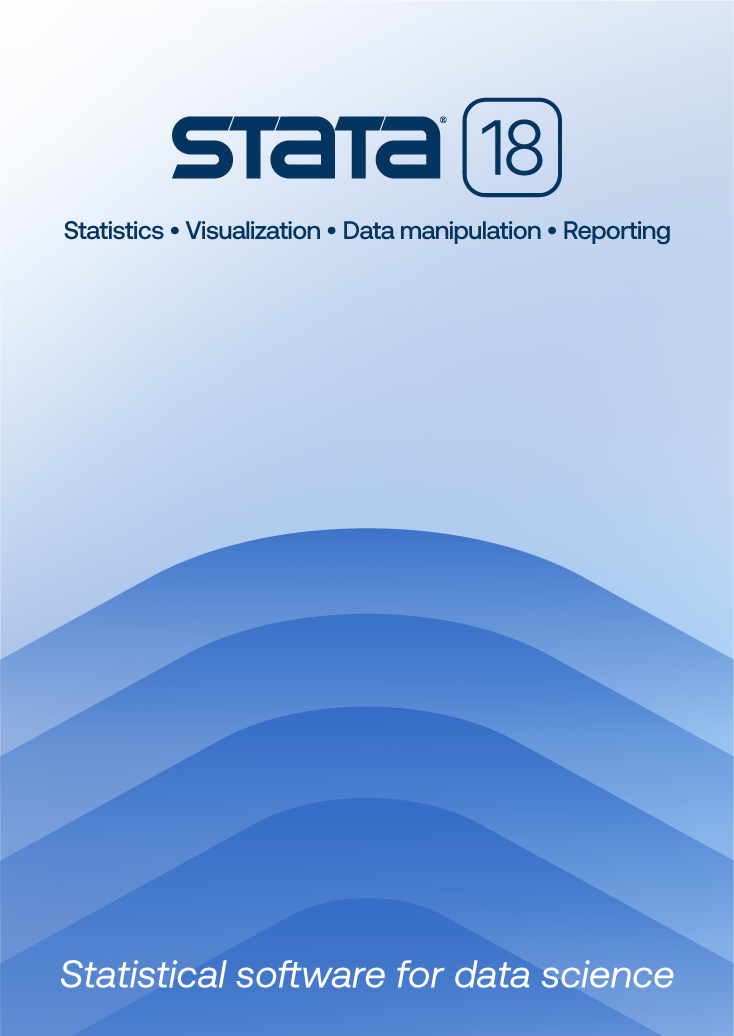
2:00–3:00 | Difference estimation in heterogeneous differences in Stata
Abstract:
The effects of a treatment may be different for groups treated
at different time periods or may change over time after a group
has been treated.
Think, for example, of the effect of job training programs on
earnings or the effectiveness of COVID vaccines. To capture this
heterogeneity, Stata 18 introduces two commands that estimate
specific treatment effects for each cohort and time period. For
repeated cross-sectional data, we have hdidregress. For
panel data, we have xthdidregress. Both commands allow you
to group treatment effects by cohort and treatment exposure and
display these effects graphically. Pretreatment parallel trend
testing is also available. This presentation will illustrate how
the two commands work and briefly discuss the theory behind
them.
Additional information:
Dr. Eduardo García Echeverri
StataCorp LLC
|
3:00–4:00 | Introduction to Bayesian model averaging in Stata
Abstract:
Model selection represents a key aspect in regression analysis.
Most empirical applications consider a fixed unknown underlying
data-generating model (DGM) that researchers try to find, based
on a particular theoretical framework that is combined with the
data associated to the variables involved in the selected model
specification. Bayesian model averaging provides an approach,
where instead of focusing the estimation on the search for that
unique unknown model, researchers can incorporate the
uncertainty about the DGM to obtain probabilities associated to
relevant predictors, measurements about complementary or
substitutable predictors across different model candidates, and
predictions that incorporate uncertainty about the model
and the parameters. In this presentation, we will use the new
suite of bma commands to illustrate those and other aspects
that can be derived using Bayesian model averaging.
Additional information:
Dr. Gustavo Sánchez
StataCorp LLC
|
2:00–3:00 | Differences in stacked differences and local projections
Abstract:
The differences-in-differences (DID) method is a popular tool
for estimating treatment effects with observational data.
Usually, this method is implemented with fixed-effects
estimators. However, recent studies have shown that fixed-effect
estimates may be biased in scenarios with heterogeneous
treatment effects. In this presentation, the estimation methods
of stacked DID and local projections are discussed. These
methods are simple and flexible and unify several of DID's
estimators for heterogeneous effects. It shows how to implement
these estimators and compares them with the DID tools of Stata
18.
Additional information:
Dr. Jorge Pérez
Banco de México
|
3:00–4:00 | GEE analysis: A methodology for the analysis of repeated data
Abstract:
Some studies have results where the outcome is a variable that
is measured repeatedly; that is, the variable is measured in the
same individual several times.
The GEE analysis or generalized estimating equation system is an
important option. This system accounts for the correlation
structure between the multiple observations in the individual.
It assumes an a priori 'operational correlation' and corrects
for the dependency between the observations in the subject.
Additional information:
Dr. William Martínez
Médico, Doctor en Epidemiología e Investigador
|
3:00–4:00 | Stata for causal inference: What to expect when you're expecting (the war is over)
Abstract:
One of the channels through which wars affect development is
that they have negative consequences on people's health from the
moment they are in the womb.
This study presents evidence of the consequences of the end of
the armed conflict, based on the analysis of a natural
experiment presented in Colombia and generated by the end of
the conflict between the national government and the guerrillas
of the Revolutionary Armed Forces of Colombia (FARC). Using the
administrative records of more than five million births between
2011 and 2018, and through a difference-in-differences model, I
find evidence that the end of the conflict increased the
probability of very low birthweights because, in the absence of
conflict, the birth of fetuses with less health endowment was
possible.
Additional information:
Camilo Arias
Universidad de los Andes
|
3:00–4:00 | Machine learning for time-series structures using Stata and Python
Abstract:
The high degree of volatility in the information requires
considering techniques and methods that ensure stability for the
different forecasts of variables of interest.
In this presentation, a comparison will be presented between
classical techniques versus machine learning for forecasting,
considering performance evaluation, associated with time-series
structures.
Additional information:
Franco Mansilla
Banco Crédito e Inversiones de Chile
|
The logistics organizer for the 2023 Colombian Stata Conference is Software Shop, the official distributor of Stata in Argentina, Bolivia, Chile, Colombia, Costa Rica, Ecuador, Mexico, Peru, and Venezuela.
View the proceedings of previous Stata Conferences and Users Group meetings.