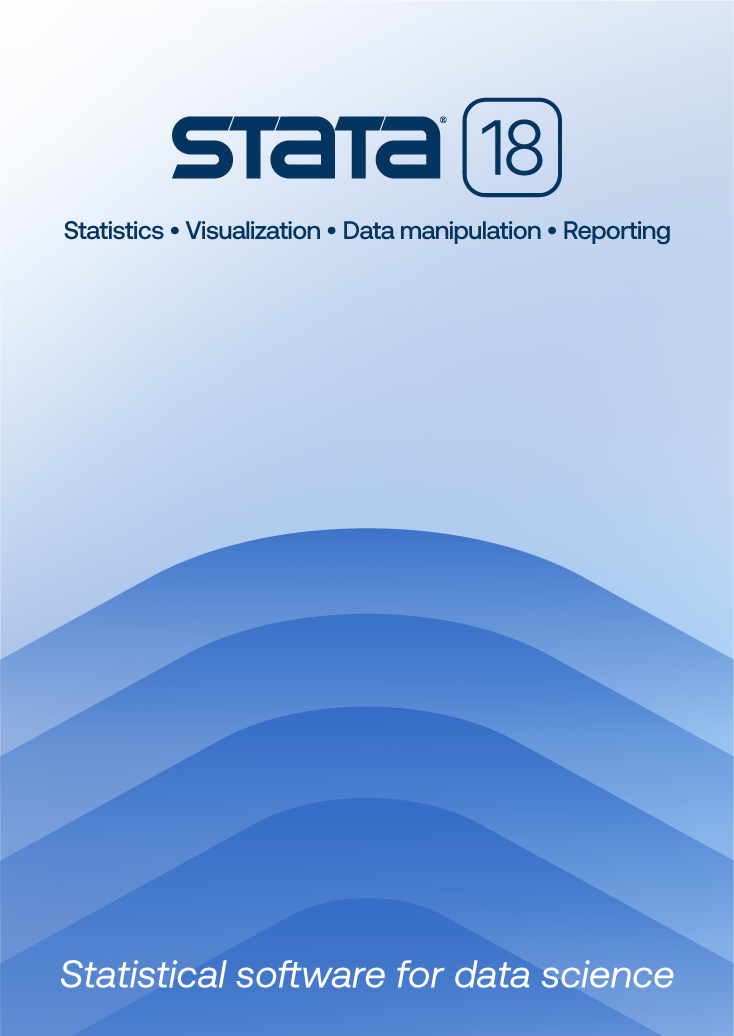
9:40–10:10 | A quasisynthetic control method for nonlinear models with high-dimensional covariates
Abstract:
To make the conventional synthetic control methods more flexible
to estimate the average treatment effect (ATE), this
presentation proposes a quasisynthetic control method for
nonlinear models under the index model framework with possible
high-dimensional covariates. The presentation suggests using
the minimum average variance estimation (MAVE) method to
estimate parameters and the lasso-type procedure to choose
high-dimensional covariates. We derive the asymptotic
distribution of the proposed ATE estimators for both finite and
diverging dimensions of covariates.
Additional information:
Fang Ying
Xiamen University
|
10:30–11:30 | Data visualization with Stata
Abstract:
This presentation will demonstrate how to produce informative,
robust, and complex graphs using reproducible official and
community-contributed routines in Stata. We will also discuss
commonly used programming tools and tips for creating more
engaging graphs.
