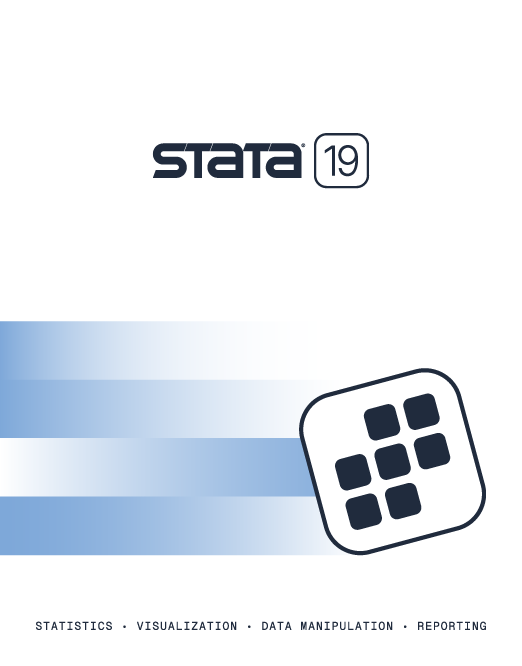
2025 Stata Conference • Nashville, TN • 31 July–01 August
Mata is a programming language that looks a lot like Java and C but adds direct support for matrix programming. Mata is a compiled language, which makes it fast. You can use Mata interactively when you want to quickly perform matrix calculations, or you can use Mata when you need to write complex programs. Mata has the structures, pointers, and classes that you expect in your programming language. In fact, Mata is Stata's development language. Most new features of Stata are written in Mata. This includes multilevel modeling, latent class analysis, Bayesian estimation, and even the core algorithms of the graphical SEM Builder. But Mata is not just for Stata developers; you too can take advantage of this powerful programming language.
You can use Mata interactively.
A = 1,2\3,4 // Input a 2 × 2 matrix b = -1\3 // Input a 2 × 1 column vector A'b // Compute A'b A[2,1] // Access the (2,1) element of A A[,2] // Access the second column of A B = diag(b) // Create a 2 × 2 matrix with the elements of b on the diagonal C = A,B // Combine matrices A and B by column D = A\B // Or by row I = I(3) // Create a 3 × 3 identity matrix I trace(A) // Compute the trace of A det(A) // And the determinant of A G*H // Multiply G (n × k) by H (k × k) G:*g // Multiply each column of G (n × k) by g (n × 1) st_data(.,.) // Load Stata dataset into Mata st_data((1,5\7,9), "mpg") // Observations 1 to 5 and 7 to 9 of variable mpg
Or you can write a simple program for regression.
mata st_view(y=., ., "mpg") st_view(X=., ., ("weight", "foreign", "cons")) b = invsym(X'X)*X'y b e = y - X*b n = rows(X) k = cols(X) s2= (e'e)/(n-k) V = s2*invsym(X'X) V se = sqrt(diagonal(V)) (b, se)
Or build a regression system using structures or classes.
class linreg { public: void setup() real scalar N() real scalar k() real scalar K() real colvector b_X() real scalar b_c() real colvector b() real matrix V() real scalar s2() real scalar ee() real colvector yhat() protected: // --------------------------------------- inputs pointer(real colvector) scalar y pointer(real matrix) scalar X real scalar cons // --------------------------------------- derived real matrix XX // X'X; K x K real colvector Xy // X'y; K x K real matrix XXinv // (X'X)^(-1); K x K real colvector b // coefficients; K x 1 real matrix V // variance matrix (VCE); K x K real scalar s2 // variance of e real scalar yy // y'y real scalar ee // e'e, error sum of squares real scalar K_adj real scalar k_adj /* Deviation from mean notation: S = X - mean(X) X as deviation from mean t = y - mean(y) y as deviation from mean */ real matrix SS // S'S; k x k real colvector St // S't; K x 1 (sic) real matrix SSinv // (S'S)^(-1); K x K (sic) real rowvector mean_X // means of rows of X; 1 x k real scalar mean_y // mean of row of y; 1 x 1 void init() real matrix XX() real colvector Xy() real scalar yy() real matrix XXinv() real matrix SSinv() void calc_SSinv_cons() real matrix SS() real colvector St() real rowvector mean_X() real scalar mean_y() real scalar K_adj() real scalar k_adj() } void linreg::init() { XX = .z Xy = .z XXinv = .z b = .z V = .z s2 = .z yy = .z ee = .z SS = .z St = .z mean_X = .z mean_y = .z SSinv = .z K_adj = .z k_adj = .z } /* -------------------------------------------------- entry points --- */ void linreg::setup( real colvector user_y, real matrix user_X, real scalar user_cons ) { assert(rows(y)==rows(X)) y = &user_y X = &user_X cons = (user_cons!=0) init() // initialize derived vars } real scalar linreg::N() return(rows(*X)) real scalar linreg::k() return(cols(*X)) real scalar linreg::K() return(cols(*X) + cons) /* Throughout this code, distinguish between k and K: k = # of independent variables EXCLUDING intercept K = # of independent variables INCLUDING intercept In models without an intercept, K = k In models with an intercept, K = k + 1 Note: *X is k x k, X does not include vector of 1s, and b is K x 1, coefficient include intercept if there is one. To code the equivalent of X*b, you extract the first k elements of b and add the intercept separately. To make this easier think of b = (b_X, b_c) b_X() returns b_X: 1 x k b_c() returns b_c: 1 x 1 containing b[K] or 0. Thus, X*b can be calculated as (*X)*b_X() :+ b_c() in all cases. */ real colvector linreg::b_X() { if (cons) { if (k()) return( b()[| 1\k() |]) else return( J(0, 1, .)) } else return(b()) } real scalar linreg::b_c() { return(cons ? b()[K()] : 0) } real colvector linreg::b() { if (b == .z) { if (cons) { b = SSinv() * St() b[K()] = mean_y() - mean_X()*b_X() } else b = XXinv() * Xy() } return(b) } real matrix linreg::V() { if (V == .z) { V = s2() * (cons ? SSinv() : XXinv()) } return(V) } real scalar linreg::s2() { if (s2 == .z) { s2 = ee() / ( N() - K_adj() ) } return(s2) } real