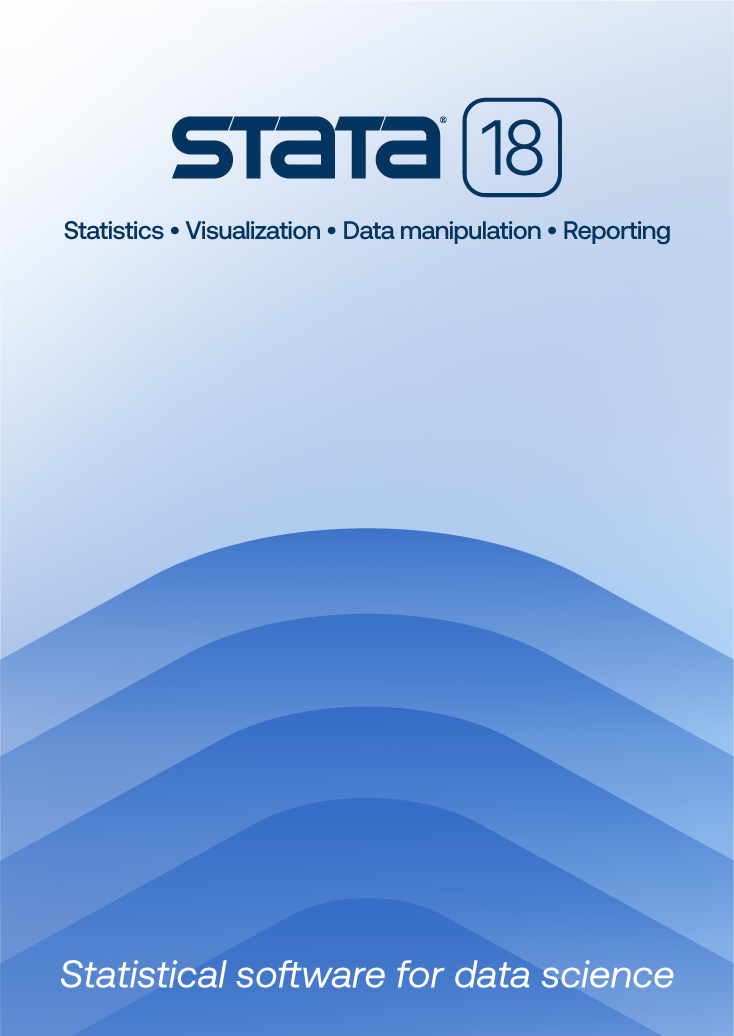
9:00–10:00 | Introduction to customizable tables in Stata 17
Abstract:
This presentation will introduce the new customizable tables in Stata 17.
I will discuss how to use the new table and collect commands
to collect, organize, manipulate, and display the results from Stata
commands to create customizable tables.
Additional information: Hua Peng
StataCorp
|
10:20–11:20 | Global VAR and Bayesian VAR in Stata
Abstract:
When applying the VAR model to panel data from multiple countries or
regions, the cross-sectional correlation and excessive parameters
should be considered. Global VAR and Bayesian VAR are commonly used
methods for fitting this large-scale VAR model.
This presentation
introduces the new Stata commands for these two VAR models,
gvar and bvar. The program has various built-in prior
distributions such as Litterman, Normal–Wishart, and
Giannone–Lenza–Primeceri, as well as Gibbs and MH sampling.
Additional information: Wang Qunyong
Nankai University
|
2:00–3:00 | Regression control method and Stata application
Abstract:
The regression control method (Hsiao, Ching, and Wan 2012) has become an
important method for evaluating policy effects using panel data. This
presentation will introduce the basic principles of the regression control
method, including the use of information criteria or lasso to select
cross-sectional units, and the addition of covariates to the regression
control method.
Then, through the community-contributed command, the specific
operation of the regression control method is introduced in detail with
classic cases, including the perfect drawing function and placebo test.
Additional information: Yan Guanpeng
Shandong University
|
3:20–4:20 | Quantile control method and Stata application
Abstract:
The causal inference of panel data is becoming increasingly popular,
including synthetic control methods, regression control methods, etc.,
but it is difficult to obtain standard errors, p-values, or confidence
intervals.
Starting from the regression control method, we propose the
quantile control method using quantile regression forests (that is, through random
forests for quantile regression) to construct the treatment effect of each
period confidence interval. Monte Carlo simulation shows that the confidence
interval constructed using the quantile control method performs well in a
limited sample. Finally, through the community-contributed command, the
specific operation of the quantile control method is introduced in detail
with a classic case.
Additional information: Chen Qiang
Shandong University
|
4:40–5:40 | Application of Stata in causal inference
Abstract:
Causal inference is a simple question often raised spontaneously by
human instinct: Why? In fact, as the core and purpose of scientific
research, causal inference is serious thinking, scientific verification,
or causal analysis on this issue. At present, causal inference models
and methods have been valued and favored in the fields of economics,
finance, sociology, management, demography, and public health, and have
become the core weapon of empirical research.
But causal inference is
not easy. As John Dewey said, "Scientific principles and laws do not
lie on the surface of nature. They are hidden, and must be wrested
from nature by an active and elaborate technique of inquiry."
