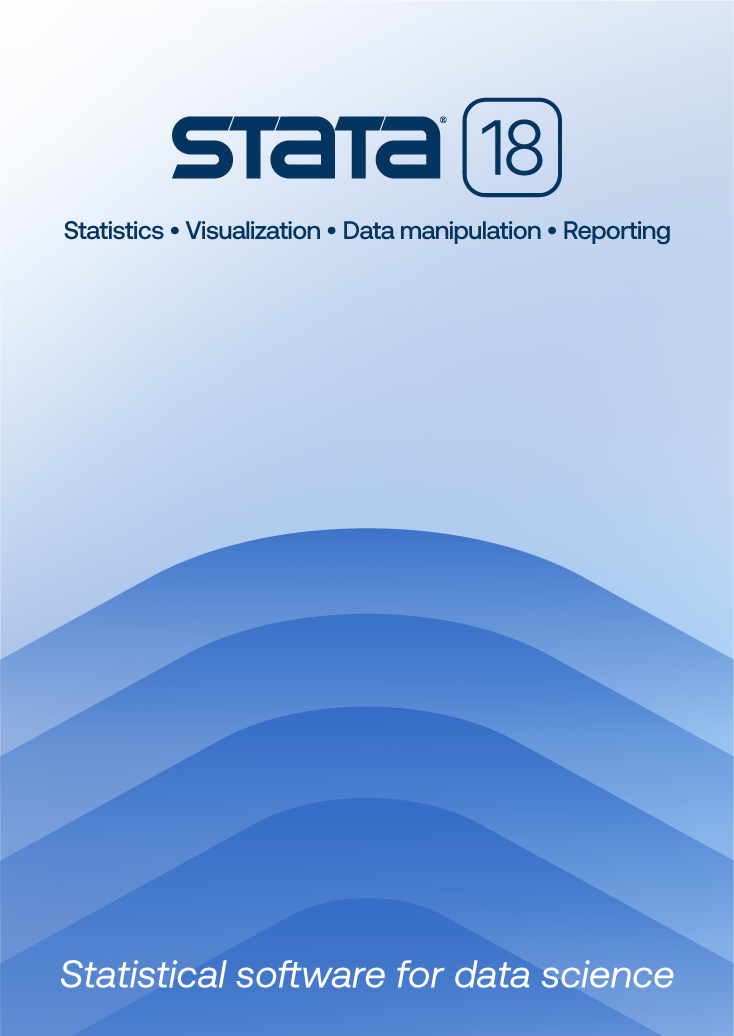
Multiple Imputation and Its Application |
||||||||||||||||||||||||||||||||||||
![]() Click to enlarge See the back cover |
![]()
As an Amazon Associate, StataCorp earns a small referral credit from
qualifying purchases made from affiliate links on our site.
