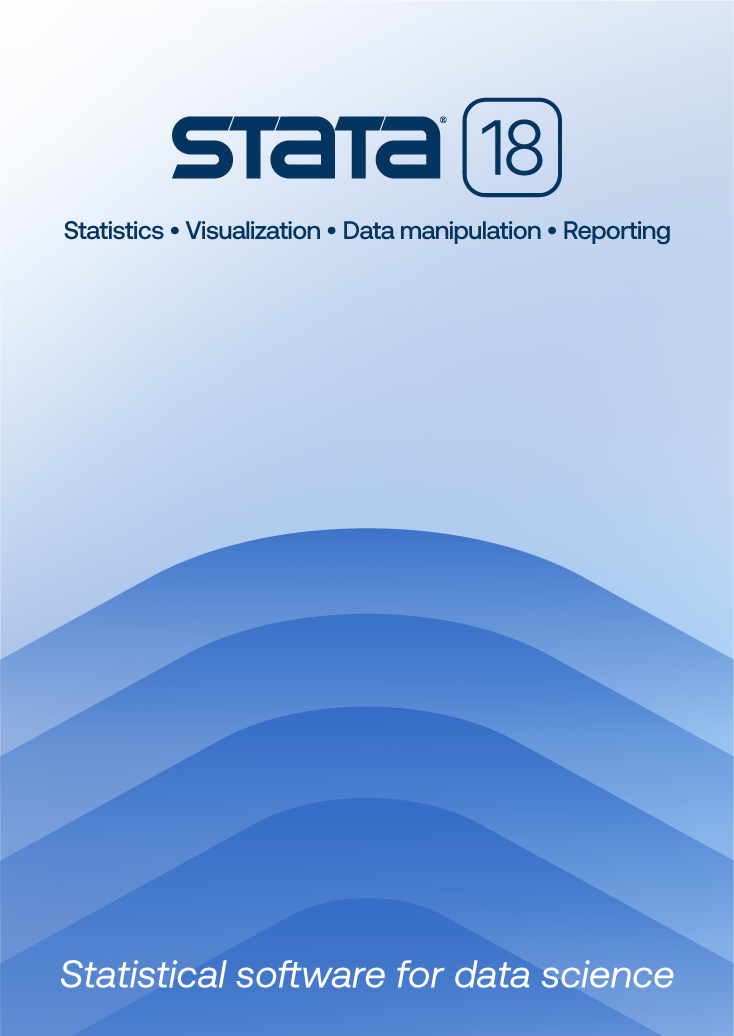
Session chair: Ronnie Babigumira |
|
9:05–9:30 | Application of stpm2 to estimate relative survival for cancer patients in the Nordic countries
Abstract:
In this presentation, I will describe the benefits and
challenges with comparing population-based survival across the
Nordic countries using the relative survival framework.
I used the NORDCAN database, which includes patients diagnosed with
cancer from 1990 to 2016 from Denmark, Finland, Iceland, Norway, and
Sweden. I adopted a model-based approach using flexible
parametric survival models and compared them with nonparametric
estimates. stpm2 and standsurv were
used for obtaining parametric estimates, and strs for
nonparametric estimates. I will discuss issues such as
age standardization, model stability, winsorizing, and
conditional survival.
Additional information:
Frida Lundberg
Karolinska Institutet
|
9:30–10:15 | Improving fitting and predictions for flexible parametric survival models
Abstract:
Flexible parametric survival models have been available in Stata
since 2000 with Patrick Royston’s stpm command.
I developed stpm2 in 2008, which added various
extensions. However, the command is old and does not take
advantage of some of the features Stata has added over the
years. I will introduce stpm3, which has been completely
rewritten and adds a number of useful features, including
Additional information:
Paul Lambert
University of Leicester and Karolinska Institutet
|
10:15–10:40 | Survival by first-line treatment type and timing of progression among follicular lymphoma patients
Abstract:
In follicular lymphoma (FL), progression of disease within 24
months has emerged as a popular prognostic marker for overall
survival (OS).
While it has considerable clinical relevance, there are also
inherent limitations in relation to the fixed time point of 24
months and potential variation by treatment type and choice of
comparison group. In this presentation, we will highlight some
of the methodical limitations and present the first results from
a large population-based cohort of FL patients. National
register-based information has been combined with detailed
medical record data to create a unique cohort with detailed
treatment and follow-up information. We allow progression to be
time varying and estimate relative rates, as well as OS by
first-line treatment and timing of progression using an
illness-death modeling approach. Stata packages merlin
and multistate were applied, and example code will be
presented. Our findings show that progression is associated
with worse survival beyond the 24-month time point, illustrating
the need for individualized management by timing of progression
for optimal care of patients with FL.
