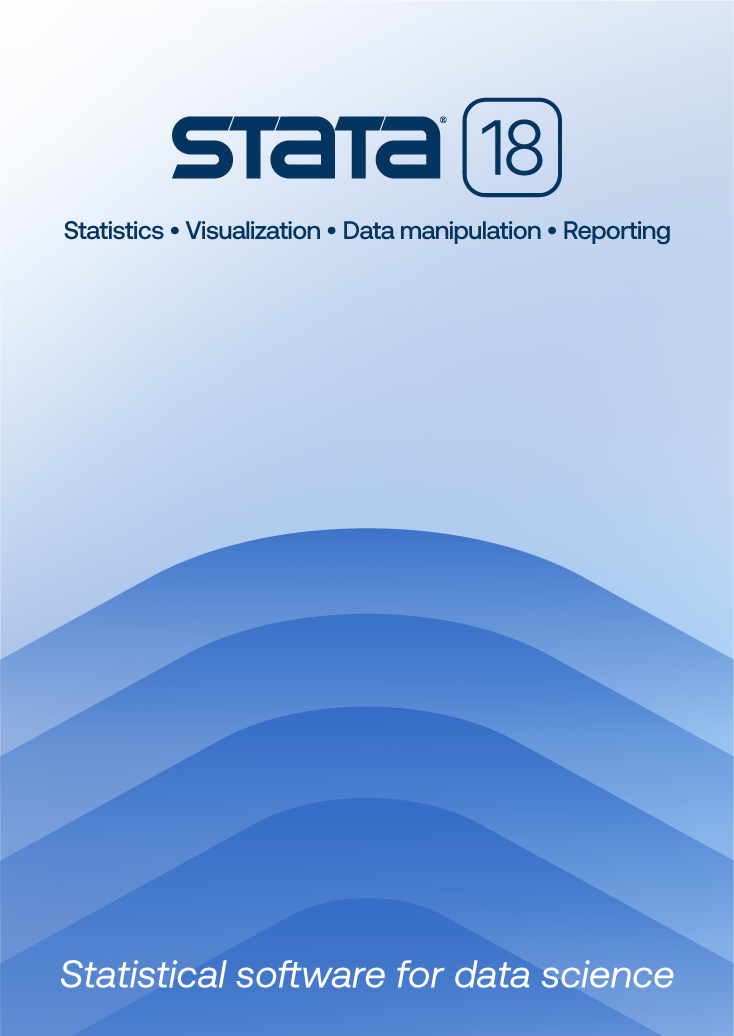
Modeling Count Data |
||||||||||||||||||||||||||||||||||||||
![]() Click to enlarge See the back cover |
![]()
As an Amazon Associate, StataCorp earns a small referral credit from
qualifying purchases made from affiliate links on our site.
eBook not available for this title
eBook not available for this title |
|
||||||||||||||||||||||||||||||||||||
Comment from the Stata technical groupComments from the publisherIn Modeling Count Data, examples demonstrate how to fit models using a combination of official and community-contributed commands in Stata. In addition, Stata datasets and code are available at the author's website. See the following editorial reviews from the publisher:
Awards: |
||||||||||||||||||||||||||||||||||||||
Table of contentsView table of contents >>
Preface
Chapter 1 Varieties of Count Data
Some Points of Discussion
1.1 What Are Counts? 1.2 Understanding a Statistical Count Model
1.2.1 Basic Structure of a Linear Statistical Model
1.3 Varieties of Count Models 1.2.2 Models and Probability 1.2.3 Count Models 1.2.4 Structure of a Count Model 1.4 Estimation – the Modeling Process
1.4.1 Software for Modeling
1.5 Summary
1.4.2 Maximum Likelihood Estimation 1.4.3 Generalized Linear Models and IRLS Estimation
Chapter 2
Poisson Regression
Some Points of Discussion
2.1 Poisson Model Assumptions 2.2 Apparent Overdispersion 2.3 Constructing a "True" Poisson Model 2.4 Poisson Regression: Modeling Real Data 2.5 Interpreting Coefficients and Rate Ratios
2.5.1 How to Interpret a Poisson Coefficient and Associated Statistics
2.6 Exposure: Modeling over Time, Area, and Space 2.5.2 Rate Ratios and Probability 2.7 Prediction 2.8 Poisson Marginal Effects
2.8.1 Marginal Effect at the Mean
2.9 Summary
2.8.2 Average Marginal Effects 2.8.3 Discrete Change or Partial Effects
Chapter 3
Testing Overdispersion
Some Points of Discussion
3.1 Basics of Count Model Fit Statistics 3.2 Overdispersion: What, Why, and How 3.3 Testing Overdispersion
3.3.1 Score Test
3.4 Methods of Handling Overdispersion 3.3.2 Lagrange Multiplier Test 3.3.3 Chi2 Test: Predicted versus Observed Counts
3.4.1 Scaling Standard Errors: Quasi-count Models
3.5 Summary
3.4.2 Quasi-likelihood Models 3.4.3 Sandwich or Robust Variance Estimators 3.4.4 Bootstrapped Standard Errors
Chapter 4
Assessment of Fit
Some Points of Discussion
4.1 Analysis of Residual Statistics 4.2 Likelihood Ratio Test
4.2.1 Standard Likelihood Ratio Test
4.3 Model Selection Criteria 4.2.2 Boundary Likelihood Ratio Test
4.3.1 Akaike Information Criterion
4.4 Setting up and Using a Validation Sample 4.3.2. Bayesian Information Criterion 4.5 Summary and an Overview of the Modeling Process
