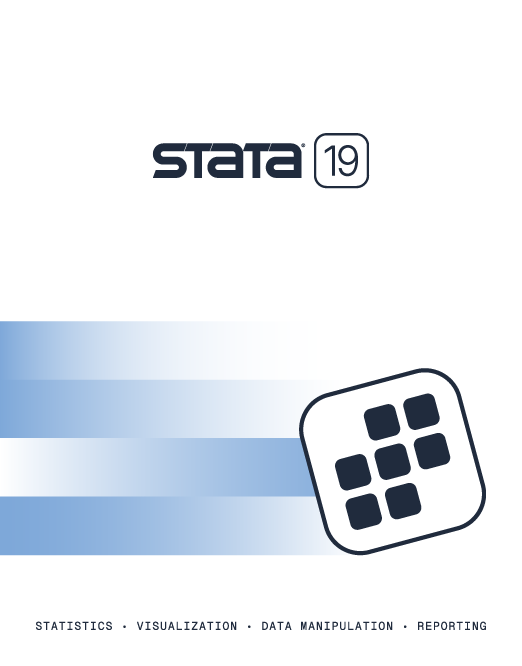
Stata 19 is here—explore all the features.
Regression Methods in Biostatistics: Linear, Logistic, Survival, and Repeated Measures Models, Second Edition |
||||||||||||||||||||||||||||||||||||
![]() Click to enlarge See the back cover |
![]()
As an Amazon Associate, StataCorp earns a small referral credit from
qualifying purchases made from affiliate links on our site.
eBook not available for this title
eBook not available for this title |
|
||||||||||||||||||||||||||||||||||
Comment from the Stata technical groupRegression Methods in Biostatistics: Linear, Logistic, Survival, and Repeated Measures Models, Second Edition is intended as a teaching text for a one-semester or two-quarter secondary statistics course in biostatistics. The book's focus is multipredictor regression models in modern medical research. The authors recommend as a prerequisite an introductory course in statistics or biostatistics, but the first three chapters provide sufficient review material to make this requirement not critical. Vittinghoff, Glidden, Shiboski, and McCulloch take a unified approach to regression models. They begin with linear regression and then discuss issues such as model statement and assumptions, types of regressors (for example, categorical versus continuous), interactions, causation and confounding, inference and testing, diagnostics, and alternative models for when assumptions are violated. Then they discuss these same issues in the contexts of other multipredictor regression models, namely, logistic regression, the Cox model, and generalized linear models (GLMs). The authors then cover generalized estimating equations (GEE) and the analysis of survey data. Almost all analyses are performed using Stata. The second edition provides two new chapters and substantially expands some of the existing chapters. Specifically, a new chapter on strengthening causal inference describes the fundamentals of causal inference and concentrates on two estimation methods—inverse probability weighting and what the authors call potential outcomes estimation. This chapter also covers propensity scores, time-dependent treatments, instrumental variables, and principal stratification. The other new chapter is on missing data. The authors describe the missing-data problem and its impact on statistical inference. They then discuss three approaches for handling missing data: maximum likelihood estimation, multiple imputation, and inverse weighting. Among the substantially revised chapters are chapters on logistic regression, now including categorical outcomes; on survival analysis, now including competing risks; on generalized linear models, now including negative binomial and zero-truncated and zero-inflated count models; and more. All the Stata examples used in the book have been updated for Stata 12. |
||||||||||||||||||||||||||||||||||||
Table of contentsView table of contents >> Preface
1. Introduction
1.1 Example: Treatment of Back Pain
1.2 The Family of Multipredictor Regression Methods 1.3 Motivation for Multipredictor Regression
1.3.1 Prediction
1.4 Guide to the Book 1.3.2 Isolating the Effect of a Single Predictor 1.3.3 Understanding Multiple Predictors 2. Exploratory and Descriptive Methods
2.1 Data Checking
2.2 Types of Data 2.3 One-Variable Descriptions
2.3.1 Numerical Variables
2.4 Two-Variable Descriptions 2.3.2 Categorical Variables
2.4.1 Outcome Versus Predictor Variables
2.5 Multivariable Descriptions 2.4.2 Continuous Outcome Variable 2.4.3 Categorical Outcome Variable 2.6 Summary 2.7 Problems 3. Basic Statistical Methods
3.1 t-Test and Analysis of Variance
3.1.1 t-Test
