The Chinese Stata Conference took place on 19–20 August 2020. There was also a Stata Summer Training Camp offered 17–18 and 21–22 August.
Proceedings
9:15–10:15 | Using Stata to obtain and process COVID-19 data
Abstract:
Rapid data acquisition and analysis are the bases for public
health decisions. I will show various data acquisition toolkits
in Stata and their applications in acquiring and processing
COVID-19 data.
Additional information: Hua Peng
StataCorp
|
9:15–10:15 | Call Stata from Python
Abstract:
Stata 16 introduces tight integration with Python, allowing users
to embed and execute Python code from within Stata. In this presentation,
I will demonstrate a new functionality we have been working on—calling
Stata from within Python. We are working on
providing two ways to let
users interact with Stata from within Python: the IPython magic commands
and a suite of API functions. With those utilities, you will be able to
run Stata conveniently from Python environments, such as Jupyter
Notebook/console, Jupyter Lab/console, Spyder IDE, or Python launched
from a Windows Command Prompt, Unix terminal, etc.
Additional information: Zhao Xu
StataCorp
|
9:15–10:15 | Mixing regression methods and Stata applications
Abstract:
The sampling frequency of common variables is not uniform. For example,
GDP is often quarterly, while inflation rate is monthly, and financial
market data are often daily. Mixing data regression is a method that uses
low-frequency variables to regress high-frequency
variables and uses a
data-driven method to assign weights to high-frequency variables in
different periods to improve accurate prediction. It is more and more
widely used in macroeconomic forecasting. This presentation introduces the
mixing regression model and Stata's new command: midasreg. This
command allows regression of data of a variety of different frequencies,
includes multiple weighting functions such as STEP, PDL, and Beta, and is
compatible with Stata's standard commands such as estat and predict.
Additional information: Wang Qunyong
Nankai University
|
9:15–10:15 | Source of endogenity based on Stata simulation and its response
Abstract:
Although endogeneity is a common topic, it is still easily
overlooked in the modeling process. Through a unified framework and
simple Stata program simulation, I show how self-selection bias,
simultaneous causality, missing variables, measurement errors, etc.,
lead
to endogeneity. I combine easy-to-understand cases to show the
conditions under which control variables, difference estimation,
instrumental variables, breakpoint regression, matching, and other
methods are used. I also show how to overcome endogenity and obtain
consistent estimates.
Additional information: Chen Chuanbo
Renmin University of China
|
9:15–10:15 | Smooth conversion model and Stata application
Abstract:
The smooth transition model describes the transition relationship
between two or more states between variables, and the states are
realized by a smooth transition function. This model is widely used in
macroeconomics and financial markets, for example, oil prices and
economic fluctuations, banking and economic development, etc. This
presentation introduces the setting and related tests of the smooth
transition model, and Stata's new command: stregress. This command is
suitable for time series, cross-sectional data, and panel data, and
includes multiple conversion functions such as LSTR, ESTR, NSTR, and L2STR.
This presentation also includes multiple tests such as linearity of the model,
serial correlation of residuals, and parameter-constant characteristics.
Additional information: Wang Qunyong
Nankai University
|
9:15–10:15 | Span regression, regression, skewness, and kurtosis regression in Stata applications
Abstract:
Quantile regression is a powerful tool to study the effects of
covariates on key quantiles of conditional distribution. Yet we often
still lack a general picture about how covariates affect the overall
shape of conditional distribution. Using quantile-based measures of
spread, skewness, and kurtosis, we propose spread regression, skewness
regression, and kurtosis regression as empirical tools to quantify the
effects of covariates on the spread, skewness and kurtosis of
conditional distribution. While spread regression can be implemented by
the official Stata command iqreg, I provide the new commands
skewreg and kurtosisreg for skewness regression and kurtosis
regression, respectively, and illustrate them with an example of US
wage data with substantive findings.
