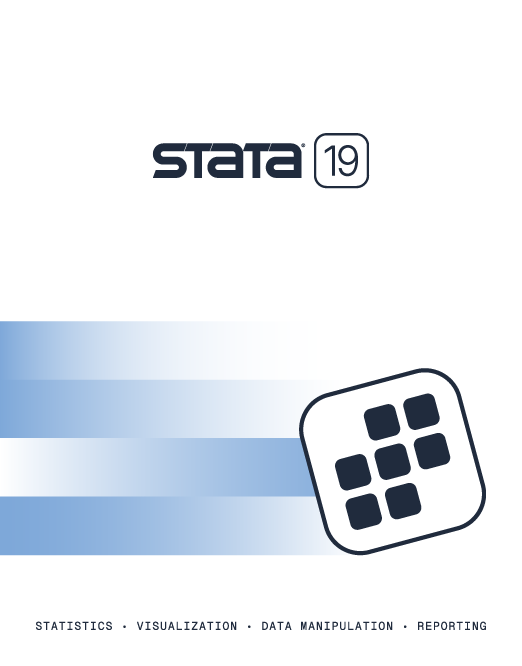
2025 Stata Conference • Nashville, TN • 31 July–01 August
Time Series Analysis for the Social Sciences |
||||||||||||||||||||||||||||||||||||
![]() Click to enlarge See the back cover |
![]()
As an Amazon Associate, StataCorp earns a small referral credit from
qualifying purchases made from affiliate links on our site.
eBook not available for this title
eBook not available for this title |
|
||||||||||||||||||||||||||||||||||
Comment from the Stata technical groupTime Series Analysis for the Social Sciences provides a rigorous yet accessible introduction to time-series analysis. The book emphasizes the interpretation of the results and the intuition behind the techniques rather than technical details. The methodologies presented in the book are accompanied with examples that illustrate the concepts developed using real data. The first part of the book presents univariate time-series models. The book subsequently incorporates the concepts of univariate time series in the context of dynamic social interactions. The second part of the book provides introductions to multivariate time series, stationarity, and cointegration methods. The book also touches on more advanced topics such as forecasting and structural breaks. Time Series Analysis for the Social Sciences is ideal for researchers and students who want an intuitive introduction to time series. The intuitive discussions linking time-series methods and the dynamic social-science process make this book uniquely instructive. |
||||||||||||||||||||||||||||||||||||
Table of contentsView table of contents >> Preface
1. Modeling Social Dynamics
1.1 Time Series Analysis
1.1.1 Contending Approaches and the Quasi-Experimental Tradition
1.2 Challenges in Time Series Modeling
1.1.2 The Architecture of the Book
1.2.1 Time Series Measurement
1.3 The First Step 1.2.2 Fit: On the Use of Time Trends and Counters 1.2.3 Discerning Structural Change 1.4 For Further Reading 2. Univariate Time Series Models
2.1 Understanding Univariate Processes
2.1.1 Why Stochastic Models of Time Series?
2.2 Univariate Processes
2.1.2 White Noise 2.1.3 A Note on Notation
2.2.1 The Autoregressive (AR) Process
2.3 Diagnosing Stationary Univariate Processes
2.2.2 The Moving Average (MA) Process 2.2.3 The Equivalence of AR and MA Processes
2.3.1 The Autocorrelation Function (ACF)
2.4 Model Estimation, Interpretation, and Diagnosis
2.3.2 The Partial Autocorrelation Function (PACF) 2.3.3 Seasonality
2.4.1 Residual Analysis
2.5 Application: U.S. Homicide Rates 2.4.2 Metadiagnosis 2.6 Application: U.S. Relations with the Middle East 2.7 Intervention Models
2.7.1 Impact Assessment: Continuing the Middle East Example
2.8 Conclusion 2.7.2 Structural Breaks 2.9 For Further Reading 3. Dynamic Regression Models
3.1 Variations of the General Dynamic Regression Model
3.1.1 Building Blocks
3.2 Obtaining Consistent Estimates
3.1.2 Autoregressive Models/Lagged Endogenous Variables 3.1.3 The Workhorse Model 3.1.4 Serial Correlation: Tests and Solutions
3.2.1 Approach Number One: Pseudo-GLS Estimation
3.3 Illustration: Explaining Voting Intentions in the United Kingdom
3.2.2 Approach Number Two: Hatanaka's Method
3.3.1 Voting Intentions and Exchange Rates: Results
3.4 Dynamic Regression Models with Multiple Causal Variable Lags
3.4.1 Distributed Lag Models
3.5 Conclusion 3.4.2 Autoregressive Distributed Lag Models 3.6 For Further Reading 4. Modeling the Dynamics of Social Systems
4.1 Two Approaches to Multivariate Time Series Analysis
4.2 The Structural Equation Approach
4.2.1 The Workhorse Model of Time Series Regression Revisited
4.3 Vector Autoregression
4.2.2 The Structural Equation Approach in Practice 4.2.3 Application: The Political Economy of Monetary Policy in the United Kingdom
4.3.1 Terminology and Stationarity
4.4 The Political Economy of Monetary Policy in the United Kingdom
Revisited 4.3.2 Specification and Estimation 4.3.3 Tools for Inference in VAR Modeling 4.3.4 VAR Modeling in Practice 4.5 Conclusion: SEQ and VAR Modeling Compared 4.6 For Further Reading 5. Univariate, Nonstationary Processes: Tests and Modeling
