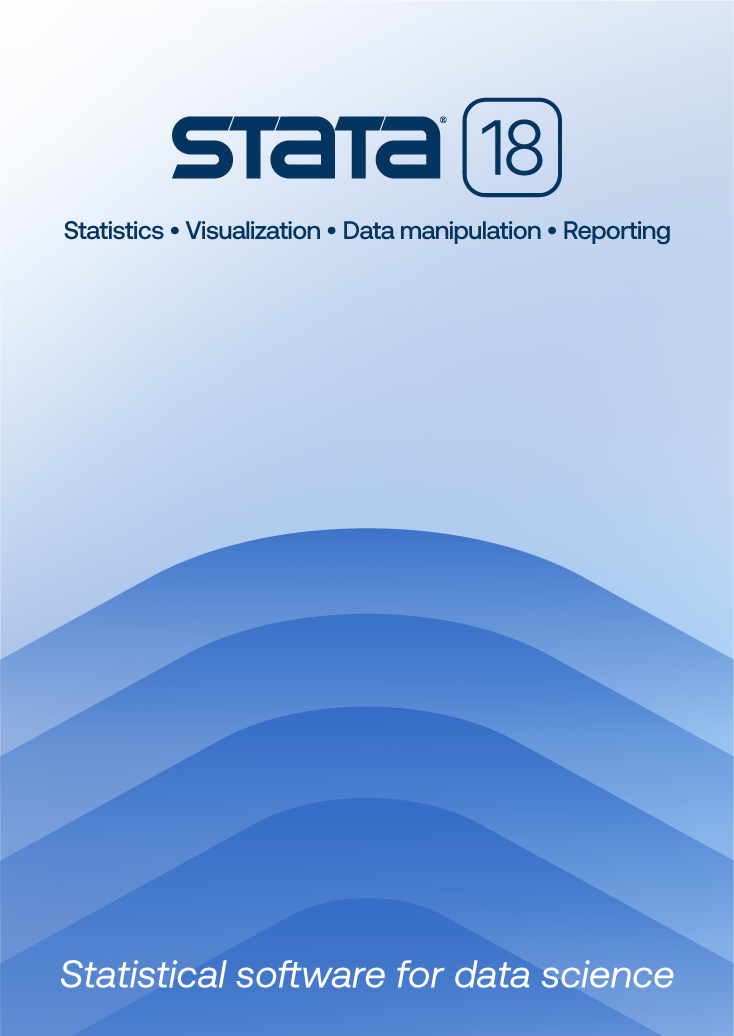
Multivariate Analysis, Second Edition |
||||||||||||||||||||||||||||||||||||
![]() Click to enlarge See the back cover |
![]()
As an Amazon Associate, StataCorp earns a small referral credit from
qualifying purchases made from affiliate links on our site.
