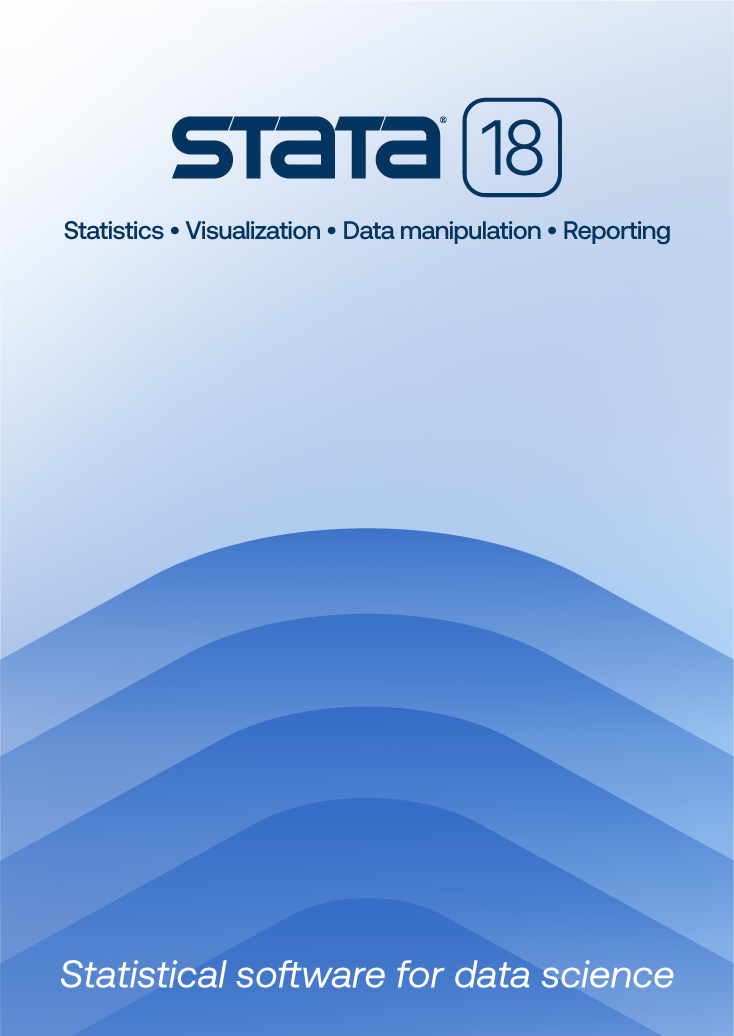
Statistical Tools for Epidemiologic Research |
||||||||||||||||||||||||||||||||||||
![]() Click to enlarge See the back cover |
![]()
As an Amazon Associate, StataCorp earns a small referral credit from
qualifying purchases made from affiliate links on our site.
eBook not available for this title
eBook not available for this title |
|
||||||||||||||||||||||||||||||||||
Comment from the Stata technical groupThis is a new book from the author of the acclaimed Statistical Analysis of Epidemiologic Data. It is intended for a second course in statistical methods. Calculus is used occasionally, but most of the text requires only a knowledge of elementary mathematics. Stata datasets and output are available at the book's website. The book is mostly devoted to regression modeling, with separate chapters on logistic regression, Poisson regression, and conditional logistic regression. The chapters emphasize confounding, interaction, and connections to the two-by-two table. There are also chapters on topics not often covered at this level, including misclassification, longitudinal data analysis, and smoothing. |
||||||||||||||||||||||||||||||||||||
Table of contentsView table of contents >> 1. Two Measures of Risk: Odds Ratios and Average Rates
Odds Ratio
Properties of the Odds Ratio Three Statistical Terms Average Rates
Geometry of an Average Rate
Proportionate Mortality “Rates” 2. Tabular Data: The 2 × k Table and Summarizing
2 × 2 Tables
The 2 × k Table
Independence/Homogeneity
Independence
Regression Homogeneity Two-Sample: Comparison of Two Mean Values An Example: Childhood Cancer and Prenatal X-ray Exposure Summary of the Notation for a 2 × k Table Summarizing 2 × 2 Tables: Application of a Weighted Average Another Summary Measure: Difference in Proportions
Confounding
3. Two Especially Useful Estimation Tools
Maximum Likelihood Estimation
Four Properties of Maximum Likelihood Estimates Likelihood Statistics The Statistical Properties of a Function
Application 1: Poisson Distribution
Application 2: Variance of a Logarithm of a Variable Application 3: Variance of the Logarithm of a Count 4. Linear Logistic Regression: Discrete Data
The Simplest Logistic Regression Model: The 2 × 2 Table
The Logistic Regression Model: The 2 × 2 × 2 Table Additive Logistic Regression Model A Note on the Statistical Power to Identify Interaction Effects The Logistic Regression Model: The 2 × k Table The Logistic Regression Model: Multivariable Table Goodness-of-Fit: Multivariable Table Logistic Regression Model: The Summary Odds Ratio Description of the WCGS Data Set 5. Logistic Regression: Continuous Data
Four Basic Properties of Multivariable Regression Model Analysis
Additivity
Logistic Regression AnalysisConfounding Influence The Geometry of Interaction and Confounding The Geometry of Statistical Adjustment 6. Analysis of Count Data: Poisson Regression Model
Poisson Multivariable Regression Model: Technical Description
Illustration of the Poisson Regression Model Poisson Regression Model: Hodgkin Disease Mortality The Simplest Poisson Regression Model: The 2 × 2 Table Application of the Poisson Regression Model: Categorical Data Application of the Poisson Regression Model: Count Data Poisson Regression Example: Adjusted Perinatal Mortality Rates
First Approach: Weight-Specific Comparisons
Second Approach: A Model-Free Summary Third Approach: Poisson Regression Model 7. Analysis of Matched Case–Control Data
The 2 × 2 Case–Control Table
Odds Ratio for Matched Data Confidence Interval for the Matched-Pairs Odds Ratio Evaluating an Estimated Odds Ratio Disregarding the Matched Design Interaction with the Matching Variable Matched Pairs Analysis: More than One Control Matched Analysis: Multilevel Categorical Risk Factor Conditional Analysis of Logistic Regression Models Conditional Logistic Analysis: Binary Risk Factor Multiple Controls per Case Conditional Logistic Analysis: A Bivariate Regression Model Conditional Logistic Analysis: Interactions with the Matching Variable Conditional Logistic Analysis: k-Level Category Risk Variable Conditional Logistic Analysis: Continuous Variables Additive Logistic Regression Model 8. Spatial Data: Estimation and Analysis
Poisson Probability Distribution: An Introduction
Nearest-Neighbor Analysis Comparison of Cumulative Probability Distribution Functions Randomization Test Bootstrap Estimation
Example: Bootstrap Estimation of a Percentage Decrease