Additional information: Chen Qiang
Shandong University
|
9:15–10:15 | Causal mediation
Abstract:
The estimation of the effect of intervention or exposure is a common
effect evaluation and an important statistical analysis aspect of
causal inference. In order to further understand the mechanism of action
or the path of action to improve intervention strategies, the
mediation
effect has become an important research aspect of social
development disciplines and epidemiological research, and has formed
different schools. Causal mediation starts from the counterfactual
framework, dealing with exposure-mediating confounding, exposure-outcome
confounding, and sensitivity testing of mediating-outcome confounding.
Additional information: Jin Chenggang
Beijing Normal University
|
9:15–10:15 | On improvement of placebo test of synthetic control method—statistical inference based on standardized treatment effect and nonrefusal domain
Abstract:
The statistical inference of the synthetic control method mainly relies
on the "placebo test" with the permutation test as the basic idea, but
this method has serious problems of excessive rejection and significance
pursuit. This presentation uses "quasi-standardized"
conversion to punish the
noise component in the placebo test process to avoid the inconsistency
of the policy effect distribution after the intervention to
ensure that we can implement the placebo test without deleting the
observations. The above improvements can overcome the excessive
rejection and saliency chasing problems faced by traditional statistics.
The study found that (1) the standardization of placebo test can
effectively reduce the heteroskedasticity of random shocks and the
excessive rejection caused by estimation bias; (2) the standardized
treatment of the placebo test can make the treatment group and the
distribution of policy effects of potential control groups meet
consistency and avoids the problem of chasing significant results;
(3) based on the standardized processing results, we can construct the
"nonrejection domain" of policy effects by using the bootstrap method
under relatively clean data conditions. This ensures that the
statistical inference framework of the synthetic control method is
consistent with traditional statistical inference.
Additional information: Lian Yujun
Sun Yat-sen University
|
9:15–10:15 | Measuring technical efficiency and total factor productivity change with undesirable outputs in Stata
Abstract:
In recent years, considering undesirable output in efficiency and
productivity analysis has become an important research direction.
Corresponding to this, in the field of efficiency and productivity
analysis, many new models that can consider both desirable and
undesirable output have gradually developed. This presentation shares
the nonparametric frontier estimation methods and applications of
efficiency and productivity, as well as the new Stata commands teddf
and gtfpch, cowritten with Du Kerui from Xiamen University. These
commands are suitable for estimating the efficiency and productivity
of decision-making units with undesirable output and cover a variety of
nonparametric frontier models based on directional distance functions.
Additional information: Daoping Wang
Shanghai University of Finance and Economics
|
Stata Summer Training Camp
Course name
Nonlinear models tell extraordinary stories
Presenter
Wang Qunyong
Date & Time
17–18 August 2020 from 9:00 a.m. to 5:00 p.m.
Description
This training mainly introduces the basic ideas and algorithms of nonlinear models, combined with specific cases, and introduces practical operation of Stata. Stata 16 is used throughout the demonstration.
Course name
Natural experiment and causal inference
Presenter
Wang Qunyong
Date & Time
21–22 August 2020 from 9:00 a.m. to 5:00 p.m.
Description
Causal inference is one of the important characteristics of micro-metric analysis. Counterfactuals are the most basic framework for causal inference. Policy endogeneity caused by self-selection and other issues has brought many obstacles to policy evaluation. This course introduces several tools to use observational data to infer causality: how to use natural experiments (or quasi-experiments) to infer causality, how to overcome policy endogenous problems, how breakpoint design weakens policy endogenous problems and model error settings problems, and how to use double check and synthetic control to construct counterfactual strategies in the panel data.