Additional information:
Hua Peng
StataCorp LLC
|
11:40–12:10 | The health effects of clean energy development: Taking the West–East Gas Transmission Project as an example
Abstract:
How to solve the negative impact of environmental pollution
caused by energy consumption on public health is an important
challenge to achieving the goal of a healthy China, and the
development of clean energy provides a feasible governance path
for this. This presentation takes the commissioning and
operation of the West–East Gas Pipeline II Project as a
quasinatural experiment and uses the China Health and
Nutrition Survey (CHNS) data from 2006 to 2015 to empirically
examine how clean energy development affects public health. The
study found that the West–East Gas Pipeline Project has produced
health effects, and after passing multiple robustness tests, it
can still significantly improve the public health level in the
areas along the route. However, this effect is mainly reflected
in urban residents and the elderly, and the improvement of
household energy consumption structure, enterprise pollution
reduction, and improvement of urban environmental quality are
the main channels of action. Further analysis shows that the
“coal to gas” policy helps to enhance the health
effects of the project.
Additional information:
Wang Weiguo
Dongbei University of Finance and Economics
|
2:00–2:30 | New quality productivity measurement and evaluation methods
Abstract:
This presentation focuses on the current status of new quality
productivity research and the issues that should be paid
attention to in the measurement and evaluation process. No
substantial calculation of the new quality productivity
development index has been carried out. It is recommended that
the National Bureau of Statistics should regularly produce and
publish the "New Quality Productivity Development Index" as a
product, rather than having it published by the private sector.
Additional information:
Zhang Xiaotong
Nankai University
|
2:40–3:10 | Identification and estimation of average causal response function in a high-dimensional sample-selection model
Abstract:
Average causal response function (ACRF) is a useful tool to
assess treatment effect with dose functions, especially when the
treatment is endogenous. This presentation presents the
identification and estimation of an ACRF with sample selection
and high-dimensional controls. We derive the Neyman-orthogonal
moments with multiple nuisance parameters.
Additional information:
Zhou Yahong
Shanghai University of Finance and Economics
|
3:40–4:40 | Two analytical frameworks of regression discontinuity and Stata application
Abstract:
As one of the most important quasiexperimental causal inference
methods, regression discontinuity design has two major
analytical frameworks, which are quite different in terms of
premise assumptions, bandwidth selection, and inference methods.
Among them, the continuity-based framework assumes that the
conditional expectation of the potential results is continuous
and is widely used in empirical research. The local
randomization framework is a rising star. This framework assumes
that the driving variables can be regarded as randomly assigned
in a small window near the breakpoint. This presentation will
introduce the principles and techniques of these two frameworks,
including identification, estimation, and inference, and compare
the differences between the two through Monte Carlo simulation
and Stata cases, as well as their application prospects.
Additional information:
Chen Qiang
Shandong University
|
4:50–5:20 | Estimating interaction effects in probit models with endogenous regressors: eivprobit
Abstract:
The interaction effect in endogenous probit models with an
interaction term is consistently estimated in Zhou and Li
(2021). However, the estimation and test are time-consuming when
the sample size is large. In this presentation, a new Stata
command, eivprobit, is developed to implement
Zhou–Li's method in much less time. Besides, the marginal
effects of the two interacted regressors and the quadratic
effect of a regressor with a squared term can also be estimated
by the command. The eivprobit estimation is based on the
control function approach and the standard errors of the
estimated effects are obtained by nonparametric bootstrapping.
Moreover, the finite sample Monto Carlo simulation shows that
the estimator of the interaction effect behaves well and better
than the usual methods such as Ai and Norton (2003)'s estimator
ignoring endogeneity or the coefficient estimator of the
interaction term in IV-probit estimation.