eBook not available for this title
eBook not available for this title |
|
||||||||||||||||||||||||||||||||||
Comment from the Stata technical groupMultiple Imputation and its Application, by James R. Carpenter and Michael G. Kenward, provides an excellent review of multiple imputation (MI) from basic to advanced concepts. MI is a statistical method for analyzing incomplete data. The flexibility of the MI procedure has prompted its use in a wide variety of applications. This book describes the rationale for MI and its underlying assumptions in a broad range of statistical settings, and demonstrates the use of this procedure for handling missing data in complex data structures. The text provides a good mixture of theory and practice. Throughout the book, the concepts are illustrated with real data examples. The book is divided into three parts: foundations, MI for cross-sectional data, and advanced topics. The first part reviews the basic concepts of missing data, such as types of missing data and missing-data assumptions, and of multiple imputation, such as the MI procedure and its justification. The second part describes the use of MI for handling missing values in cross-sectional data, including the imputation of different types of data (continuous, binary, ordinal, etc.), and for handling nonlinearities and interactions during imputation. The third part discusses the advanced use of MI for dealing with missing data in complex data structures such as survival data and multilevel data. Other important advanced topics are covered, including the handling of survey weights during imputation, sensitivity analysis, and robust MI. |
||||||||||||||||||||||||||||||||||||
Table of contentsView table of contents >> Preface
Data acknowledgements
Acknowledgements
Glossary
PART 1 FOUNDATIONS
1 Introduction
1.1 Reasons for missing data
1.2 Examples 1.3 Patterns of missing data
1.3.1 Consequences of missing data
1.4 Inferential framework and notation
1.4.1 Missing Completely At Random (MCAR)
1.5 Using observed data to inform assumptions about the missingness
mechanism 1.4.2 Missing At Random (MAR) 1.4.3 Missing Not At Random (MNAR) 1.4.4 Ignorability 1.6 Implications of missing data mechanisms for regression analyses
1.6.1 Partially observed response
1.7 Summary 1.6.2 Missing covariates 1.6.3 Missing covariates and response 1.6.4 Subtle issues I: The odds ratio 1.6.5 Implication for linear regression 1.6.6 Subtle issues II: Subsample ignorability 1.6.7 Summary: When restricting to complete records is valid 2 The multiple imputation procedure and its justification
2.1 Introduction
2.2 Intuitive outline of the MI procedure 2.3 The generic MI procedure 2.4 Bayesian justification of MI 2.5 Frequentist inference
2.5.1 Large number of imputations
2.6 Choosing the number of imputations 2.5.2 Small number of imputations 2.7 Some simple examples 2.8 MI in more general settings
2.8.1 Survey sample settings
2.9 Constructing congenial imputation models 2.10 Practical considerations for choosing imputation models 2.11 Discussion PART II MULTIPLE IMPUTATION FOR CROSS SECTIONAL DATA
3 Multiple imputation of quantitative data
3.1 Regression imputation with a monotone missingness pattern
3.1.1 MAR mechanisms consistent with a monotone pattern
3.2 Joint modelling 3.1.2 Justification
3.2.1 Fitting the imputation model
3.3 Full conditional specification
3.3.1 Justification
3.4 Full conditional specification versus joint modelling 3.5 Software for multivariate normal imputation 3.6 Discussion 4 Multiple imputation of binary and ordinal data
4.1 Sequential imputation with monotone missingness pattern
4.2 Joint modelling with the multivariate normal distribution 4.3 Modelling binary data using latent normal variables
4.3.1 Latent normal model for ordinal data
4.4 General location model 4.5 Full conditional specification
4.5.1 Justification
4.6 Issues with over-fitting 4.7 Pros and cons of the various approaches 4.8 Software 4.9 Discussion 5 Multiple imputation of unordered categorical data
5.1 Monotone missing data
5.2 Multivariate normal imputation for categorical data 5.3 Maximum indicant model
5.3.1 Continuous and categorical variable
5.4 General location model 5.3.2 Imputing missing data 5.3.3 More than one categorical variable 5.5 FCS with categorical data 5.6 Perfect prediction issues with categorical data 5.7 Software 5.8 Discussion 6 Nonlinear relationships
6.1 Passive imputation
6.2 No missing data in nonlinear relationships 6.3 Missing data in nonlinear relationships
6.3.1 Predictive Mean Matching (PMM)
6.4 Discussion 6.3.2 Just Another Variable (JAV) 6.3.3 Joint modelling approach 6.3.4 Extension to more general models and missing data patterns 6.3.5 Metropolis-Hastings sampling 6.3.6 Rejection sampling 6.3.7 FCS approach 7 Interactions