Contributors:
T. Wästerlid
B.E. Wahling
P.O. Andersson
S. Ekberg
S. Lockmer
G. Enblad
M. J. Crowther
E. Kimby
K. E. Smedby
Additional information:
Caroline Weibull
Karolinska Institutet
|
11:00–11:15 | The devil is in the details ... and the data: Tutorial on preparing data for multistate modeling
Abstract:
Data preparation for multistate models is a foundation prior to
estimation using parametric models or nonparametric approaches.
In Stata, for example, the multistate packages command
msset is a data-preparation tool to transform data in
wide format into long format. However, msset is not
optimal for multistate model settings with reversible
transitions, that is, transitions that allow recovery from one
state to another. In this case, correctly defining each
transition’s risk time and event (status) without using a
program may be preferred. This presentation aims to guide users
to prepare data for a reversible multistate model from wide
format to long format without using a data-preparation command
and will provide tutorials with example data.
Additional information:
Enoch Yi-Tung Chen
Karolinska Institutet
|
11:15–11:40 | Establishing upper reference limits for left-censored and contaminated data
Abstract:
When one establishes reference interval limits, measurements can
sometimes be characterized by either A) being left-censored or
B) being contaminated in the upper end.
Although solutions to both characteristics have been described
separately, no one to our knowledge has been handling the case
when both characteristics are present. Left-censored data (A)
are often wrongfully handled simply by using limit of detection
(LOD), which leads to high mean estimates and too-low standard
deviation estimates and hence incorrect cutoffs. Ignoring the
characteristic (B), researchers often use transformations to
handle the observed non-Gaussianity, which leads to too-high
cutoffs and an increased proportion of false negatives (type 2
error). We propose a method based on normal quantile plots and
OLS regressions to find the upper limit of a reference interval
for measurements in a case characterized by A) and B). We also
demonstrate how our method can be used to identify whether B)
is present in a dataset. We demonstrate our proposed method
using real data in two cases. Based on joint work by Niels
Henrik Bruun, Stine Linding Andersen, Nanna Maria Uldall Torp,
and Peter Astrup Christensen.
Additional information:
Niels Henrik Bruun
Aalborg University Hospital
|
Session chair: Tor Åge Myklebust |
|
1:00–1:25 | Flexible and fast estimation of quantile treatment effects: The rqr and rqrplot commands
Abstract:
Using quantile regression models to estimate quantile treatment effects
is becoming increasingly popular.
This presentation introduces the rqr command, which can be
used to estimate residualized quantile regression (RQR)
coefficients, and the rqrplot postestimation command, which
can be used to effortlessly plot the coefficients. The main
advantages of rqr compared with other Stata commands that
estimate (unconditional) quantile treatment effects are that it
can include high-dimensional fixed effects and that it is
considerably faster than the other commands.
Contributors:
Andreas Haupt
Karlsruhe Institute of Technology
Øyvind Wiborg
University of Oslo
Additional information:
Nicolai T. Borgen
University of Oslo
|
1:25–1:50 | Visualizations of marginal and conditional quantiles based on weighted mixed-effects models
Abstract:
Dose–response meta-analysis is widely used in a variety of
fields to answer research questions based on multiple studies.
A challenge in such applications is presenting the magnitude of
uncertainty emerging from the data in light of the assumed
statistical model. The aim of this talk is to illustrate a
visualization tool that follows the command drmeta to
graph marginal and conditional quantiles of the predicted
dose–response relationships based on weighted mixed-effects
models estimated on tables of aggregated data. The developed
postestimation command works with different study designs, dose
transformations, and outcome measures; it allows the
investigator to derive any quantile (0.01 to 0.99) of the
pointwise dose–response relationship; it allows the
investigator to define a fine grid of dose values and to choose
a referent; it shades quantiles to help distinguishing common versus
extreme quantiles; it allows the user to overlay the
study-specific BLUPs; it returns both static images for research
articles and interactive html visualizations for web
dissemination; it is based on Plotly Graphing Library taking
advantage of the Stata/Python integration. Real and simulated
data will be used to illustrate the use of the postestimation
command.
Additional information:
Nicola Orsini
Karolinska Institutet
|
1:50–2:50 | Econometrics strikes back: GMM and two-way fixed effects
Abstract:
Two-way fixed effects is not a broken methodology. As Wooldridge
(2021) shows, the estimator can be used to obtain heterogeneous
treatment effects.
I illustrate how to obtain these treatment
effects using GMM. Additionally, I show how some other proposed
estimators for heterogeneous treatment effects can be fit using
GMM.
Additional information:
Enrique Pinzon
StataCorp
|
3:15–3:40 | Recursive bivariate copula estimation and decomposition of marginal effects
Abstract:
This presentation describes a new Stata command,
rbicopula, for fitting copula-based maximum-likelihood
estimation of recursive bivariate models that enable a flexible
residual distribution and differ from bivariate copula or probit
models in allowing the first dependent variable to appear on the
right-hand side of the second dependent variable.
