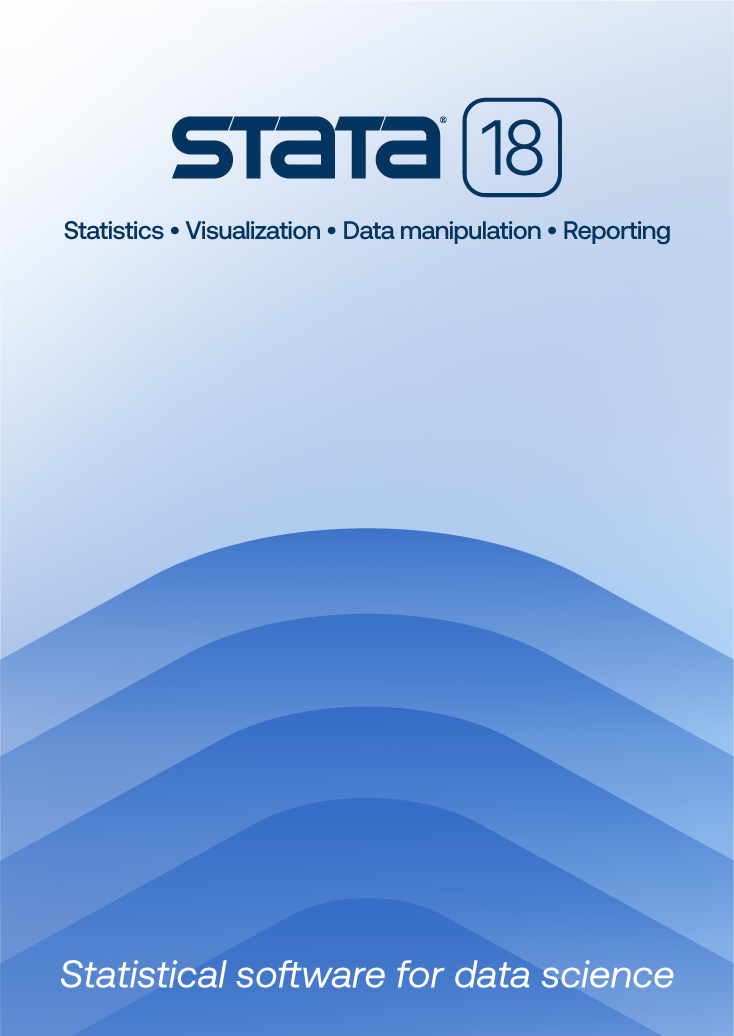
The seventh UK Stata Users Group meeting attracted about 50 participants to the Royal Statistical Society in London on 14 and 15 May. The meeting was organized by Bianca L. De Stavola (London School of Hygiene and Tropical Medicine) and Stephen Jenkins (University of Essex) with the administrative support of Timberlake Consultants, who also generously sponsored the speakers. William Gould and Robert Gutierrez from StataCorp attended, and enlivened, the meeting. Despite the UK label, the meeting attracted participants from other countries, e.g., US and Sweden, with the largest non-UK contingent from Italy.
The meeting was opened by Nick Cox (Durham University). His talk, "Plotting graded data: a Tukey-ish approach", was as lively and original as the UK SUG regulars are used to expect from him. Inspired by the recent death of John Tukey, Nick used plots of cumulative probabilities to compare graded data observed in different groups of subjects. These plots can be produced by ordplot using a wide selection of scales, ranging from flog to froot (!) via the better known logit. Not surprisingly the results offered greater insights into the data than their tabulation would have revealed. This was followed by a contribution by Andrew Pickles (University of Manchester). The features of Census Data available to researchers motivated the topic, "Fitting log-linear models with ignorable and non-ignorable missing data". The need to use both individual level data and data aggregated at some higher level within a log-linear model framework led Andrew and his collaborators to implement the composite link approach to missing data via first some complex data reorganization (carried out in makecct) and then an ml-based command (cctfit).
The next three talks introduced new commands for the st family. Patrick Royston (MRC Clinical Trials Unit) proposed a command for fitting proportional hazards and proportional odds models to survival data in "Flexible parametric alternatives to the Cox model...and more". The command, stpm, allows the estimation of a non-parametric baseline hazard as well as the relevant hazards or odds ratios. Since the baseline hazard is specified as a spline function, plotting it turns out to be easy and informative. Ian White introduced a new command, strbee, pronounced "strawberry" (despite Ian's drawing, to many resembling a tomato!). The command allows the user to estimate a treatment effect in randomised clinical trials when patients cross-over from their assigned treatment to the alternative one during follow-up. The method, developed by Ian and his collaborators, is based on work by Robins and Tsiatis (1991) and applies to accelerated life survival models. A related method for the analysis of observational studies was presented by Kate Tilling and Jonathan Sterne for their program, stgest, the name standing for G-estimation. It applies to survival data where both the exposure of interest and the confounder change over time with the latter's value s possibly on the causal path of the former. G-estimation requires at least three time-points where data are collected and uses those before the last, e.g., the first two when three are available to mimic the relative effect of being or not-being exposed for every exposed subject.