$89.68 VitalSource eBook Add to carteBook not available for this title |
|
||||||||||||||||||||||||||||||||||
Comment from the Stata technical groupThe first edition of Multivariate Analysis, by Mardia, Kent, and Bibby, has long been an essential resource for students seeking a mathematical treatment of multivariate statistical analysis. The second edition, by Mardia, Kent, and Taylor, offers new chapters on modern multivariate methods such as graphical models and approaches to high-dimensional data, as well as considerably updated chapters on supervised and unsupervised learning. The book begins by introducing the basic concepts of random vectors and matrices, distributions, estimation, and hypothesis testing, while the second half dives deep into theory and methods for multivariate regression, multivariate analysis of variance, principal component analysis, factor analysis, and much more. Additionally, each chapter ends with exercises so that readers can practice what they have learned. Newly updated, Multivariate Analysis continues to be a trusted resource both for students learning this material for the first time and for researchers seeking deeper knowledge of multivariate statistical theory. |
||||||||||||||||||||||||||||||||||||
Table of contentsView table of contents >> Epigraph xvii
Preface to the Second Edition xix
Preface to the First Edition xxi
Acknowledgments from First Edition xxv
Notation, Abbreviations, and Key Ideas xxvii
1 Introduction
1.1 Objects and Variables
1.2 Some Multivariate Problems and Techniques
1.2.1 Generalizations of Univariate Techniques
1.3 The Data Matrix 1.2.2 Dependence and Regression 1.2.3 Linear Combinations 1.2.4 Assignment and Dissection 1.2.5 Building Configurations 1.4 Summary Statistics
1.4.1 The Mean Vector and Covariance Matrix
1.5 Linear Combinations 1.4.2 Measures of Multivariate Scatter
1.5.1 The Scaling Transformation
1.6 Geometrical Ideas 1.5.2 Mahalanobis Transformation 1.5.3 Principal Component Transformation 1.7 Graphical Representation
1.7.1 Univariate Scatters
1.8 Measures of Multivariate Skewness and Kurtosis 1.7.2 Bivariate Scatters 1.7.3 Harmonic Curves 1.7.4 Parallel Coordinates Plot Exercises and Complements 2 Basic Properties of Random Vectors
2.1 Cumulative Distribution Functions and Probability Density Functions
2.2 Population Moments
2.2.1 Expectation and Correlation
2.3 Characteristic Functions 2.2.2 Population Mean Vector and Covariance Matrix 2.2.3 Mahalanobis Space 2.2.4 Higher Moments 2.2.5 Conditional Moments 2.4 Transformations 2.5 The Multivariate Normal Distribution
2.5.1 Definition
2.6 Random Samples 2.5.2 Geometry 2.5.3 Properties 2.5.4 Singular Multivariate Normal Distribution 2.5.5 The Matrix Normal Distribution 2.7 Limit Theorems Exercises and Complements 3 Nonnormal Distributions
3.1 Introduction
3.2 Some Multivariate Generalizations of Univariate Distributions
3.2.1 Direct Generalization
3.3 Families of Distributions 3.2.2 Common Components 3.2.3 Stochastic Generalizations
3.3.1 The Exponential Family
3.4 Insights into Skewness and Kurtosis 3.3.2 The Spherical Family 3.3.3 Elliptical Distributions 3.3.4 Stable Distributions 3.5 Copulas
3.5.1 The Gaussian Copula
Exercises and Complements 3.5.2 The Clayton–Mardia Copula 3.5.3 Archimedean Copulas 3.5.4 Fréchet-Höffding Bounds 4 Normal Distribution Theory
4.1 Introduction and Characterization
4.1.1 Introduction
4.2 Linear Forms 4.1.2 A Defintion by Characterization 4.3 Transformations of Normal Data Matrices 4.4 The Wishart Distribution
4.4.1 Introduction
4.5 The Hotelling T² Distribution 4.4.2 Properties of Wishart Matrices 4.4.3 Partitioned Wishart Matrices 4.6 Mahalanobis Distance
