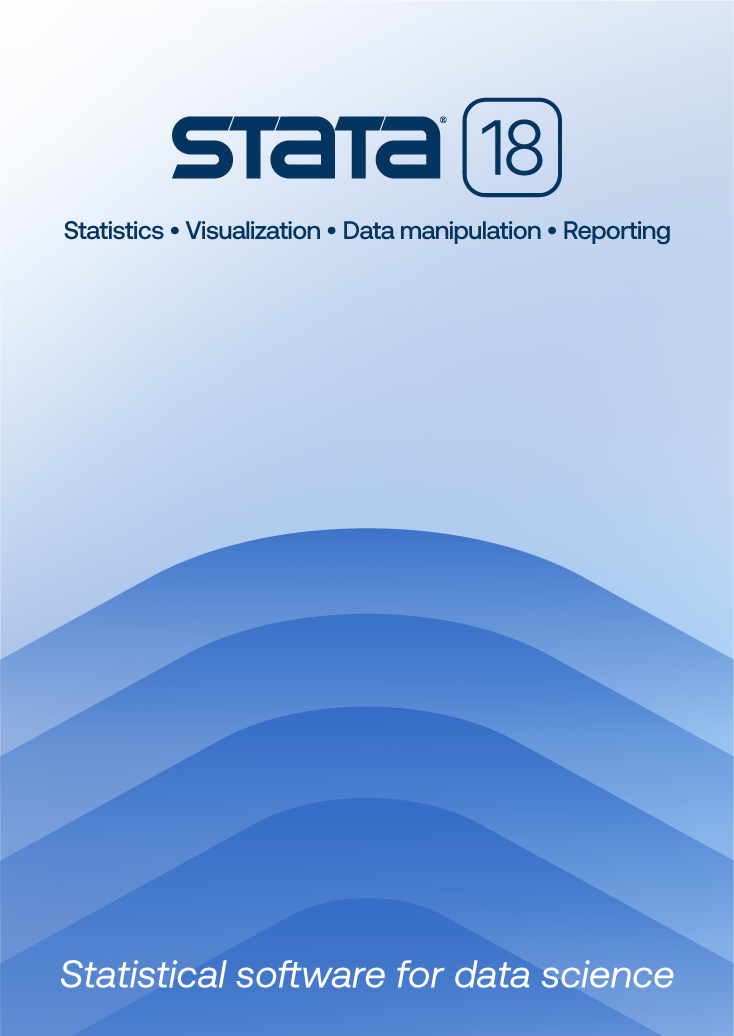
The Statistical Evaluation of Medical Tests for Classification and Prediction |
||||||||||||||||||||||||||||||||||||||
![]() Click to enlarge See the back cover |
![]()
As an Amazon Associate, StataCorp earns a small referral credit from
qualifying purchases made from affiliate links on our site.
eBook not available for this title
eBook not available for this title |
|
||||||||||||||||||||||||||||||||||||
Comment from the Stata technical groupThis book begins with an overview of clinical studies: their purpose, the two basic types of sample selection, paired and unpaired observations, internal validity, and sources of bias. Eight datasets that serve as motivation for examples are then introduced. The next three chapters of the book discuss the different methods for measuring accuracy, including the receiver operating characteristic (ROC) curve. The rest of the book discusses estimating these measures of accuracy, accounting for covariates, dealing with various forms of bias, and the phases of research for a medical test. Each chapter contains concluding remarks and exercises for readers to test their understanding of the material. Although the text itself does not show how to use Stata to reproduce results presented in the book, the book provides access to a website that contains Stata datasets and programs for that purpose. |
||||||||||||||||||||||||||||||||||||||
Table of contentsView table of contents >> 1 Introduction
1.1 The medical test
1.1.1 Tests, classification and the broader context
1.2 Elements of study design1.1.2 Disease screening versus diagnosis 1.1.3 Criteria for a useful medical test
1.2.1 Scale for the test result
1.3 Examples and datasets1.2.2 Selection of study subjects 1.2.3 Comparing tests 1.2.4 Test integrity 1.2.5 Sources of bias
1.3.1 Overview
1.4 Topics and organization1.3.2 The CASS dataset 1.3.3 Pancreatic cancer serum biomarkers study 1.3.4 Hepatitis metastasis ultrasound study 1.3.5 CARET PSA biomarker study 1.3.6 Ovarian cancer gene expression study 1.3.8 Neonatal audiology data 1.3.9 St Louis prostate cancer screening study 1.5 Exercises 2 Measures of accuracy for binary tests
2.1 Measures of accuracy
2.1.1 Notation
2.2 Estimating accuracy with data 2.2.2 Disease-specific classification probabilities 2.2.3 Predictive values 2.2.4 Diagnostic likelihood ratios
2.2.1 Data from a cohort study
2.3 Quantifying the relative accuracy of tests 2.2.2 Proportions: (FPF, TPF) and (PPV, NPV) 2.2.3 Ratios of proportions: DLRs 2.2.4 Estimation from a case–control study 2.2.5 Merits of case–control versus cohort studies
2.3.1 Comparing classification probabilities
Concluding remarks 2.3.2 Comparing predictive values 2.3.3 Comparing diagnostic likelihood ratios 2.3.4 Which test is better? Exercises 3 Comparing binary tests and regression analysis
3.1 Study designs for comparing tests
3.1.1 Unpaired designs
3.2 Comparing accuracy with unpaired data 3.1.2 Paired designs
3.2.1 Empirical estimators of comparative measures