scalar linreg::ee() { real colvector e if (ee == .z) { e = *y - yhat() ee = quadcross(e, e) } return(ee) } real colvector linreg::yhat() { return( (*X)*b_X() :+ b_c() ) } /* ------------------------------------------internal subroutines --- */ real matrix linreg::XX() { if (XX == .z) { XX = quadcross(*X, cons, *X, cons) } return(XX) } real colvector linreg::Xy() { if (Xy == .z) { Xy = quadcross(*X, cons, *y, 0) } return(Xy) } real scalar linreg::yy() { if (yy == .z) { yy = quadcross(*y, *y) } return(yy) } real matrix linreg::XXinv() { if (XXinv == .z) { XXinv = invsym(XX()) } return(XXinv) } real matrix linreg::SSinv() { if (SSinv == .z) { if (cons) calc_SSinv_cons() } return(SSinv) } void linreg::calc_SSinv_cons() { real scalar K, k, i real rowvector v real matrix kxk, rowK, colK K = K() // # X vars including constant k = k() // # x vars excluding constant /* Calculate inverse of S'S = SS(). This routine called only if cons, meaning K = k + 1. SS() is k x k -- it excludes constant. We want the full K x K inverse. */ // ---------------------------------------- SSinv 1 x 1 case --- if (K==1) { SSinv = 1/N() return } // ----------------------------------- SSinv 2 x 2 or larger --- SSinv = J(K, K, .) // matrix now K x K // Range subscripts: kxk = (1,1 \ k,k) // k x k submatrix rows & cols 1..k rowK = (K,1 \ K,k) // 1 x k submatrix; row K colK = (1,K \ k,K) // k x 1 submatrix; col K SSinv[|kxk |] = invsym(SS()) SSinv[|rowK|] = v = -(mean_X() * SSinv[|kxk|]) SSinv[|colK|] = v' // SSinv[ K,K ] = 1/N() - mean_X()*SSinv[|colK|] SSinv[ K,K ] = 1/N() - mean_X()*v' } real matrix linreg::SS() { if (SS == .z) { if (cons) { SS = quadcrossdev(*X, 0, mean_X(), *X, 0, mean_X() ) } } return(SS) } real colvector linreg::St() { if (St == .z) { St = quadcrossdev(*X, 0, mean_X(), *y, 0, mean_y()) \ 0 } return(St) } real rowvector linreg::mean_X() { if (mean_X == .z) mean_X = mean(*X) return(mean_X) } real scalar linreg::mean_y() { if (mean_y == .z) mean_y = mean(*y) return(mean_y) } real scalar linreg::K_adj() { if (K_adj == .z) { if (cons) K_adj = colsum(diagonal(SSinv()) :!= 0) else K_adj = colsum(diagonal(XXinv()) :!= 0) } return(K_adj) } real scalar linreg::k_adj() { if (k_adj == .z) { k_adj = k() - (K()-K_adj()) } return(k_adj) } end
There are many great resources for learning Mata.
The official documentation:
We recommend starting with the intro sections:
The Mata Book: A Book for Serious Programmers and Those Who Want to Be by William Gould
The Mata Matters articles published in the Stata Journal:
Programming an estimation command in Stata, a series of posts on the Stata Blog.
An Introduction to Mata from the Social Science Computing Cooperative at the University of Madison–Wisconsin
Estimation of the Mixture of Gaussians Using Mata, blog post by Aramayis Dallakyan.
Learn more about all of Mata's features.
Learn
Free webinars
NetCourses
Classroom and web training
Organizational training
Video tutorials
Third-party courses
Web resources
Teaching with Stata
© Copyright 1996–2025 StataCorp LLC. All rights reserved.
×
We use cookies to ensure that we give you the best experience on our website—to enhance site navigation, to analyze usage, and to assist in our marketing efforts. By continuing to use our site, you consent to the storing of cookies on your device and agree to delivery of content, including web fonts and JavaScript, from third party web services.
Cookie Settings
Last updated: 16 November 2022
StataCorp LLC (StataCorp) strives to provide our users with exceptional products and services. To do so, we must collect personal information from you. This information is necessary to conduct business with our existing and potential customers. We collect and use this information only where we may legally do so. This policy explains what personal information we collect, how we use it, and what rights you have to that information.
These cookies are essential for our website to function and do not store any personally identifiable information. These cookies cannot be disabled.
This website uses cookies to provide you with a better user experience. A cookie is a small piece of data our website stores on a site visitor's hard drive and accesses each time you visit so we can improve your access to our site, better understand how you use our site, and serve you content that may be of interest to you. For instance, we store a cookie when you log in to our shopping cart so that we can maintain your shopping cart should you not complete checkout. These cookies do not directly store your personal information, but they do support the ability to uniquely identify your internet browser and device.
Please note: Clearing your browser cookies at any time will undo preferences saved here. The option selected here will apply only to the device you are currently using.