7.1 Interaction variables fully observed
7.2 Interactions of categorical variables 7.3 General nonlinear relationships 7.4 Software 7.5 Discussion PART III ADVANCED TOPICS
8 Survival data, skips and large datasets
8.1 Time-to-event data
8.1.1 Imputing missing covariate values
8.2 Nonparametric, or ‘hot deck’ imputation 8.1.2 Survival data as categorical 8.1.3 Imputing censored survival times
8.2.1 Nonparametric imputation for survival data
8.3 Multiple imputation for skips 8.4 Two-stage MI 8.5 Large datasets
8.5.1 Large datasets and joint modelling
8.6 Multiple imputation and record linkage 8.5.2 Shrinkage by constraining parameters 8.5.3 Comparison of the two approaches 8.7 Measurement error 8.8 Multiple imputation for aggregated scores 8.9 Discussion 9 Multilevel multiple imputation
9.1 Multilevel imputation model
9.2 MCMC algorithm for imputation model 9.3 Imputing level-2 covariates using FCS 9.4 Individual patient meta-analysis
9.4.1 When to apply Rubin’s rules
9.5 Extensions
9.5.1 Random level-1 covariance matrices
9.6 Discussion 9.5.2 Model fit 10 Sensitivity analysis: MI unleashed
10.1 Review of MNAR modelling
10.2 Framing sensitivity analysis 10.3 Pattern mixture modelling with MI
10.3.1 Missing covariates
10.4 Pattern mixture approach with longitudinal data via MI 10.3.2 Application to survival analysis
10.4.1 Change in slope post-deviation
10.5 Piecing together post-deviation distributions from other trial arms 10.6 Approximating a selection model by importance weighting
10.6.1 Algorithm for approximate sensitivity analysis by re-weighting
10.7 Discussion 11 Including survey weights
11.1 Using model based predictions
11.2 Bias in the MI variance estimator
11.2.1 MI with weights
11.3 A multilevel approach 11.2.2 Estimation in domains 11.4 Further developments 11.5 Discussion 12 Robust multiple imputation
12.1 Introduction
12.2 Theoretical background
12.2.1 Simple estimating equations
12.3 Robust multiple imputation 12.2.2 The Probability Of Missingness (POM) model 12.2.3 Augmented inverse probability weighted estimating equation
12.3.1 Univariate MAR missing data
12.4 Simulation studies 12.3.2 Longitudinal MAR missing data
12.4.1 Univariate MAR missing data
12.5 The RECORD study 12.4.2 Longitudinal monotone MAR missing data 12.4.3 Longitudinal nonmonotone MAR missing data 12.4.4 Nonlongitudinal nonmonotone MAR missing data 12.4.5 Results and discussion 12.6 Discussion Appendix A Markov Chain Monte Carlo
Appendix B Probability distributions
B.1 Posterior for the multivariate normal distribution
Bibliography
Index of Authors
Index of Examples
Index
|
Learn
Free webinars
NetCourses
Classroom and web training
Organizational training
Video tutorials
Third-party courses
Web resources
Teaching with Stata
© Copyright 1996–2025 StataCorp LLC. All rights reserved.
×
We use cookies to ensure that we give you the best experience on our website—to enhance site navigation, to analyze usage, and to assist in our marketing efforts. By continuing to use our site, you consent to the storing of cookies on your device and agree to delivery of content, including web fonts and JavaScript, from third party web services.
Cookie Settings
Last updated: 16 November 2022
StataCorp LLC (StataCorp) strives to provide our users with exceptional products and services. To do so, we must collect personal information from you. This information is necessary to conduct business with our existing and potential customers. We collect and use this information only where we may legally do so. This policy explains what personal information we collect, how we use it, and what rights you have to that information.
These cookies are essential for our website to function and do not store any personally identifiable information. These cookies cannot be disabled.
This website uses cookies to provide you with a better user experience. A cookie is a small piece of data our website stores on a site visitor's hard drive and accesses each time you visit so we can improve your access to our site, better understand how you use our site, and serve you content that may be of interest to you. For instance, we store a cookie when you log in to our shopping cart so that we can maintain your shopping cart should you not complete checkout. These cookies do not directly store your personal information, but they do support the ability to uniquely identify your internet browser and device.
Please note: Clearing your browser cookies at any time will undo preferences saved here. The option selected here will apply only to the device you are currently using.