3.3 Comparing accuracy with paired data 3.2.2 Large sample inference
3.3.1 Sources of correlation
3.4 The regression modeling framework 3.3.2 Estimation of comparative measures 3.3.3 Wide of long data representations 3.3.4 Large sample inference 3.3.5 Efficiency of paired versus unpaired designs 3.3.6 Small sample properties 3.3.7 The CASS study
3.4.1 Factors potentially affecting test performance
3.5 Regression for true and false positive fractions 3.4.2 Questions addressed by regression modeling 3.4.3 Notation and general set-up
3.5.1 Binary marginal GLM models
3.6 Regression modeling of predictive values 3.5.2 Fitting marginal models to data 3.5.3 Illustration: Factors affecting test accuracy 3.5.4 Comparing tests with regression analysis
3.6.1 Model formulation and fitting
3.7 Regression models for DLRs 3.6.2 Comparing tests 3.6.3 The incremental value of a test for prediction
3.7.1 The model form
3.8 Concluding remarks 3.7.2 Fitting the DLR model 3.7.3 Comparing DLRs of two tests 3.7.4 Relationships with other regression models 3.9 Exercises 4 The receiver operating characteristic curve
4.1 The context
4.1.1 Examples of non-binary tests
4.2 The ROC curve for continuous tests 4.1.2 Dichotomizing the test result
4.2.1 Definition of the ROC
4.3 Summary indices 4.2.2 Mathematical Properties of the ROC curve 4.2.3 Attributes of and uses for the ROC curve 4.2.4 Restrictions and alternatives to the ROC curve
4.3.1 The area under the ROC curve (AUC)
4.4 The binormal ROC curve 4.3.2 The ROC (t0) and partial AUC 4.3.3 Other summary indices 4.3.4 Measures of distance between distributions
4.4.1 Functional form
4.5 The ROC for ordinal tests 4.4.2 The binormal AUC 4.4.3 The binormal assumption
4.5.1 Tests with ordered discrete results
4.6 Concluding remarks 4.5.2 The latent decision variable model 4.5.3 Identification of the latent variable ROC 4.5.4 Changes in accuracy versus thresholds 4.5.5 The discrete ROC curve 4.5.6 Summary measures for the discrete ROC curve 4.7 Exercises 5 Estimating the ROC curve
5.1 Introduction
5.1.1 Approaches
5.2 Empirical estimation 5.1.2 Notation and assumptions
5.2.1 The empirical estimator
5.3 Modeling the test result distributions 5.2.2 Sampling variability at a threshold 5.2.3 Sampling variability of RÔCe (t) 5.2.4 The empirical AUC and other indices 5.2.5 Variability in the empirical AUC 5.2.6 Comparing empirical ROC curves 5.2.7 Illustration: pancreatic cancer biomarkers 5.2.8 Discrete ordinal data ROC curves
5.3.1 Fully parametric modeling
5.4 Parametric distribution-free methods: ordinal tests 5.3.2 Semiparametric location-scale models 5.3.3 Arguments against modeling test results
5.4.1 The binormal latent variable framework
5.5 Parametric distribution-free methods: continuous tests 5.4.2 Fitting the discrete binormal ROC function 5.4.3 Generalizations and comparisons
5.5.1 LABROC
5.6 Concluding remarks 5.5.2 The ROC–GLM estimator 5.5.3 Inference with parametric distribution-free methods 5.7 Exercises 5.8 Proofs of theoretical results 6 Covariate effects on continuous and ordinal tests