Additional information:
Zhou Xianbo
Sun Yat-sen University
|
5:30–6:00 | Causal mediation analysis with multiple mediators and censored outcomes by GAN approach
Abstract:
Mediation models with censored outcomes play a crucial role in
social and medical sciences. However, the inherent censoring
characteristics of the data often lead existing models to rely
on assumptions of linearity, homogeneity, and normality for
estimation. Unfortunately, these assumptions may not align with
the complexities of real-world problems, limiting the
persuasiveness of causal analyses. In this study, I investigate
causal mediation analysis within a counterfactual framework by
framing it as a neural style transfer problem commonly
encountered in image processing. Acknowledging the impressive
capabilities of generative adversarial networks (GANs) in
handling neural style transfer, I propose a novel GAN-based
model named generative adversarial censored mediation network to
address mediation issues under my concern. My model employs
rectified linear unit (ReLU) activation function and designs a
particular multichannel network structure to implement the
censored outcome mechanism while accommodating multiple
mediators. To guide my model in accurately learning the
underlying data patterns, I also develop a novel min-max
optimization problem. Leveraging the strengths of GANs, my model
fundamentally relaxes the stringent assumptions present in
traditional models, resulting in more precise estimations of
mediation effects and promising inference outcomes, especially
in the context of intricate data patterns. Through unique
insights and techniques, this study illustrates how generative
learning methods can serve as an effective and robust approach
for diverse causal mediation problems. I substantiate our claims
with numerical results obtained from synthetic and realistic
datasets, showcasing the superior performance of my method.
Additional information:
Li Zhanfeng
Zhongnan University of Economics and Law
|
9:00–10:00 | Treatment-effects estimation using lasso
Abstract:
You can use treatment-effects estimators to draw causal
inferences from observational data. You can use lasso when you
want to control for many potential covariates. With standard
treatment-effects models, there is an intrinsic conflict between
two required assumptions. The conditional independence
assumption is likely to be satisfied with many variables in the
model, while the overlap assumption is likely to be satisfied
with fewer variables in the model.
Additional information:
Di Liu
StataCorp LLC
|
10:10–11:10 | Recent updates on econometrics of program evaluation
Abstract:
Over the past thirty years, project evaluation econometrics has
experienced great development. Thanks to the continuous progress
of econometric analysis software technology and the increasing
abundance of data, the empirical research paradigm of economics
and even the research paradigm of economics as a whole has
undergone a huge transformation, which has profoundly affected
the teaching and research of economics. In the past five years,
mainstream project evaluation econometric methods such as
DID, IV, and RD and a number of new econometric theoretical
advances have emerged. On the one hand, they have repaired and
improved the original theoretical methods and application
practices, and on the other hand, they have promoted the further
development of project evaluation econometric methods. I will
give a general introduction to these advances.
Additional information:
Zhang Chuanchuan
Zhejiang University
|
11:20–12:20 | Heterogeneity and endogenous peer-effect model and Stata application
Abstract:
The peer-effect (or neighbor-effect) model is an important model
for studying the mutual influence between individuals. Its
setting is similar to that of the spatial econometric model.
However, in the spatial econometric model, the adjacency matrix
is often regarded as exogenous. If the adjacency matrix is not a
geographical network, but a social or economic network then the
exogeneity assumption is unreasonable. This presentation proposes Stata
estimation commands for heterogeneous peer-effect models and
endogenous peer-effect models, snreghnet and
snregenet. snreghnet can examine the row
heterogeneity and column heterogeneity of the model.
snregenet calculates the two-stage instrumental-variables
estimation of the model and uses wild bootstrapping to calculate
the standard error.
Additional information:
Wang Qunyong
Nankai University
|
2:00–2:40 | xtteifeci: Estimating and inferring treatment effects using factorial methods
Abstract:
Li et al. (2024) extended the factor-based approach for
estimating and inferring treatment effects in interactive
fixed-effect panel models. This presentation introduces a new
Stata command, xtteifeci, which generates confidence
intervals and p-values for treatment effects period by
period and supports a variety of model settings, including
models that include covariates and nonstationary trends.
Finally, the specific operation of the command is introduced in
detail with a classic case.
Additional information:
Yan Guanpeng
Shandong University of Finance and Economics
|
2:50–3:30 | Spatial heterogeneity analysis of the development level of digital economy
Abstract:
With the rapid development of information technology and the
deepening of global economic integration, the digital economy
has become an important engine for driving global economic
growth. However, due to differences in resource endowments,
economic foundations, policy support and other factors in
different regions, the development level of the digital economy
shows obvious spatial heterogeneity in different regions.