3.2 Correlation Coefficient 3.1.2 One- and Two-Sided Hypothesis Test 3.1.3 Paired t-Test 3.1.4 One-Way Analysis of Variance 3.1.5 Pairwise Comparisons in ANOVA 3.1.6 Multi-way ANOVA and ANCOVA 3.1.7 Robustness to Violations of Normality Assumption 3.1.8 Nonparametric Alternatives 3.1.9 Equal Variance Assumption
3.2.1 Spearman Rank Correlation Coefficient
3.3 Simple Linear Regression Model 3.2.2 Kendall's τ
3.3.1 Systematic Part of the Model
3.4 Contingency Table Methods for Binary Outcomes 3.3.2 Random Part of the Model 3.3.3 Assumptions About the Predictor 3.3.4 Ordinary Least Squares Estimation 3.3.5 Fitted Values and Residuals 3.3.6 Sums of Squares 3.3.7 Standard Errors of the Regression Coefficients 3.3.8 Hypothesis Tests and Confidence Intervals 3.3.9 Slope, Correlation Coefficient, and R2
3.4.1 Measures of Risk and Association for Binary Outcomes
3.5 Basic Methods for Survival Analysis 3.4.2 Tests of Association in Contingency Tables 3.4.3 Predictors with Multiple Categories 3.4.4 Analyses Involving Multiple Categorical Predictors 3.4.5 Collapsibility of Standard Measures of Association
3.5.1 Right Censoring
3.6 Bootstrap Confidence Intervals 3.5.2 Kaplan–Meier Estimator of the Survival Function 3.5.3 Interpretation of Kaplan–Meier Curves 3.5.4 Median Survival 3.5.5 Cumulative Event Function 3.5.6 Comparing Groups Using the Logrank Test 3.7 Interpretation of Negative Findings 3.8 Further Notes and References 3.9 Problems 3.10 Learning objectives 4. Linear Regression
4.1 Example: Exercise and Glucose
4.2 Multiple Linear Regression Model
4.2.1 Systematic Part of the Model
4.3 Categorical Predictors 4.2.2 Random Part of the Model 4.2.3 Generalization of R2 and r 4.2.4 Standardized Regression Coefficients
4.3.1 Binary Predictors
4.4 Confounding 4.3.2 Multilevel Categorical Predictors 4.3.3 The F-Test 4.3.4 Multiple Pairwise Comparisons Between Categories 4.3.5 Testing for Trend Across Categories
4.4.1 Range of Confounding Patterns
4.5 Mediation 4.4.2 Confounding Is Difficult to Rule Out 4.4.3 Adjusted Versus Unadjusted βs 4.4.4 Example: BMI and LDL
4.5.1 Indirect Effects via the Mediator
4.6 Interaction 4.5.2 Overall and Direct Effects 4.5.3 Percent Explained 4.5.4 Example: BMI, Exercise, and Glucose 4.5.5 Pitfalls in Evaluating Mediation
4.6.1 Example: Hormone Therapy and Statin Use
4.7 Checking Model Assumptions and Fit 4.6.2 Example: BMI and Statin Use 4.6.3 Interaction and Scale 4.6.4 Example: Hormone Therapy and Baseline LDL 4.6.5 Details
4.7.1 Linearity
4.8 Sample Size, Power, and Detectable Effects 4.7.2 Normality 4.7.3 Constant Variance 4.7.4 Outlying, High Leverage, and Influential Points 4.7.5 Interpretation of Results for Log Transformed Variables 4.7.6 When to Use Transformations
4.8.1 Calculations Using Standard Errors Based on Published
Data
4.9 Summary 4.10 Further Notes and References
4.10.1 Generalized Additive Models
4.11 Problems 4.12 Learning Objectives 5. Logistic Regression
5.1 Single Predictor Models
5.1.1 Interpretation of Regression Coefficients
5.2 Multipredictor Models 5.1.2 Categorical Predictors
5.2.1 Likelihood Ratio Tests
5.3 Case–Control Studies 5.2.2 Confounding 5.2.3 Mediation 5.2.4 Interaction 5.2.5 Prediction 5.2.6 Prediction Accuracy
5.3.1 Matched Case–Control Studies
5.4 Checking Model Assumptions and Fit
5.4.1 Linearity
5.5 Alternative Strategies for Binary Outcomes 5.4.2 Outlying and Influential Points 5.4.3 Model Adequacy 5.4.4 Technical Issues in Logistic Model Fitting
5.5.1 Infectious Disease Transmission Models
5.6 Likelihood 5.5.2 Pooled Logistic Regression 5.5.3 Regression Models Based on Risk Differences and Relative Risks 5.5.4 Exact Logistic Regression 5.5.5 Nonparametric Binary Regression 5.5.6 More Than Two Outcome Levels 5.7 Sample Size, Power, and Detectable Effects 5.8 Summary 5.9 Further Notes and References 5.10 Problems 5.11 Learning Objectives 6. Survival Analysis