A general contribution to the analysis of epidemiological data was given by Michael Hills who showed a menu interface, efmenu, to the effects commands he and David Clayton presented at last year's SUG. Their command translates both input and output for generalized linear models, as used in epidemiology, into a "classical" framework where exposures and confounders are declared before the analysis is carried out. The menu makes this transition extremely smooth, although the programming involved apparently was not. Paul Seed concluded the packed morning session with a clear description of his new command for xt-type data, xtgraph. This allows producing summary graphs of the observed data using, for example, geometric means or medians, together with their values as predicted by any of the regression commands.
The afternoon session started in the same way as the morning one, that is with a presentation by Nick Cox. This time the topic was "Triangular plots" which can be produced with triplot. Such plots can be used to represent the distribution of three inter-related variables, for example, the percentages of workforce employed in agriculture, industry and services, over another dimension, e.g., time or region. It was then Sophia Rabe-Hesketh's turn to describe some of the new extensions to gllamm6, the generalized linear latent and mixture program she published with Andrew Pickles and C. Taylor in STB-53 (sg129). The new version of the program is called simply gllamm. To illustrate the extension that involves modelling multilevel nominal data and rankings, Sophia used British election data from 1987 and 1992 while the Diet data from the Stata manual was used to describe models with latent variables (true dietary intake) in the pathway between explanatory (occupation) and outcome (coronary heart disease) variables.
Another example of a menu-driven command was given by Abdel Babiker who developed it with Patrick Royston. Its use is for sample size calculations in randomised clinical trials where more than two groups may be compared in terms of survival. The menu is invoked by the ssmenu command. This allows the user to select a series of complex options (in the Stata sense) for the command calcssi. Losses to follow-up, staggered patient entry and non-proportional hazards are some of its more notable features. The afternoon was concluded by Bill Gould (StataCorp President) who entertained the audience with glimpses of the future (Stata-wise only, unfortunately) while the audience responded with a short list of grumbles. The serious part of the day over, most participants followed tradition and visited first the local pub and then the "Last Days of the Raj" in Covent Garden. Here the conversation ranged from "what is an Essex girl" to the future of British politics but ended when Bill Gould started to sing (this is nearly true).
The second day started with an interesting talk by Mohamed Ali who presented mtable (twinned to ltable), a program for computing cumulative incidence rates (and their SE) in the presence of competing risks. Mohamed stressed how the method implemented in his program, unlike the use of the complement of Kaplan-Meier curves, gives the correct estimates. A talk with an economic flavour then followed, despite the topic being still centred on survival data. Stephen Jenkins spoke about his program — spsurv — that estimates a discrete-time split population ("cure") survival model. In the standard survival model each subject is assumed to experience the relevant event sometime; in the split population model, an estimable fraction is allowed to experience the event. (In a biostatistics context this is the proportion of subjects under treatment who are `cured'.) Another economist, Kit Baum, then addressed the problems arising from managing large panel data sets consisting of pair-wise information on economic trade between 18 countries, spanning over many time points. The task appeared to be horrendous but Stata made Kit's life easy or, at least, that is what he claimed! Hundreds of non-linear regression models were then fitted for each country's trade pattern with every other country, and the results post-processed and summarised graphically.
Roger Newson took the audience back to medical applications with a step-by-step presentation of how splines can be parameterised and then fitted in a format that makes them more understandable by non-mathematicians. This is achieved via his program frencurv. With Barbara Sianesi we enthusiastically went back to an economic application. This concerned "propensity score matching" to be used for dealing with non-random allocation of individuals to a "treatment" (e.g., a training programme) and the estimation of its effect on an "outcome" (e.g., earnings). The method mirrors applications in biostatistics but the command, match, is tailored to econometricians.
The morning concluded with one more presentation on survival analysis and one on ordinal outcomes. The first talk had an economic motivation and the second a medical one, but both can be widely applied. The first was by Ken Simons who introduced sthaz for fitting smoothed hazards to survival data, via kernel density estimation. Confidence intervals can be computed while extensions to allow variable bandwidth smoothing are in the pipeline. The last presentation of the morning was by Mark Lunt who very lucidly reviewed the most used methods for the analysis of ordinal data. To this list Mark added the stereotype model, which is nested within the multinomial model and for which a program soreg (stereotype ordinal regression) is available.
After lunch all participants reconvened to listen to Bobby Gutierrez (StataCorp) who reviewed current features and future developments of frailty survival models in Stata. At a very fast pace, which reflected the speaker's enthusiasm for the topic, Bobby explained the conceptual difference between frailty and shared frailty models and discussed the effects of ignoring either of them when fitting parametric survival models. Extensions to Cox regression (shared) frailty models are still being developed.