5.1 Stationary Data
5.2 Stationarity and Unit Root Tests
5.2.1 Dickey-Fuller and Augmented Dickey-Fuller Tests
5.3 Application: Macropartisanship
5.2.2 Variance Ratio Test 5.2.3 Modified Rescaled Range Test 5.2.4 KPSS Test 5.2.5 Double Unit Roots
5.3.1 Macropartisanship: Diagnostic Plots
5.4 Conclusion 5.3.2 Macropartisanship: Formal Statistical Tests 5.3.3 Macropartisanship: An ARIMA Model 5.5 For Further Reading 6. Cointegration and Error Correction Models
6.1 Introduction
6.2 The Intuition behind Cointegration 6.3 Estimating an ECM
6.3.1 Engle-Granger Regression-Based Approach
6.4 ECMs for Non-Integrated Data? 6.3.2 Johansen's VAR Approach 6.3.3 Application: The Engle-Granger Methodology and the Indo-Pakistani Arms Race 6.5 Conclusion 6.6 For Further Reading 7. Selections on Time Series Analysis
7.1 Fractional Integration
7.1.1 Fractional Cointegration
7.2 Incorporating Heterogeneity 7.1.2 Near and Fractional Integration 7.3 Forecasting
7.3.1 Purpose and Definitions
7.4 Estimating and Modeling with Unknown Structural Breaks
7.3.2 Approaches to Forecasting 7.3.3 Criteria for Determining Optimum Forecast 7.3.4 Illustration: Forecasts of the U.S. Uniform Crime Reports Homicide Data, 1975-1993 7.3.5 Forecasting Discussion
7.4.1 Identifying a Single Unknown Structural Break
7.5 Conclusion 7.4.2 Identifying Multiple Unknown Structural Breaks 7.4.3 Hypothesis Tests for Multiple Structural Breaks 7.6 For Further Reading 8. Concluding Thoughts for the Time Series Analyst
8.1 A Review
8.2 Looking Ahead Appendix: Time Series Models as Difference Equations
A.1 Introduction
A.2 The Nature of Difference Equation Models
A.2.1 Expressing Difference Equations
A.3 Finding the Solutions of Difference Equation Models
A.2.2 The Solutions of Difference Equations
A.3.1 Solving the Most Simple First-Order Difference Equations
A.4 Bring on the Data
A.3.2 Solving More Complicated Difference Equations A.3.3 Solving Systems of Difference Equations Bibliography Index
|
Learn
Free webinars
NetCourses
Classroom and web training
Organizational training
Video tutorials
Third-party courses
Web resources
Teaching with Stata
© Copyright 1996–2025 StataCorp LLC. All rights reserved.
×
We use cookies to ensure that we give you the best experience on our website—to enhance site navigation, to analyze usage, and to assist in our marketing efforts. By continuing to use our site, you consent to the storing of cookies on your device and agree to delivery of content, including web fonts and JavaScript, from third party web services.
Cookie Settings
Last updated: 16 November 2022
StataCorp LLC (StataCorp) strives to provide our users with exceptional products and services. To do so, we must collect personal information from you. This information is necessary to conduct business with our existing and potential customers. We collect and use this information only where we may legally do so. This policy explains what personal information we collect, how we use it, and what rights you have to that information.
These cookies are essential for our website to function and do not store any personally identifiable information. These cookies cannot be disabled.
This website uses cookies to provide you with a better user experience. A cookie is a small piece of data our website stores on a site visitor's hard drive and accesses each time you visit so we can improve your access to our site, better understand how you use our site, and serve you content that may be of interest to you. For instance, we store a cookie when you log in to our shopping cart so that we can maintain your shopping cart should you not complete checkout. These cookies do not directly store your personal information, but they do support the ability to uniquely identify your internet browser and device.
Please note: Clearing your browser cookies at any time will undo preferences saved here. The option selected here will apply only to the device you are currently using.