6.1 Survival Data
6.1.1 Why Linear and Logistic Regression Would not Work
6.2 Cox Proportional Hazards Models 6.1.2 Hazard Function 6.1.3 Hazard Ratio 6.1.4 Proportional Hazards Assumption
6.2.1 Proportional Hazards Models
6.3 Extensions to the Cox Model 6.2.2 Parametric Versus Semi-Parametric Models 6.2.3 Hazard Ratios, Risk, and Survival Times 6.2.4 Hypothesis Tests and Confidence Intervals 6.2.5 Binary Predictors 6.2.6 Multilevel Categorical Predictors 6.2.7 Continuous Predictors 6.2.8 Confounding 6.2.9 Mediation 6.2.10 Interaction 6.2.11 Model Building 6.2.12 Adjusted Survival Curves for Comparing Groups 6.2.13 Predicted Survival for Specific Covariate Patterns
6.3.1 Time-Dependent Covariates
6.4 Checking Model Assumptions and Fit 6.3.2 Stratified Cox Model
6.4.1 Log-Linearity of the Hazard Function
6.5 Competing Risks Data 6.4.2 Proportional Hazards
6.5.1 What Are Competing Risks Data?
6.6 Some Details 6.5.2 Notation for Competing Risks Data 6.5.3 Summaries for Competing Risks Data
6.6.1 Bootstrap Confidence Intervals
6.7 Sample Size, Power, and Detectable Effects 6.6.2 Prediction 6.6.3 Adjusting for Nonconfounding Covariates 6.6.4 Independent Censoring 6.6.5 Interval Censoring 6.6.6 Left-Truncation 6.8 Summary 6.9 Further Notes and References 6.10 Problems 6.11 Learning Objectives 7. Repeated Measures and Longitudinal Data Analysis
7.1 A Simple Repeated Measures Example: Fecal Fat
7.1.1 Model Equations for the Fecal Fat Example
7.2 Hierarchical Data 7.1.2 Correlations Within Subjects 7.1.3 Estimates of the Effects of Pill Type
7.2.1 Example: Treatment of Back Pain
7.3 Longitudinal Data 7.2.2 Example: Physician Profiling 7.2.3 Analysis Strategies for Hierarchical Data
7.3.1 Analysis Strategies for Longitudinal Data
7.4 Generalized Estimating Equations 7.3.2 Analyzing Change Scores
7.4.1 Example: Birthweight and Birth Order Revisited
7.5 Random Effects Models 7.4.2 Correlation Structures 7.4.3 Working Correlation and Robust Standard Errors 7.4.4 Tests and Confidence Intervals 7.4.5 Use of xtgee for Clustered Logistic Regression 7.6 Re-Analysis of the Georgia Babies Data Set 7.7 Analysis of the SOF BMD Data
7.7.1 Time Varying Predictors
7.8 Marginal Versus Conditional Models 7.7.2 Separating Between- and Within-Cluster Information 7.7.3 Prediction 7.7.4 A Logistic Analysis 7.9 Example: Cardiac Injury Following Brain Hemorrhage
7.9.1 Bootstrap Analysis
7.10 Power and Sample Size for Repeated Measures Designs
7.10.1 Between-Cluster Predictor
7.11 Summary 7.10.2 Within-Cluster Predictor 7.12 Further Notes and References
7.12.1 Missing Data
7.13 Problems 7.12.2 Computing 7.14 Learning Objectives 8. Generalized Linear Models
8.1 Example: Treatment for Depression
8.1.1 Statistical Issues
8.2 Example: Costs of Phototherapy 8.1.2 Model for the Mean Response 8.1.3 Choice of Distribution 8.1.4 Interpreting the Parameters 8.1.5 Further Notes
8.2.1 Model for the Mean Response
8.3 Generalized Linear Models 8.2.2 Choice of Distribution 8.2.3 Interpreting the Parameters
8.3.1 Example: Risky Drug Use Behavior
8.4 Sample Size for the Poisson Model 8.3.2 Modeling Data with Many Zeros 8.3.3 Example: A Randomized Trial to Reduce Risk of Fracture 8.3.4 Relationship of Mean to Variance 8.3.5 Non-Linear Models 8.5 Summary 8.6 Further Notes and References 8.7 Problems 8.8 Learning Objectives 9. Strengthening Causal Inference
9.1 Potential Outcomes and Causal Effects
9.1.1 Average Causal Effects
9.2 Regression as a Basis for Causal Inference 9.1.2 Marginal Structural Model 9.1.3 Fundamental Problem of Causal Inference 9.1.4 Randomization Assumption 9.1.5 Conditional Independence 9.1.6 Marginal and Conditional Means 9.1.7 Potential Outcomes Estimation 9.1.8 Inverse Probability Weighting
