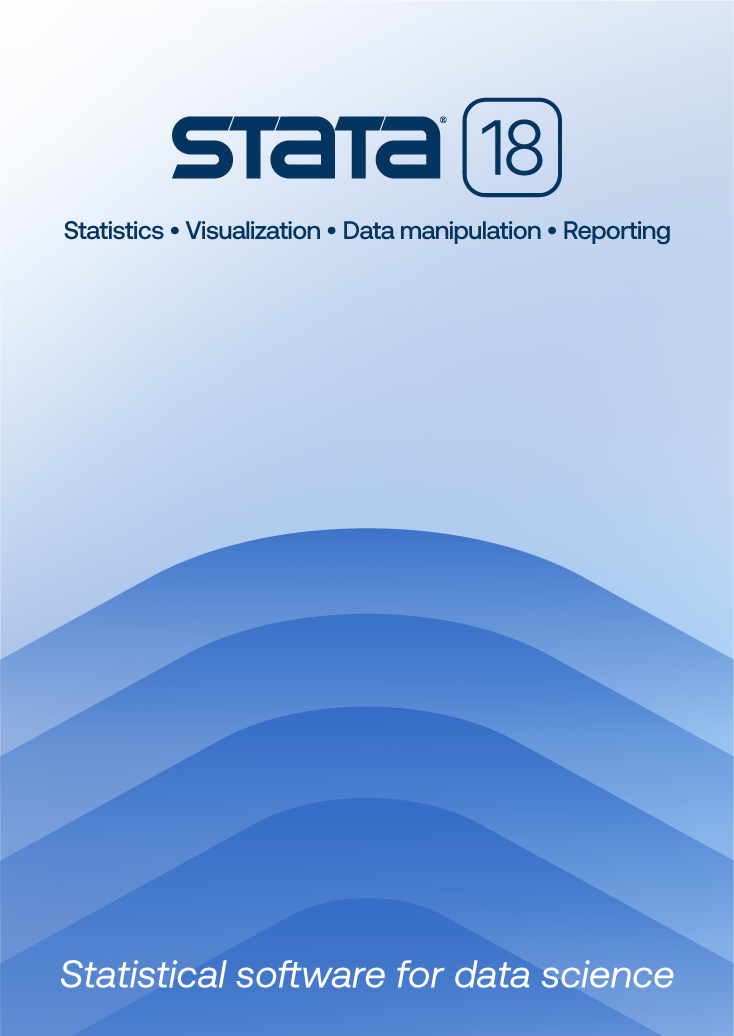
General linear models
Fit one- and two-way models. Or fit models with three, four,
or even more factors. Analyze data with nested factors, with fixed and
random factors, or with repeated measures. Use ANCOVA models when you have
continuous covariates and MANOVA models when you have multiple outcome
variables.
Further explore the relationships between your outcome and
predictors by estimating effect sizes and computing least-squares and marginal
means. Perform contrasts and pairwise comparisons. Analyze and plot
interactions.
Linear, binary, and count regressions
Fit classical ANOVA and linear regression models of the relationship between a
continuous outcome, such as weight, and the determinants of weight, such as
height, diet, and level of exercise. If your response is binary, ordinal,
categorical, or count, don't worry.
Stata has estimators for these types of
outcomes too. Use logistic regression to estimate odds ratios. Estimate
incidence rates using a Poisson model. Analyze matched case–control data with
conditional logistic regression. A vast array of tools is available after
fitting such models. Predict outcomes and their confidence intervals. Test
equality of parameters. Compute linear and nonlinear combinations of
parameters.
Power, precision, and sample size
Before you conduct your experiment, determine the sample size needed to detect
meaningful effects without wasting resources. Do you intend to compute CIs for
means or variances or perform tests for proportions or correlations? Do you
plan to fit a Cox proportional hazards model or compare survivor functions
using a log-rank test? Do you want to use a Cochran—Mantel—Haenszel test of
association or a Cochran—Armitage trend test? Use Stata's
power command to
compute power and sample size, create customized tables, and automatically
graph the relationships between power, sample size, and effect size for your
planned study. Or use the ciwidth
command to do the same but for CIs instead
of hypothesis tests by computing the required sample size for the desired CI
precision. Or use gsdesign
to compute stopping boundaries and the required sample sizes for group sequential
designs. Instead of commands, use the interactive Control Panel to perform your analysis.
Marginal means, contrasts, and interactions
Marginal means and contrasts let you analyze the relationships between your
outcome variable and your covariates, even when that outcome is binary, count,
ordinal, categorical, or survival. Compute adjusted predictions with covariates
set to interesting or representative values.
Or compute marginal means for
each level of a categorical covariate. Make comparisons of the adjusted
predictions or marginal means using contrasts. If you have multilevel data
and random effects, these effects are automatically integrated out to provide
marginal (that is, population-averaged) estimates. After fitting almost any
model in Stata, analyze the effect of covariate interactions, and easily create
plots to visualize those interactions.
Multilevel mixed-effects models
Whether the groupings in your data arise in a nested fashion (patients nested
in clinics and clinics nested in regions) or in a nonnested fashion (regions
crossed with occupations), you can fit a multilevel model to account for the
lack of independence within these groups.
Fit models for continuous, binary,
count, ordinal, and survival outcomes. Estimate variances of random intercepts
and random coefficients. Compute intraclass correlations. Predict random
effects. Estimate relationships that are population averaged over the random
effects.
Meta-analysis
Combine results of multiple studies to estimate an overall effect. Use
forest plots to visualize results. Use subgroup analysis and
meta-regression to explore study heterogeneity. Use funnel plots and
formal tests to explore publication bias and small-study effects. Use
trim-and-fill analysis to assess the impact of publication bias on
results. Perform cumulative and leave-one-out meta-analysis. Perform
univariate, multilevel, and multivariate meta-analysis. Use the meta suite, or let the Control Panel interface
guide you through your entire meta-analysis.