Therefore, it is necessary and of practical significance to
conduct an in-depth analysis of the spatial heterogeneity of the
development level of the digital economy. This will not only
help us fully understand the distribution and development trends
of the digital economy in the country and even the world, but
also help to reveal the interactive relationship between the
digital economy and regional economic development, identify the
development gaps and potential risks of the digital economy
between regions, and promote the sustainable development of the
economy.
Additional information:
Tian Sisi
Data Analyst
|
4:00–4:40 | Stata text analysis: Possibilities and limitations
Abstract:
With the development of the times and the advancement of
technology, general statistical data has been widely used. At
the same time, unstructured data in the form of text is
gradually becoming the backbone of the empirical field of
business management. It is worth noting that researchers usually
give priority to using tools such as Python when conducting text
analysis. However, the migration of tools from Stata to Python
is often accompanied by a considerable learning cost. In this
case, we can't help but wonder, can we use Stata to do text
analysis content? This topic aims to introduce the mainstream
text analysis methods in the field of business management, and
explore the possibilities and limitations of using Stata for
these text analyses.
Additional information:
Zuo Xiangtai
Xiamen University
|
4:50–5:30 | Causal inference in networks with continuous disorders
Abstract:
In the case of perturbations when a treatment of one unit also
affects the outcomes of other units, the SUTVA assumption of
traditional causal inference is violated. When perturbations
work, policy evaluation relies mainly on the assumptions of
randomized experiments under cluster perturbations and binary
treatments. Instead, we consider non-experimental treatments
under continuous treatments and network perturbations.
Specifically, we define spillover effects by defining the
exposure to network treatments as the weighted average of the
treatments received by units connected by physical, social, or
economic interactions. Building on Forastiere et al. (2021), we
provide an estimator based on generalized propensity scores to
estimate the direct and spillover effects of continuous
treatments. Our estimator also allows for the consideration of
asymmetric network connections characterized by different
strengths. This presentation introduces a new Stata command that
combines the advantages of Mathematica, estimates individual
propensity scores and neighborhood propensity scores using
linear regression and machine learning methods, supports
multiple model settings, estimates the outcome model and
dose-response function (ADRF) using generalized linear models,
and calculates standard errors using bootstrapping. Finally, a
practical example is used to introduce the specific operation of
the command in detail.
Additional information:
Zhao Jun
Nankai University
|
4:50–5:30 | framerge: Horizontal merging of data through data frames
Abstract:
Stata's official commands merge and joinby can
perform horizontal merges of 1:1, m:1, 1:m, and m:m data.
However, these two commands require the use of datasets to be
saved to the hard disk, which not only increases the time cost
but also may generate a large number of intermediate files,
affecting efficiency. Stata's data frame function allows users
to operate multiple datasets in memory at the same time without
saving the data to the hard disk but its frlink and
frget commands support only 1:1 and m:1 merge types, not
1:m and m:m. The usual solution is to save the data using the
data frame to the hard disk, and then merge the data into the
main data frame with the help of the merge and joinby
commands.
Obviously, when the amount of data to be merged is large or there are many datasets involved, this method is very inefficient. This talk introduces a new command, framerge, to solve the above problems. The framerge command not only supports multiple data merge relationships (including 1:1, m:1, 1:m, and m:m) but can also directly operate data in memory without reading and writing hard disks, thereby improving the efficiency of data processing. The specific operation of the command is introduced in detail with a case. Propensity score supports multiple model settings, uses generalized linear model to fit the outcome model and dose–response function (ADRF), and uses bootstrapping methods to calculate standard error.
Additional information:
Chen Qiaowen
Xiamen University
|
The 2024 Chinese Stata Conference is organized by Beijing Uone Info & Tech Co., Ltd. (Uone-Tech), an official reseller of Stata in China.
View the proceedings of previous Stata Conferences and international meetings.