6.1 How and why?
6.1.1 Notation
6.2 Reference distributions 6.1.2 Aspects to model 6.1.3 Omitting covariates/pooling data
6.2.1 Non-diseased as the reference population
6.3 Modeling covariate effects on test results 6.2.2 The homogenous population 6.2.3 Nonparametric regression quantiles 6.2.4 Parametric estimation of SD,Z 6.2.5 Semiparametric models 6.2.6 Application 6.2.7 Ordinal test results
6.3.1 The basic idea
6.4 Modeling covariate effects on ROC curves 6.3.2 Induced ROC curves for continuous tests 6.3.3 Semiparametric location-scale families 6.3.4 Induced ROC curves for ordinal tests 6.3.5 Random effect models for test results
6.4.1 The ROC–GLM regression model
6.5 Approaches to ROC regression 6.4.2 Fitting the model to data 6.4.3 Comparing ROC curves 6.4.4 Three examples
6.5.1 Modeling ROC summary indices
6.6 Concluding remarks 6.5.2 A qualitative comparison 6.7 Exercises 7 Incomplete data and imperfect reference tests
7.1 Verification biased sampling
7.1.1 Context and definition
7.2 Verification restricted to screen positives 7.1.2 The missing at random assumption 7.1.3 Correcting for bias with Bayes' theorem 7.1.4 Inverse probability weighting/imputation 7.1.5 Sampling variability of corrected estimates 7.1.6 Adjustments for other biasing factors 7.1.7 A broader context 7.1.8 Non-binary tests
7.2.1 Extreme verification bias
7.3 Imperfect reference tests 7.2.2 Identifiable parameters for a single test 7.2.3 Comparing tests 7.2.4 Evaluating covariate effects on (DP, FP) 7.2.5 Evaluating covariate effects on (TPF, FPF) and on prevalence 7.2.6 Evaluating covariate effects on (rTPF, rFPF) 7.2.7 Alternative strategies
7.3.1 Examples
7.4 Concluding remarks 7.3.2 Effects on accuracy parameters 7.3.3 Classic latent class analysis 7.3.4 Relaxing the conditional independence assumption 7.3.5 A critique of latent class analysis 7.3.6 Discrepant resolution 7.3.7 Composite reference standards 7.5 Exercises 7.6 Proofs of theoretical results 8 Study Design and Hypothesis Testing
8.1 The phases of medical test development
8.1.1 Research as a process
8.2 Sample sizes for phase 2 studies 8.1.2 Five phases for the development of a medical test
8.2.1 Retrospective validation of a binary test
8.3 Sample sizes for phase 3 studies 8.2.2 Retrospective validation of a continuous test 8.2.3 Sample size based on the AUC 8.2.4 Ordinal tests
8.3.1 Comparing two binary tests—paired data
8.4 Sample sizes for phase 4 studies 8.3.2 Comparing two binary tests—unpaired data 8.3.3 Evaluating population effects on test performance 8.3.4 Comparisons with continuous test results 8.3.5 Estimating the threshold for screen positivity 8.3.6 Remarks on phase 3 analyses
8.4.1 Designs for inference about (FPF, TPF)
8.5 Phase 5 8.4.2 Designs for predictive values 8.4.3 Designs for (FP, DP) 8.4.4 Selected verification of screen negatives 8.6 Matching and stratification
8.6.1 Stratification
8.7 Concluding remarks 8.6.2 Matching 8.8 Exercises 9 More topics and conclusions
9.1 Meta-analysis
9.1.1 Goals of meta-analysis
9.2 Incorporating the time dimension 9.1.2 Design of a meta-analysis study 9.1.3 The summary ROC curve 9.1.4 Binomial regression models
9.2.1 The context
9.3 Combining multiple test results 9.2.2 Incident cases and long-term controls 9.2.3 Interval cases and controls 9.2.4 Predictive values 9.2.5 Longitudinal measurements
9.3.1 Boolean combinations
9.4 Concluding remarks 9.3.2 The likelihood ratio principle 9.3.3 Optimality of the risk score 9.3.4 Estimating the risk score 9.3.5 Development and assessment of the combination score
9.4.1 Topics we only mention
9.5 Exercises 9.4.2 New applications and new technologies Bibliography
Index
|
Learn
Free webinars
NetCourses
Classroom and web training
Organizational training
Video tutorials
Third-party courses
Web resources
Teaching with Stata
© Copyright 1996–2025 StataCorp LLC. All rights reserved.
×
We use cookies to ensure that we give you the best experience on our website—to enhance site navigation, to analyze usage, and to assist in our marketing efforts. By continuing to use our site, you consent to the storing of cookies on your device and agree to delivery of content, including web fonts and JavaScript, from third party web services.
Cookie Settings
Last updated: 16 November 2022
StataCorp LLC (StataCorp) strives to provide our users with exceptional products and services. To do so, we must collect personal information from you. This information is necessary to conduct business with our existing and potential customers. We collect and use this information only where we may legally do so. This policy explains what personal information we collect, how we use it, and what rights you have to that information.
These cookies are essential for our website to function and do not store any personally identifiable information. These cookies cannot be disabled.
This website uses cookies to provide you with a better user experience. A cookie is a small piece of data our website stores on a site visitor's hard drive and accesses each time you visit so we can improve your access to our site, better understand how you use our site, and serve you content that may be of interest to you. For instance, we store a cookie when you log in to our shopping cart so that we can maintain your shopping cart should you not complete checkout. These cookies do not directly store your personal information, but they do support the ability to uniquely identify your internet browser and device.
Please note: Clearing your browser cookies at any time will undo preferences saved here. The option selected here will apply only to the device you are currently using.