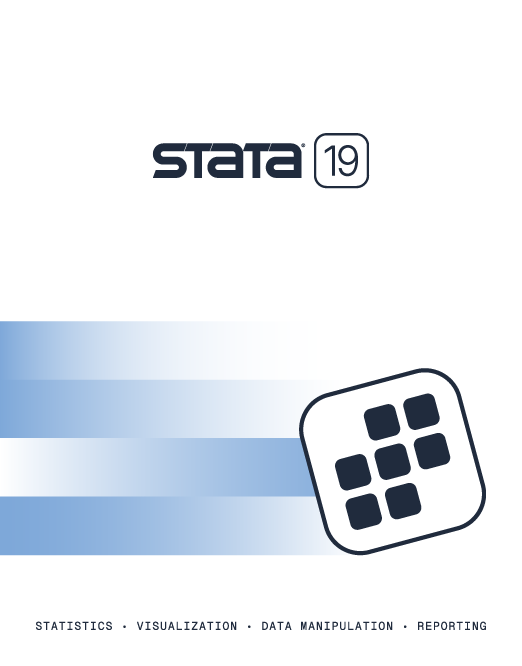
Stata 19 is here—explore all the features.
A Primer for Spatial Econometrics, Second Edition |
||||||||||||||||||||||||||||||||
![]() Click to enlarge See the back cover |
![]()
As an Amazon Associate, StataCorp earns a small referral credit from
qualifying purchases made from affiliate links on our site.
eBook not available for this title
eBook not available for this title |
|
||||||||||||||||||||||||||||||
Comment from the Stata technical groupGiuseppe Arbia's book, A Primer for Spatial Econometrics, is written for anyone wanting to learn and apply spatial econometrics in empirical research. The book is suitable for beginners because only undergraduate econometrics training is assumed. Those with more experience will also find it beneficial because it outlines the recent developments in spatial econometrics research. The book is divided into five chapters. The first three chapters introduce readers to the foundation of spatial econometrics: classical linear regression, the spatial weighting matrix, and spatial linear autoregressive models. The fourth chapter outlines recent developments in spatial econometrics research, such as heterogeneity, spatial models for binary variables, the spatial panel-data model, and the endogenous spatial weighting matrix. Finally, the fifth chapter introduces alternative spatial model specifications for big datasets. A good feature of this book is that there are sections dedicated to computer codes in Stata, R, and Python for each different spatial econometrics model. This allows readers to get practical experience to deepen their understanding of spatial econometrics theory. In summary, A Primer for Spatial Econometrics, is a great introduction for researchers and students wanting to learn spatial econometrics from theoretical, empirical, and computational points of view. |
||||||||||||||||||||||||||||||||
Table of contentsView table of contents >> I The Classical Linear Regression Model
1.1 The Basic Linear Regression Model
1.2 Non-sphericity of the Disturbances 1.3 Endogeneity 1.4 Computer Codes
1.4.1 Running a Regression with R
References 1.4.2 Running a Regression with Stata 1.4.3 Running a Regression with Python 2 Some Important Spatial Definitions
2.1 The Spatial Weight Matrix W and the Definition of Spatial Lag
2.2 Testing Sptial Autocorrelation Among OLS Residuals Without an Explicit Alternative Hypothesis 2.3 Computer Codes: R
2.3.1 Creating and Managing W Matrices
2.4 Computer Codes: Stata 2.3.2 Calculating Moran's I Spatial Correlation 2.3.3 Some Useful Spatial R Databases
2.4.1 Creating and Managing W Matrices
2.5 Computer Codes: Python 2.4.2 Calculating Moran's I Spatial Correlation 2.4.3 Some Useful Databases to Be Used in Stata
2.5.1 Creating and Managing W Matrices
References 2.5.2 Calculating Moran's I Spatial Correlation 2.5.3 Some Useful PySAL Databases 3 Spatial Linear Regression Models
3.1 Generalities
3.2 Pure Spatial Autoregression 3.3 The Spatial Lag of X Model (SLX) 3.4 The Spatial Error Model (SEM)
3.4.1 Introduction
3.5 The Spatial Lag Model (SLM) 3.4.2 Maximum Likelihood Estimator 3.4.3 Feasible GLS
3.5.1 Generalities
3.6 The Spatial Durbin Model 3.5.2 Maximum Likelihood Estimator 3.5.3 Two Stages Least Squares Estimators 3.7 The General SARAR(1,1) Model
3.7.1 Generalities
3.8 Testing Spatial Autocorrelation Among the Residuals with an Explicit Alternative Hypothesis 3.7.2 Maximum Likelihood Estimator 3.7.3 The Generalized Spatial Two Stages Least Squares (GS2SLS) 3.7.4 The Lee Fully Efficient Estimators
3.8.1 Testing Spatial Autocorrelation Among the Residuals Using SEM or SLM as Alternatives
3.9 Interpretation of the Parameters in Spatial Econometric Models 3.8.2 Testing Spatial Autocorrelation Among the Residuals Using a Spatial Model as an Alternative: The Modified Moran's I Test 3.11 Estimating Linear Spatial Models with Stata 3.12 Estimating Linear Spatial Models with Python References to the Chapter 4 Further Topics in Spatial Econometrics
4.1 Heteroscedastic Innovations
4.1.1 Generalities
4.2 Spatial Models for Binary Response Variables
4.1.2 The SARAR Model with Heteroscedastic Disturbances 4.1.3 Spatial HAC Estimators
4.2.1 Introduction