The new command provides various copulas, allowing the user to
choose a copula that best captures the dependence features of
the data caused by the presence of common unobserved
heterogeneity. Although the estimation of model parameters does
not differ from the bivariate case, the existing
community-contributed command bicop does not consider
the structural model's recursive nature for predictions and
doesn't enable margins as a postestimation command.
rbicopula estimates the model parameters, computes
treatment effects of the first dependent variable, and gives the
marginal effects of independent variables. In addition, marginal
effects can be decomposed into direct and indirect effects if
covariates appear in both equations. Moreover, the
postestimation commands incorporate two goodness-of-fit tests.
Dependent variables of the recursive bivariate model may be
binary, ordinal, or a mixture of both. I present and explain the
rbicopula command and the available postestimation
commands using simulated data and data from the Stata website.
Additional information:
Mustafa Coban
Institute for Employment Research (IAB)
|
3:40–4:05 | Illuminating the factor and dependence structure in large panel models
Abstract:
In panel models, a precise understanding about the number of
common factors and dependence across the cross-sectional
dimension is key for any applied work.
This presentation will give an overview about how to estimate
the number of common factors and how to test for cross-sectional
dependence. It does so by presenting two community-contributed
commands: xtnumfac and xtcd2. xtnumfac
implements 10 different methods to estimate the number of
factors, among them the popular methods by Bai and Ng (2002) and
Ahn and Horenstein (2013). The degree of cross-section dependence
is investigated using xtcd2. xtcd2 allows
implements three different tests for cross-section dependence,
based on Pesaran (2015), Juodis and Reese (2021), and Pesaran and Xie
(2021). The presentation includes a review of the theory, a
discussion of the commands, and empirical examples.
Additional information:
Jan Ditzen
Free University of Bozen-Bolzano
|
4:05–4:30 | sttex: A new dynamic document command for Stata and LaTeX
Abstract:
In this presentation, I will introduce a new command for
processing a dynamic LaTeX document in Stata, for example, a
document containing both LaTeX paragraphs and Stata code.
A key feature of the new command is that it tracks changes in
the Stata code and executes the code only when needed, allowing
for an efficient workflow. The command is useful for creating
automated statistical reports, writing articles with data
analysis, preparing slides for a methods course or a conference
talk, or even writing a complete textbook with examples of
applications.
Additional information:
Ben Jann
University of Bern
|
4:30–4:55 | Estimating adjusted absolute risks in a cross-sectional register-based study with logit and margins
Abstract:
In epidemiology, we are often interested in quantifying effects
of exposures not only on the relative scale (for example, risk
ratios and odds ratios) but also on the absolute scale (for
example, risks and risk differences).
In a cross-sectional study using data from the Swedish Medical
Birth Register, we used logit to estimate odds ratios of
adverse obstetric outcomes for different exposures. Adjusted
absolute risks and absolute risks differences were estimated
using the postestimation command margins. We will also
discuss possibilities to extend these methods to matched
cross-sectional data.
Additional information:
Anna Johansson
Karolinska Institutet and Cancer Registry of Norway
|
11:40–11:55 | Securing Stata in a secure environment. Data access and logging.
Abstract:
At Cancer Registry of Norway, we have developed a secure
environment for using Stata. A short description of this work is
given describing data access, logging of data extraction
(Java plugins+JDBC), and logging of Stata commands.
Additional information:
Bjarte Aagnes
Cancer Registry of Norway
|
4:55–5:30 | Open panel discussion with Stata developers
Contribute to the Stata community by sharing your feedback with StataCorp's developers. From feature improvements to bug fixes and new ways to analyze data, we want to hear how Stata can be made better for our users.
|
Michael J. Crowther, PhD
13 October 2022
This workshop is designed for statisticians and researchers with a good working knowledge of the principles and practice of survival analysis, including modeling of survival data.
It aims to provide an overview of multilevel mixed-effects survival analysis, including recurrent event analysis and joint recurrent-terminal event models, as well as introduce and illustrate tools in Stata for conducting multilevel survival analysis, including both modeling and prediction, with a focus on calculating clinically useful predictions.
Visit the official course page for more information.
Tor Åge Myklebust – Chair Cancer Registry of Norway |
Morten W. Fagerland Oslo University Hospital |
Arne Risa Hole Universitat Jaume I |
Peter Hedström Linköping University |
Anna L.V. Johansson Karolinska Institutet & Cancer Registry of Norway |
Bjarte Aagnes Cancer Registry of Norway |
The 2022 Northern European Stata Conference is jointly organized by Metrika Consulting AB, the official distributor of Stata for Russia and the Nordic and Baltic countries, and the Cancer Registry of Norway—Institute of Population-based Cancer Research.
View the proceedings of previous Stata Conferences and Users Group meetings.