Logistics organizer
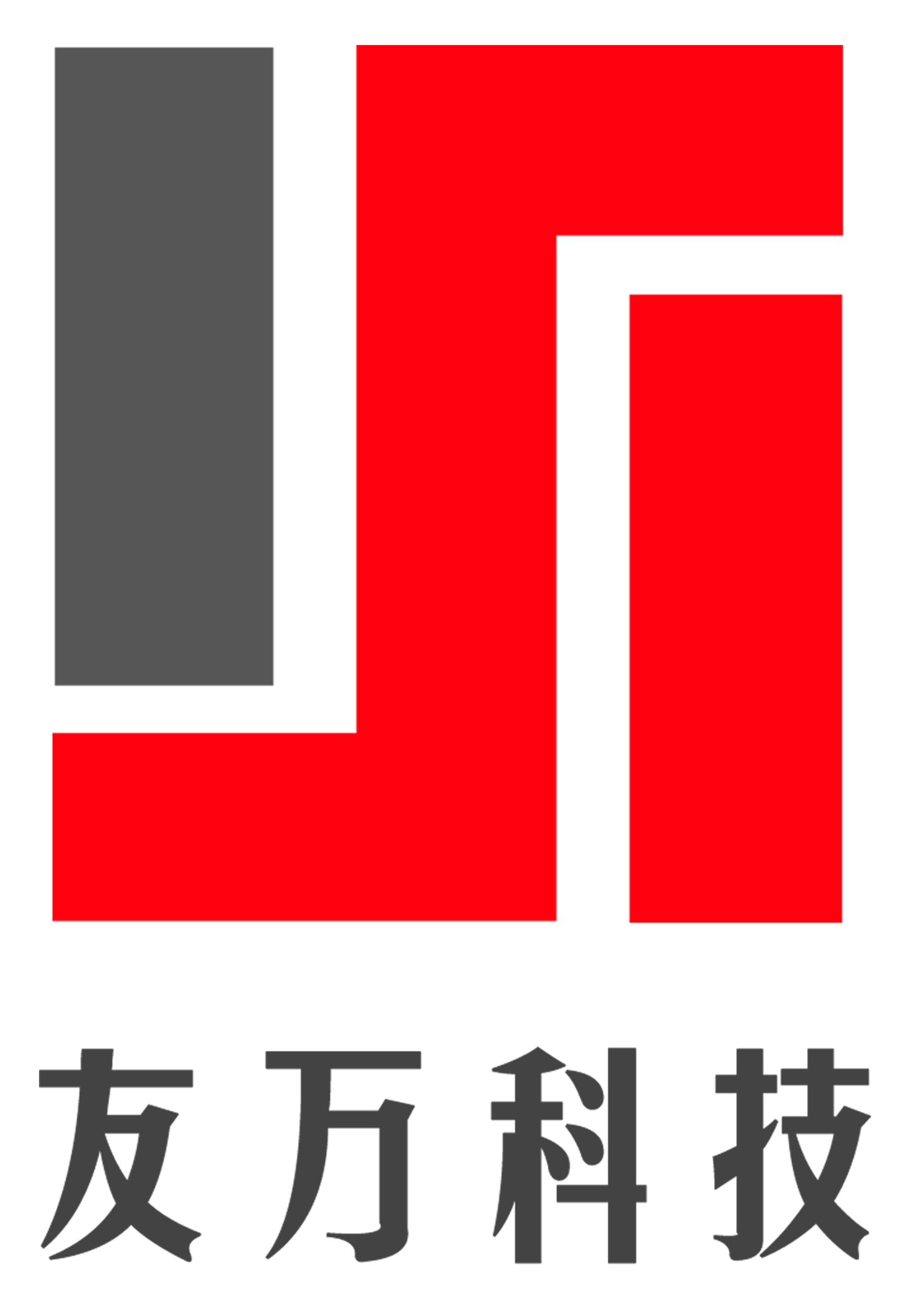
The 2020 Chinese Stata Conference is jointly organized by
Beijing Uone Info & Tech Co., Ltd. (Uone-Tech),
an official reseller
of Stata in China, and the Institute of Quantitative Economics, Nankai University.
View the proceedings of previous Stata Conferences and Users Group meetings.