4.3 Spatial Panel Data Models (Written by Giovanni Millo)4.2.2 The A-Spatial Logit and Probit Models 4.2.3 The Spatial Logit and Probit Models 4.2.4 The Spatial Tobit Model 4.2.5 Further Spatial Discrete Choice Models
4.3.1 Generalities
4.4 Non-stationary Spatial Econometric Models 4.3.2 Unobserved Heterogeneity and Individual Effects 4.3.3 Spatial Panel Models with Random Effects 4.3.4 Spatial Panel Models with Fixed Effects 4.3.5 Estimation 4.3.6 Further Modeling Frameworks in Spatial Panel Data Modeling
4.4.1 Generalities
4.5 Bayesian Spatial Models 4.4.2 Geographically Weighted Regression 4.4.3 Further Developments 4.6 Stochastic and Endogenous Weighting Matrices
4.6.1 Stochastic Weight Matrices
4.7 Computer Codes 4.6.2 Endogenous Weight Matrices
4.7.1 Estimating Heteroscedastic Linear Spatial Models with R
References to the Chapter 4.7.2 Estimating Heteroscedastic Linear Spatial Models with Stata 4.7.3 Estimating Heteroscedastic Linear Spatial Models with Python 4.7.4 Estimating Spatial Probit/Logit/Tobit Models with R 4.7.5 Estimating Spatial Probit/Logit/Tobit Models with Stata 4.7.6 Estimating Spatial Panel Models with R 4.7.7 Estimating Spatial Panel Models with Stata 4.7.8 Estimating Spatial Panel Models with Python 4.7.9 Estimating Geographically Weighted Regression Models with R 4.7.10 Estimating Geographically Weighted Regression Models with Stata 4.7.11 Estimating Geographically Weighted Regression Models with Python 4.7.12 Estimating Bayesian Spatial Econometric Models with R 5 Alternative Model Specifications for Big Datasets
5.1 Introduction: Spatial Econometrics and the Big Data Revolution
5.2 The MESS Specification
5.2.1 A MESS Spatial Lag Specification
5.3 The Unilateral Approximation Approach 5.2.2 A MESS Spatial Error Specification and Further Extensions
5.3.1 The Importance of Asymmetries and Anisotropies in Spatial Econometrics
5.4 A Composite Likelihood Approach 5.3.2 Testing Isotropy in Spatial Lag Models 5.3.3 Inference for a Unilateral Spatial Lag Model
5.4.1 Generalities
5.5 Handling the Second "V" of Big Data (Velocity) in Spatial Regressions5.4.2 A Bivariate Marginal Likelihood Approach to Spatial Error Model Estimation 5.6 R Codes References 6 Conclusions: What's next?
References
Index
|
Learn
Free webinars
NetCourses
Classroom and web training
Organizational training
Video tutorials
Third-party courses
Web resources
Teaching with Stata
© Copyright 1996–2025 StataCorp LLC. All rights reserved.
×
We use cookies to ensure that we give you the best experience on our website—to enhance site navigation, to analyze usage, and to assist in our marketing efforts. By continuing to use our site, you consent to the storing of cookies on your device and agree to delivery of content, including web fonts and JavaScript, from third party web services.
Cookie Settings
Last updated: 16 November 2022
StataCorp LLC (StataCorp) strives to provide our users with exceptional products and services. To do so, we must collect personal information from you. This information is necessary to conduct business with our existing and potential customers. We collect and use this information only where we may legally do so. This policy explains what personal information we collect, how we use it, and what rights you have to that information.
These cookies are essential for our website to function and do not store any personally identifiable information. These cookies cannot be disabled.
This website uses cookies to provide you with a better user experience. A cookie is a small piece of data our website stores on a site visitor's hard drive and accesses each time you visit so we can improve your access to our site, better understand how you use our site, and serve you content that may be of interest to you. For instance, we store a cookie when you log in to our shopping cart so that we can maintain your shopping cart should you not complete checkout. These cookies do not directly store your personal information, but they do support the ability to uniquely identify your internet browser and device.
Please note: Clearing your browser cookies at any time will undo preferences saved here. The option selected here will apply only to the device you are currently using.