9.2.1 No Unmeasured Confounders
9.3 Marginal Effects and Potential Outcomes Estimation 9.2.2 Correct Model Specification 9.2.3 Overlap and the Positivity Assumption 9.2.4 Lack of Overlap and Model Misspecification 9.2.5 Adequate Sample Size and Number of Events 9.2.6 Example: Phototherapy for Neonatal Jaundice
9.3.1 Marginal and Conditional Effects
9.4 Propensity Scores 9.3.2 Contrasting Conditional and Marginal Effects 9.3.3 When Marginal and Conditional Odds-Ratios Differ 9.3.4 Potential Outcomes Estimation 9.3.5 Marginal Effects in Longitudinal Data
9.4.1 Estimation of Propensity Scores
9.5 Time-Dependent Treatments 9.4.2 Effect Estimation Using Propensity Scores 9.4.3 Inverse Probability Weights 9.4.4 Checking for Propensity Score/Exposure Interaction 9.4.5 Addressing Positivity Violations Using Restriction 9.4.6 Average Treatment Effect in the Treated (ATT) 9.4.7 Recommendations for Using Propensity Scores
9.5.1 Models Using Time-Dependent IP Weights
9.6 Mediation 9.5.2 Implementation 9.5.3 Drawbacks and Difficulties 9.5.4 Focusing of New Users 9.5.5 Nested New-User Cohorts 9.7 Instrumental Variables
9.7.1 Vulnerabilities
9.8 Trials with Incomplete Adherence to Treatment 9.7.2 Structural Equations and Instrumental Variables 9.7.3 Checking IV Assumptions 9.7.4 Example: Effect of Hormone Therapy on Change in LDL 9.7.5 Extension to Binary Exposures and Outcomes 9.7.6 Example: Phototherapy for Neonatal Jaundice 9.7.7 Interpretation of IV Estimates
9.8.1 Intention-to-Treat
9.9 Summary 9.8.2 As-Treated Comparisons by Treatment Received 9.8.3 Instrumental Variables 9.8.4 Principal Stratification 9.10 Further Notes and References 9.11 Problems 9.12 Learning Objectives 10. Predictor Selection
10.1 Prediction
10.1.1 Bias–Variance Trade-off and Overfitting
10.2 Evaluating a Predictor of Primary Interest 10.1.2 Measures of Prediction Error 10.1.3 Optimism-Corrected Estimates of Prediction Error 10.1.4 Minimizing Prediction Error Without Overfitting 10.1.5 Point Scores 10.1.6 Example: Risk Stratification of Patients with Heart Disease
10.2.1 Including Predictors for Face Validity
10.3 Identifying Multiple Important Predictors 10.2.2 Selecting Predictors on Statistical Grounds 10.2.3 Interactions With the Predictor of Primary Interest 10.2.4 Example: Incontinence as a Risk Factor for Falling 10.2.5 Directed Acyclic Graphs 10.2.6 Randomized Experiments
10.3.1 Ruling Out Confounding Is Still Central
10.4 Some Details 10.3.2 Cautious Interpretation Is Also Key 10.3.3 Example: Risk Factors for Coronary Heart Disease 10.3.4 Allen–Cady Modified Backward Selection
10.4.1 Collinearity
10.5 Summary 10.4.2 Number of Predictors 10.4.3 Alternatives to Backward Selection 10.4.4 Model Selection and Checking 10.4.5 Model Selection Complicates Inference 10.6 Further Notes and References 10.7 Problems 10.8 Learning Objectives 11. Missing Data
11.1 Why Missing Data Can Be a Problem
11.1.1 Missing Predictor in Linear Regression
11.2 Classifications of Missing Data 11.1.2 Missing Outcome in Longitudinal Data
11.2.1 Mechanisms for Missing Data
11.3 Simple Approaches to Handling Missing Data
11.3.1 Include a Missing Data Category
11.4 Methods for Handling Missing Data 11.3.2 Last Observation or Baseline Carried Forward 11.5 Missing Data in the Predictors and Multiple Imputation
11.5.1 Remarks About Using Multiple Imputation
11.6 Deciding Which Missing Data Mechanism May Be Applicable 11.5.2 Approaches to Multiple Imputation 11.5.3 Multiple Imputation for HERS 11.7 Missing Outcomes, Missing Completely at Random 11.8 Missing Outcomes, Covariate-Dependent Missing Completely at Random 11.9 Missing Outcomes for Longitudinal Studies, Missing at Random