Multiple imputation
Account for missing data in your sample using multiple imputation. Choose from
univariate and multivariate methods to impute missing values in continuous,
censored, truncated, binary, ordinal, categorical, and count variables.
Then, in a single step, estimate parameters using the imputed datasets, and combine
results. Fit a linear model, logit model, Poisson model, hierarchical model,
survival model, or one of the many other supported models. Use the mi command,
or let the Control Panel interface guide you through your entire MI analysis.
Survival analysis
Analyze duration outcomes—outcomes measuring the time to an event
such as failure or death—using Stata's specialized tools for
survival analysis. Account for the complications inherent in survival
data, such as sometimes not observing the event (right-, left-, and
interval-censoring), individuals entering the study at differing times
(delayed entry), and individuals who are not continuously observed
throughout the study (gaps). You can estimate and plot the probability
of survival over time. Or model survival as a function of covariates
using Cox, Weibull, lognormal, and other regression models. Predict
hazard ratios, mean survival time, and survival probabilities. Do you
have groups of individuals in your study? Adjust for within-group
correlation with a random-effects or shared-frailty model. If you have
many potential covariates, use lasso cox and
elasticnet cox for model selection and prediction.
Epidemiological tables
Want to analyze data from a prospective (incidence) study, cohort study,
case–control study, or matched case–control study? Stata's tables for
epidemiologists make it easy to summarize your data and compute statistics
such as incidence-rate ratios, incidence-rate differences, risk ratios, risk
differences, odds ratios, and attributable fractions. You can analyze
stratified data too—compute Mantel–Haenszel combined estimates, perform
tests of homogeneity, and standardize estimates. If you have an ordinal rather
than binary exposure, you can perform a test for a trend.
Additive models of relative risk
Determine how exposures interact to put subjects at a higher risk of
experiencing an outcome of interest. For example, you might be
investigating how exposure to cigarette smoke and asbestos interact to
increase the risk of lung cancer. With Stata's reri command, you
can measure two–way interactions in an additive model of relative
risk, while accounting for other risk factors. Choose from various
supported models, such as binomial generalized linear, Poisson, negative
binomial, logistic, Cox, parametric survival, and
interval–censored parametric and semiparametric survival models.
Estimate the relative excess risk due to interaction (RERI),
attributable proportion (AP), and synergy index (SI).
Automated reporting and customizable tables
Stata is designed for reproducible research, including the ability to
create dynamic documents incorporating your analysis results. Create
Word or PDF files, populate Excel worksheets with results and format
them to your liking, and mix Markdown, HTML, Stata results, and Stata
graphs, all from within Stata. Create tables that compare
regression results or summary statistics, use default styles
or apply your own, and export your tables to Word, PDF, HTML, LaTeX,
Excel, or Markdown and include them in your reports.
Jupyter Notebook with Stata
Jupyter Notebook is widely used by
researchers and scientists to share their ideas and results for collaboration
and innovation. It is an easy-to-use web application that allows you to
combine code, visualizations, mathematical formulas, narrative text, and other
rich media in a single document (a "notebook") for interactive computing and
developing. You can invoke Stata and Mata from Jupyter Notebook with the
IPython (interactive Python) kernel. This
means you can combine the capabilities of both Python and Stata in a single
environment to make your work easily reproducible and shareable with others.
I've used a lot of stat packages over the years, but I find that I'm using Stata 95% of the time now. It's wonderful! Its speed and power are much touted, but its simplicity for beginners is perhaps one of its best features.
— Rodney Hayward
University of Michigan's Schools of Medicine & Public Health, Ann
Arbor VA's Center for Clinical Management Research
Intuitive and easy to use.
Once you learn the syntax of one estimator, graphics command,
or data management tool, you will effortlessly understand the rest.
Accuracy and reliability.
Stata is extensively and continually tested. Stata's tests produce
approximately 6 million lines of output. Each of those lines is
compared against known-to-be-accurate results
across editions of Stata and every operating system Stata supports to
ensure accuracy and reproducibility.
One package. No modules.
When you buy Stata, you obtain
everything for your statistical,
graphical, and data analysis needs. You do not need to buy separate modules
or import your data to specialized software.
Write your own Stata programs.
You can easily write your own Stata programs and commands. Share them
with others or use them to simplify your work. Utilize Stata's
do-files, ado-files, and Mata: Stata's own advanced programming
language that adds direct support for matrix programming. You can also
access and benefit from the thousands of existing Stata
community-contributed programs.
Extensive documentation.
Stata offers 35 manuals with more than 18,000 pages of PDF documentation
containing detailed examples, in-depth discussions, references to relevant literature,
and methods and formulas. Stata's documentation is a great place to learn about
Stata and the statistics, graphics, data management, and data science tools you
are using for your research.
Top-notch technical support.
Stata's technical support is known for their prompt, accurate,
detailed, and clear responses. People answering your questions have master's
and PhD degrees in relevant areas of research.
Join us for one of our free live webinars. Ready. Set. Go Stata shows you how to quickly get started manipulating, graphing, and analyzing your data. Or, go deeper in one of our special-topics webinars.
Stata's YouTube has over 300 videos with a dedicated playlist of methodologies important to medical researchers. And they are a convenient teaching aid in the classroom.
Get started quickly at using Stata effectively, or even learn how to perform rigorous time-series, panel-data, or survival analysis, all from the comfort of you home or office. NetCourses make it easy.
Stata Press offers books with clear, step-by-step examples that make teaching easier and that enable students to learn and medical researchers to implement the latest best practices in analysis.