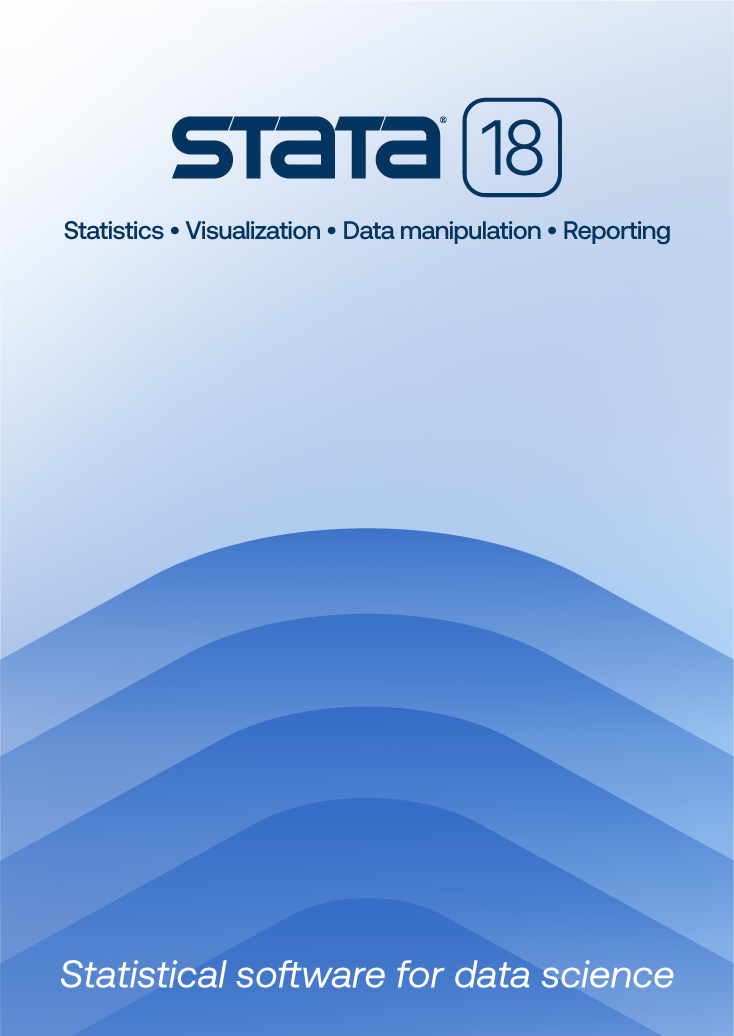
8:45–9:45 | Creating custom estimation tables
Abstract:
After fitting several regression models, researchers are often
interested in creating a regression table for the regression
results to examine the variables or statistics of interest.
This presentation introduces how to create an estimation table
using Stata’s new command etable and how to customize
the look of the table using collect.
Additional information:
Mia Lv
StataCorp
|
9:45–10:05 | cnevent: Event study is so simple
Abstract:
cnevent is an event research command newly developed by
the Stata and Python club.
Using this command, users only need to provide event lists.
cnevent will obtain the transaction data and index data
required for event research through the network and
automatically calculate the cumulative excess return according
to the market model set by users.
Additional information:
Chuntao Li
Henan University
|
10:05–10:30 | Reporting empirical results to .DOCX
Abstract:
Reporting empirical results automatically to generate structured
tables is an important but time-consuming task for empirical
researchers.
Because of the lack of commands that could effectively create and
edit Office Open XML documents (a .docx document), neither
official modules nor community-contributed commands could tabulate
results to this commonly used type of document until the launching
of putdocx in Stata 15. This presentation introduces four
new commands based on putdocx. They are sum2docx,
corr2docx, t2docx, and reg2docx. With these
commands, we can report summary statistics, correlation
coefficient matrices, split-sample t-tests, and regression
results automatically to a single .docx file. These commands
are simple and flexible to use, and they provide researchers with
new choices when reporting empirical results.
Additional information:
Yuan Xue
Huazhong University of Science and Technology
|
10:40–11:30 | Markov chain Monte Carlo simulation (MCMC) and Stata application
Abstract:
Markov chain Monte Carlo simulation (MCMC) is the core sampling
method of the Bayesian statistical and econometric model that is
used to approximate the distribution of unknown forms through
random samples.
I will introduce the concept of MCMC, Metroplis–Hasting and
Gibbs algorithms, the statistics of sampling results, and the
diagnosis of sampling quality. The application methods of
Metroplis–Hasting sampling and Gibbs sampling in Stata are
introduced through microeconometric model and macro time-series model.
Additional information:
Qunyong Wang
Nankai University
|
11:30–12:20 | Panel ARDL model and its application
Abstract:
The panel autoregressive distribution lag model (Panel ARDL model)
is mainly used to estimate the long-term relationship between
variables.
In time-series analysis, this model is mainly used to analyze the
long-term and short-term relationships between the nonstationary
series with cointegration relationships. In the panel data, we
can better control various fixed effects and consider spatial
correlation and heterogeneity to analyze the impact of a
policy or a variable with slow change (such as climate and
p) on economic growth, innovation, trade, and other
outcome variables.
Additional information:
Yujun Lian
Sun Yat-sen University
|
12:20–12:40 | Open panel discussion with Stata developers
Contribute to the Stata community by sharing your feedback with StataCorp's developers. From feature improvements to bug fixes and new ways to analyze data, we want to hear how Stata can be made better for our users.
|
The 2022 Chinese Stata Conference is jointly organized by Beijing Tianyan Rongzhi Software Co., Ltd. (TurnTech), an official reseller of Stata in China, and the Henan University School of Economics.
View the proceedings of previous Stata Conferences and Users Group meetings.