11.9.1 ML and MAR
11.10 Technical Details About Maximum Likelihood and Data Which Are
Missing at Random 11.9.2 Multiple Imputation 11.9.3 Inverse Probability Weighting
11.10.1 An Example of the EM Algorithm
11.11 Methods for Data that Are Missing Not at Random 10.10.2 The EM Algorithm Imputes the Missing Data 10.10.3 ML Versus MI with Missing Outcomes
11.11.1 Pattern Mixture Models
11.12 Summary 11.11.2 Multiple Imputation Under MNAR 11.11.3 Joint Modeling of Outcomes and the Dropout Process 11.13 Further Notes and References 11.14 Problems 11.15 Learning Objectives 12. Complex Surveys
12.1 Overview of Complex Survey Designs
12.2 Inverse Probability Weighting
12.2.1 Accounting for Inverse Probability Weights in the
Analysis
12.3 Clustering and Stratification 12.2.2 Inverse Probability Weights and Missing Data
12.3.1 Design Effects
12.4 Example: Diabetes in NHANES 12.5 Some Details
12.5.1 Ignoring Secondary Levels of Clustering
12.6 Summary 12.5.2 Other Methods of Variance Estimation 12.5.3 Model Checking 12.5.4 Postestimation Capabilities in Stata 12.5.5 Other Statistical Packages for Complex Surveys 12.7 Further Notes and References 12.8 Problems 12.9 Learning Objectives 13. Summary
13.1 Introduction
13.2 Selecting Appropriate Statistical Methods 13.3 Planning and Executing a Data Analysis
13.3.1 Analysis Plans
13.4 Further Notes and References 13.3.2 Choice of Software 13.3.3 Data Preparation 13.3.4 Record Keeping and Reproducibility of Results 13.3.5 Data Security 13.3.6 Consulting a Statistician 13.3.7 Use of Internet Resources
13.4.1 Multiple Hypothesis Tests
13.4.2 Statistical Learning References
Index
|
Learn
Free webinars
NetCourses
Classroom and web training
Organizational training
Video tutorials
Third-party courses
Web resources
Teaching with Stata
© Copyright 1996–2025 StataCorp LLC. All rights reserved.
×
We use cookies to ensure that we give you the best experience on our website—to enhance site navigation, to analyze usage, and to assist in our marketing efforts. By continuing to use our site, you consent to the storing of cookies on your device and agree to delivery of content, including web fonts and JavaScript, from third party web services.
Cookie Settings
Last updated: 16 November 2022
StataCorp LLC (StataCorp) strives to provide our users with exceptional products and services. To do so, we must collect personal information from you. This information is necessary to conduct business with our existing and potential customers. We collect and use this information only where we may legally do so. This policy explains what personal information we collect, how we use it, and what rights you have to that information.
These cookies are essential for our website to function and do not store any personally identifiable information. These cookies cannot be disabled.
This website uses cookies to provide you with a better user experience. A cookie is a small piece of data our website stores on a site visitor's hard drive and accesses each time you visit so we can improve your access to our site, better understand how you use our site, and serve you content that may be of interest to you. For instance, we store a cookie when you log in to our shopping cart so that we can maintain your shopping cart should you not complete checkout. These cookies do not directly store your personal information, but they do support the ability to uniquely identify your internet browser and device.
Please note: Clearing your browser cookies at any time will undo preferences saved here. The option selected here will apply only to the device you are currently using.