4.6.1 The Two-Sample Hotelling T² Statistic
4.7 Statistics Based on the Wishart Distribution 4.6.2 A Decomposition of Mahalanobis Distance 4.8 Other Distributions Related to the Multivariate Normal Exercises and Complements 5 Estimation
5.1 Likelihood and Sufficiency
5.1.1 The Likelihood Function
5.2 Maximum-likelihood Estimation 5.1.2 Efficient Scores and Fisher's Information 5.1.3 The Cramér–Rao Lower Bound 5.1.4 Sufficiency
5.2.1 General Case
5.3 Robust Estimation of Location and Dispersion for Multivariate Distributions 5.2.2 Multivariate Normal Case 5.2.3 Matrix Normal Distribution
5.3.1 M-Estimates of Location
5.4 Bayesian Inference 5.3.2 Minimum Covariance Determinant 5.3.3 Multivariate Trimming 5.3.4 Stahel–Donoho Estimator 5.3.5 Minimum Volume Estimator 5.3.6 Tyler's Estimate of Scatter Exercises and Complements 6 Hypothesis Testing
6.1 Introduction
6.2 The Techniques Introduced
6.2.1 The Likelihood Ratio Test (LRT)
6.3 The Techniques Further Illustrated 6.2.2 The Union Interesection Test (UIT)
6.3.1 One-sample hypotheses on µ
6.4 Simultaneous Confidence Intervals 6.3.2 One-sample hypotheses on ∑ 6.3.3 Multisample Hypotheses
6.4.1 The one-sample Hotelling T² case
6.5 The Behrens–Fisher Problem 6.4.2 The two-sample Hotelling T² case 6.4.3 Other examples 6.6 Multivariate Hypothesis Testing: Some General Points 6.7 Nonnormal Data 6.8 Mardia’s Nonparametric Test for the Bivariate Two-sample Problem Exercises and Complements 7 Multivariate Regression Analysis
7.1 Introduction
7.2 Maximum-likelihood Estimation
7.2.1 Maximum-likelihood Estimators for B and ∑
7.3 The General Linear Hypothesis 7.2.2 The distribution of B and ∑
7.3.1 The Likelihood Ratio Test (LRT)
7.4 Design Matrices of Degenerate Rank 7.3.2 The Union Intersection Test (UIT) 7.3.3 Simultaneous Confidence Intervals 7.5 Multiple Correlation
7.5.1 The Effect of the Mean
7.6 Least-squares Estimation 7.5.2 Multiple Correlation Coefficient 7.5.3 Partial Correlation Coefficient 7.5.4 Measures of Correlation Between Vectors
7.6.1 Ordinary Least-squares (OLS) Estimation
7.7 Discarding of Variables 7.6.2 Generalized Least Squares 7.6.3 Application to Multivariate Regression 7.6.4 Asymptotic Consistency of Least-squares Estimators
7.7.1 Dependence Analysis
Exercises and Complements 7.7.2 Interdependance Analysis 8 Graphical Models
8.1 Introduction
8.2 Graphs and Conditional Independence 8.3 Gaussian Graphical Models
8.3.1 Estimation
8.4 Log-linear Graphical Models 8.3.2 Model Selection
8.4.1 Notation
8.5 Directed and Mixed Graphs 8.4.2 Log-linear Models 8.4.3 Log-linear Models with a Graphical Interpretation Exercises and Complements 9 Principal Component Analysis
9.1 Introduction
9.2 Definition and Properties of Principal Components
9.2.1 Population Principal Components
9.3 Sampling Properties of Principal Components 9.2.2 Sample Principal Components 9.2.3 Further Properties of Principal Components 9.2.4 Correlation Structure 9.2.5 The Effect of Ignoring Some Components 9.2.6 Graphical Representation of Principal Components 9.2.7 Biplots
9.3.1 Maximum-likelihood Estimation for Normal Data
9.4 Testing Hypotheses About Principal Components 9.3.2 Asymptotic Distributions for Normal Data
9.4.1 Introduction
9.5 Correspondence Analysis 9.4.2 The Hypothesis that (γ1 + ⋯ γk/γ1 + ⋯ γp) = ψ 9.4.3 The Hypothesis that (p – k) Eigenvalues of ∑ are Equal 9.4.4 Hypotheses Concerning Correlation Matrices
9.5.1 Contingency Tables
9.6 Allometry – Measurement of Size and Shape 9.5.2 Gradient Analysis 9.7 Discarding of Variables 9.8 Principal Component Regression 9.9 Projection Pursuit and Independent Component Analysis
9.9.1 Projection Pursuit
9.10 PCA in High Dimensions 9.9.2 Independent Component Analysis Exercises and Complements 10 Factor Analysis