There is no doubt that causal inference models
and methods are the core weapon for modern social science researchers
to publish high-level empirical papers using quantitative models. My
presentation focuses on how to
use Stata software for causal inference. In the seminars and
demonstrations, the causal inference model is organically combined with
the application and operation of statistical software, and I try to
share the basic ideas, principles, models, methods, and scope of
application of causal inference with you in a relatively short period
of time and use real data as the basis. I also demonstrate cases and share
the ability of causal inference, model construction, software
application, and result interpretation, laying a foundation for publishing
high-level empirical research papers.
Additional information: Wang Cuntong
Central University of Finance and Economics
|
9:00–10:00 | Fitting Cox proportional hazards model for interval-censored event-time data in Stata
Abstract:
In survival analysis, interval-censored event-time data occurs when
the event of interest is not always observed exactly but is known
to lie within some time interval. This type of data arises in many
areas, including medical, epidemiological, economic, financial, and
sociological studies. Ignoring interval-censoring will often lead to
biased estimates.
A semiparametric Cox proportional hazards regression model is used
routinely to analyze uncensored and right-censored event-time data. It
is also appealing for interval-censored data because it does not require
any parametric assumptions about the baseline hazard function. Also,
under the proportional-hazards assumption, the hazard ratios are
constant over time.
Semiparametric estimation of interval-censored event-time data is challenging because none of the event times are observed exactly. Thus, "semiparametric" modeling of these data often resorted to using spline methods or piecewise-exponential models for the baseline hazard function. Genuine semiparametric modeling of interval-censored event-time data was not available until recent methodological advances, which are implemented in the stintcox command. Join Xiao Yang, Principal Statistician and Software Developer, as she describes basic types of interval-censored data and demonstrates how to fit the semiparametric Cox proportional hazards model to these data using Stata's new stintcox command. She will also discuss how to interpret and plot results and how to graphically assess proportional-hazards assumptions.
Additional information: Xiao Yang
StataCorp
|
10:20–11:20 | Stata application of bilateral stochastic boundary model
Abstract:
The bilateral stochastic boundary model has been widely used in welfare
analysis, efficiency estimation, and corporate finance. In recent years,
the existing literature has made a series of expansions in model setting,
including different distribution assumptions and methods to reduce the
influence of distribution assumptions.
Our team has implemented an
estimation method based on the seminormal distribution and scaling property
and has developed a new Stata command. This presentation will introduce the
specific operation of the command by reproducing the classic literature and
comparing the estimation results set by different models.
Additional information: Liu Chang
Sun Yat-sen University
|
1:30–2:30 | Some thoughts of a Stata user
Abstract:
I started using Stata 8.0 in 2003. Now Stata has been upgraded to 17,
and I am deeply emotional. I have taught thousands of students and have
collaborated with more than 200 students from various universities to
write tweets and have some understanding of the puzzles that everyone
faces in study and research.
I would like to share some thoughts I have had over
the years, hoping to be helpful to everyone's study and research work.
This talk mainly includes the following issues:
Additional information: Lian Yujun
Sun Yat-sen University
|
2:50–3:50 | Stata application and cutting-edge research of synthetic control method
Abstract:
The synthetic control method for causal inference has become
one of the mainstream methods in empirical research in the past 10
years, but there are still many controversies about this method in
statistical inference. This presentation will be divided into two parts.
The first part will start with the classic practical cases of synthetic
control method combined with the relevant Stata commands, introduce
how to use this method for specific economic empirical scenarios, and
test the relevant hypotheses of the method. The second part will
systematically sort out the current controversies in the synthetic
control method and briefly introduce how the current econometrics
community solves the shortcomings of the method, such as the use of
machine learning algorithms to construct counterfactuals (Viviano and
Bradic 2019) and the use of ranking test methods realizing the
construction of confidence intervals (Chernozhukov et al. 2020).
Additional information: Lu Jiaxuan
University of Chicago
|
4:20–5:20 | Mixed regression with macrodata and microdata in Stata
Abstract:
In a type of model, the dependent variable is a macro variable and the
independent variable is a micro variable. How to use data-driven methods
to weight independent variables is always the core issue of this type of
model.
This presentation draws on the method of mixing regression (midasreg)
and introduces Stata's new program mixedreg, which is called mixed
regression. This presentation also introduces how to use the microdata of
listed companies to predict macroeconomic indicators through mixedreg.
Additional information: Wang Qunyong
Nankai University
|
4:00–4:30 | Open panel discussion with Stata developers
StataCorp
|
Bayesian Analysis and Stata Application
Wang Qunyong
16–18 August 2021 from 9:00 a.m. to 5:00 p.m.
This training covers Monte Carlo simulation, Markov chain Monte Carlo simulation, Bayesian analysis of regression models, Bayesian VAR models, Bayesian analysis of microeconometric models, Bayesian structural equation models, and writing your own likelihood functions and prior distribution.
Panel-Data Metrological Analysis and Stata Application
Wang Qunyong
21–23 August 2021 from 9:00 a.m. to 5:00 p.m.
This training covers classic models of panel data, dynamic panel, nonlinear panel model, using panel data for causal inference, panel-data restricted dependent variable models, Bayesian panel data analysis, and panel space measurement tools.
The 2021 Chinese Stata Conference is organized by Beijing Uone Info & Tech Co., Ltd. (Uone-Tech), an official reseller of Stata in China.
View the proceedings of previous Stata Conferences and Users Group meetings.