Properties of the Odds Ratio and the Logarithm of an Odds Ratio Estimation of ABO Allele Frequencies An Important Property (Bootstrap versus Randomization) A Last Example: Assessment of Spatial Data 9. Classification: Three Examples
Dendogram Classification
Principal Component Summaries Genetic Classification A Multivariate Picture 10. Three Smoothing Techniques
Smoothing: A Simple Approach
Kernel Density Estimation Spline Estimated Curves
Data Analysis with Spline-Estimated Curves: An Example
11. Case Study: Description and Analysis
12. Longitudinal Data Analysis
Within and Between Variability
A Simple Example Elementary Longitudinal Models: Polynomial Models Elementary Longitudinal Models: Spline Models Random Intercept Model Random Intercept and Random Slope Regression Model Mechanics of a Variance/Covariance Array 13. Analysis of Multivariate Tables
Analysis of Ordinal Data
Wilcoxon (Mann–Whitney) Rank Sum Test Correlation Between Ordinal Variables Log–Linear Models: Categorical Data Analysis
Independence in a Two-Way Table
Tables with Structural Zeros Capture/Recapture Model Categorical Variable Analysis from Matched Pairs Data Quasi-Independence: Association in a R × C Table The Analysis of a Three-Way Table Complete Independence Joint Independence Conditional Independence Additive Measures of Association 14. Misclassification: A Detailed Description of a Simple Case
Example: Misclassification of the Disease Status
Example: Misclassification of the Risk Factor Status A Few Illustrations of Misclassification Agreement Between Two Methods of Classification: Categorical Data Disagreement A Measurement of Accuracy: Continuous Data
Parametric Approach
Agreement Between Two Methods of Measurement: Continuous Data Nonparametric Approach A Detailed Example of a Nonparametric ROC Curve Area Under the ROC Curve Application: ROC Analysis Applied to Carotid Artery Disease Data
A Statistical Model: “Two-Measurement” Model
Another Application of Perpendicular Least-Squares Estimation An Alternative Approach: Bland–Altman Analysis 15. Advanced Topics
Confidence Intervals
An Example of a Bivariate Confidence Region Confidence Band Nonparametric Regression Methods Bivariate Loess Estimation Two-Dimensional Kernel Estimation Statistical Tests and a Few of Their Properties
Power of a Specific Statistical Test: The Normal Distribution Case
Power of a Statistical Test: The Chi-Square Distribution Case
Three Applications
Multiple Statistical Tests: Accumulation of Type I Errors
References
Index
|
Learn
Free webinars
NetCourses
Classroom and web training
Organizational training
Video tutorials
Third-party courses
Web resources
Teaching with Stata
© Copyright 1996–2024 StataCorp LLC. All rights reserved.
×
We use cookies to ensure that we give you the best experience on our website—to enhance site navigation, to analyze usage, and to assist in our marketing efforts. By continuing to use our site, you consent to the storing of cookies on your device and agree to delivery of content, including web fonts and JavaScript, from third party web services.
Cookie Settings
Last updated: 16 November 2022
StataCorp LLC (StataCorp) strives to provide our users with exceptional products and services. To do so, we must collect personal information from you. This information is necessary to conduct business with our existing and potential customers. We collect and use this information only where we may legally do so. This policy explains what personal information we collect, how we use it, and what rights you have to that information.
These cookies are essential for our website to function and do not store any personally identifiable information. These cookies cannot be disabled.
This website uses cookies to provide you with a better user experience. A cookie is a small piece of data our website stores on a site visitor's hard drive and accesses each time you visit so we can improve your access to our site, better understand how you use our site, and serve you content that may be of interest to you. For instance, we store a cookie when you log in to our shopping cart so that we can maintain your shopping cart should you not complete checkout. These cookies do not directly store your personal information, but they do support the ability to uniquely identify your internet browser and device.
Please note: Clearing your browser cookies at any time will undo preferences saved here. The option selected here will apply only to the device you are currently using.