10.1 Introduction
10.2 The Factor Model
10.2.1 Definition
10.3 Principal Factor Analysis 10.2.2 Scale Invariance 10.2.3 Nonuniqueness of Factor Loadings 10.2.4 Estimation of the Parameters in Factor Analysis 10.2.5 Use of the Correlation Matrix R in Estimation 10.4 Maximum-likelihood Factor Analysis 10.5 Goodness-of-fit Test 10.6 Rotation of Factors
10.6.1 Interpretation of Factors
10.7 Factor Scores 10.6.2 Varimax Rotation 10.8 Relationships Between Factor Analysis and Principal Component Analysis 10.9 Analysis of Covariance Structures Exercises and Complements 11 Canonical Correlation Analysis
11.1 Introduction
11.2 Mathematical Development
11.2.1 Population Canonical Correlation Analysis
11.3 Qualitative Data and Dummy Variables 11.2.2 Sample Canonical Correlation Analysis 11.2.3 Sample Properties and Tests 11.2.4 Scoring and Prediction 11.4 Qualitative and Quantitative Data Exercises and Complements 12 Discriminant Analysis and Statistical Learning
12.1 Introduction
12.2 Bayes’ Discriminant Rule 12.3 The Error Rate
12.3.1 Probabilities of Misclassification
12.4 Discrimination Using the Normal Distribution 12.3.2 Estimation of Error Rate 12.3.3 Confusion Matrix
12.4.1 Population Discriminant Rules
12.5 Discarding of Variables 12.4.2 The Sample Discriminant Rules 12.4.3 Is Discrimination Worthwhile? 12.6 Fisher’s Linear Discriminant Function 12.7 Nonparametric Distance-based Methods
12.7.1 Nearest-neighbor Classifier
12.8 Classification Trees 12.7.2 Large Sample Behavior of the Nearest-neighbor Classifier 12.7.3 Kernel Classifiers
12.8.1 Splitting Criteria
12.9 Logistic Discrimination 12.8.2 Pruning
12.9.1 Logistic Regression Model
12.10 Neural Networks 12.9.2 Estimation and Inference 12.9.3 Interpretation of the Parameter Estimates 12.9.4 Extensions
12.10.1 Motivation
Exercises and Complements 12.10.2 Multilayer Perceptron 12.10.3 Radial Basis Functions 12.10.4 Support Vector Machines 13 Multivariate Analysis of Variance
13.1 Introduction
13.2 Formulation of Multivariate One-way Classification 13.3 The Likelihood Ratio Principle 13.4 Testing Fixed Contrasts 13.5 Canonical Variables and A Test of Dimensionality
13.5.1 The Problem
13.6 The Union Intersection Approach 13.5.2 The LR Test (∑ Known) 13.5.3 Asymptotic Distribution of the Likelihood Ratio Criterion 13.5.4 The Estimated Plane 13.5.5 The LR Test (Unknown ∑) 13.5.6 The Estimated Plane (Unknown ∑) 13.5.7 Profile Analysis 13.7 Two-way Classification
13.7.1 Tests for Interactions
Exercises and Complements 13.7.2 Tests for Main Effects 13.7.3 Simultaneous Confidence Regions 13.7.4 Extension to Higher Designs 14 Cluster Analysis and Unsupervised Learning
14.1 Introduction
14.2 Probabilistic Membership Models 14.3 Parametric Mixture Models 14.4 Partitioning Methods 14.5 Hierarchical Methods
14.5.1 Agglomerative Algorithms
14.6 Distances and Similarities 14.5.2 Minimum Spanning Tree and Single Linkage 14.5.3 Properties of Different Agglomerative Algorithms
14.6.1 Distances
14.7 Grouped Data 14.6.2 Similarity Coefficients 14.8 Mode Seeking 14.9 Measures of Agreement Exercises and Complements 15 Multidimensional Scaling
15.1 Introduction
15.2 Classical Solution
15.2.1 Some Theoretical Results
15.3 Duality Between Principal Coordinate Analysis and Principal Component Analysis 15.2.2 An Algorithm for the Classical MDS Solution 15.2.3 Similarities 15.4 Optimal Properties of the Classical Solution and Goodness of Fit 15.5 Seriation
15.5.1 Description
15.6 Nonmetric Methods 15.5.2 Horseshoe Effect 15.7 Goodness of Fit Measure: Procrustes Rotation 15.8 Multisample Problem and Canonical Variates Exercises and Complements 16 High-dimensional Data
16.1 Introduction
16.2 Shrinkage Methods in Regression
16.2.1 The Multiple Linear Regression Model
16.3 Principal Component Regression 16.2.2 Ridge Regression 16.2.3 Least Absolute Selection and Shrinkage Operator (LASSO) 16.4 Partial Least Squares Regression
16.4.1 Overview
16.5 Functional Data 16.4.2 The PLS1 Algorithm to Construct the PLS Loading Matrix for p = 1 Response Variable 16.4.3 The PLS2 Algorithm to Construct the PLS Loading Matric for p > 1 Response Variables 16.4.4 The Predictor Envelope Model 16.4.5 PLS Regression 16.4.6 Joint Envelope Models
16.5.1 Functional Principal Component Analysis
Exercises and Complements 16.5.2 Functional Linear Regression Models A Matrix Algebra
A.1 Introduction A.2 Matrix Operations A.2.1 Transpose A.2.2 Trace A.2.3 Determinants and Cofactors A.2.4 Inverse A.2.5 Kronecker Products A.3.1 Orthogonal Matrices A.3.2 Equicorrelation Matrix A.3.3 Centering Matrix A.4 Vector Spaces, Rank, and Linear Equations A.4.1 Vector Spaces A.4.2 Rank A.4.3 Linear Equations A.5 Linear Transformations A.6 Eigenvalues and Eigenvectors A.6.1 General Results A.6.2 Symmetric Matrices A.7 Quadratic Forms and Definiteness A.8 Generalized Inverse A.9 Matrix Differentiation and Maximization Problems A.10 Geometrical Ideas A.10.1 n-dimensional Geometry A.10.2 Orthogonal Transformation A.10.3 Projections A.10.4 Ellipsoids B Univariate Statistics
B.1 Introduction
B.2 Normal Distribution B.3 Chi-squared Distribution B.4 F and Beta Variables B.5 t Distribution B.6 Poisson Distribution C R commands and Data
C.1 Basic R Commands Related to Matrices
C.2 R Libraries and Commands Used in Exercises and Figures C.3 Data Availability D Tables
References and Author Index
Index
|
Learn
Free webinars
NetCourses
Classroom and web training
Organizational training
Video tutorials
Third-party courses
Web resources
Teaching with Stata
© Copyright 1996–2024 StataCorp LLC. All rights reserved.
×
We use cookies to ensure that we give you the best experience on our website—to enhance site navigation, to analyze usage, and to assist in our marketing efforts. By continuing to use our site, you consent to the storing of cookies on your device and agree to delivery of content, including web fonts and JavaScript, from third party web services.
Cookie Settings
Last updated: 16 November 2022
StataCorp LLC (StataCorp) strives to provide our users with exceptional products and services. To do so, we must collect personal information from you. This information is necessary to conduct business with our existing and potential customers. We collect and use this information only where we may legally do so. This policy explains what personal information we collect, how we use it, and what rights you have to that information.
These cookies are essential for our website to function and do not store any personally identifiable information. These cookies cannot be disabled.
This website uses cookies to provide you with a better user experience. A cookie is a small piece of data our website stores on a site visitor's hard drive and accesses each time you visit so we can improve your access to our site, better understand how you use our site, and serve you content that may be of interest to you. For instance, we store a cookie when you log in to our shopping cart so that we can maintain your shopping cart should you not complete checkout. These cookies do not directly store your personal information, but they do support the ability to uniquely identify your internet browser and device.
Please note: Clearing your browser cookies at any time will undo preferences saved here. The option selected here will apply only to the device you are currently using.