4.5.1 Summary of What We Have Thus Far Discussed
Chapter 5
Negative Binomial Regression
Some Points of Discussion
5.1 Varieties of Negative Binomial Models 5.2 Negative Binomial Model Assumptions
5.2.1 A Word Regarding Parameterization of the Negative Binomial
5.3 Two Modeling Examples
5.3.1 Example: rwm1984
5.4 Additional Tests
5.3.2 Example: medpar
5.4.1 General Negative Binomial Fit Tests
5.5 Summary
5.4.2 Adding a Parameter – NB-P Negative Binomial 5.4.3 Modeling the Dispersion – Heterogeneous Negative Binomial
Chapter 6
Poisson Inverse Gaussian Regression
Some Points of Discussion
6.1 Poisson Inverse Gaussian Model Assumptions 6.2 Constructing and Interpreting the PIG Model
6.2.1 Software Considerations
6.3 Summary – Comparing Poisson, NB, and PIG Models
6.2.2 Examples
Chapter 7
Problems with Zeros
Some Points of Discussion
7.1 Counts without Zeros – Zero-Truncated Models
7.1.1 Zero-Truncated Poisson (ZTP)
7.2 Two-Part Hurdle Models
7.1.2 Zero-Truncated Negative Binomial (ZTNB) 7.1.3 Zero-Truncated Poisson Inverse Gaussian (ZTPIG) 7.1.4 Zero-Truncated NB-P (ZTNBP) 7.1.5 Zero-Truncated Poisson Log-Normal (ZTPLN) 7.1.6 Zero-Truncated Model Summary
7.2.1 Poisson and Negative Binomial Logit Hurdle Models
7.3 Zero-Inflated Mixture Models
7.2.2 PIG-Logit and Poisson Log-Normal Hurdle Models 7.2.3 PIG-Poisson Hurdle Model
7.3.1 Overview of Guidelines
7.4 Summary – Finding the Optimal Model
7.3.2 Fit Tests for Zero-Inflated Models 7.3.3 Fitting Zero-Inflated Models 7.3.4 Good and Bad Zeros 7.3.5 Zero-Inflated Poisson (ZIP) 7.3.6 Zero-Inflated Negative Binomial (ZINB) 7.3.7 Zero-Inflated Poisson Inverse Gaussian (ZIPIG)
Chapter 8
Modeling Underdispersed Count Data – Generalized Poisson
Some Points of Discussion
Chapter 9
Complex Data: More Advanced Models
Types of Data and Problems Dealt with in This Chapter
9.1 Small and Unbalanced Data – Exact Poisson Regression 9.2 Modeling Truncated and Censored Counts
9.2.1 Truncated Count Models
9.3 Counts with Multiple Components – Finite Mixture Models 9.2.2 Censored Count Models 9.2.3 Poisson-Logit Hurdle at 3 Model 9.4 Adding Smoothing Terms to a Model – GAM 9.5 When All Else Fails: Quantile Count Models 9.6 A Word about Longitudinal and Clustered Count Models
9.6.1 Generalized Estimating Equations (GEEs)
9.7 Three-Parameter Count Models 9.6.2 Mixed-Effects and Multilevel Models 9.8 Bayesian Count Models – Future Directions of Modeling? 9.9 Summary
Appendix: SAS Code
Bibliography Index |
Learn
Free webinars
NetCourses
Classroom and web training
Organizational training
Video tutorials
Third-party courses
Web resources
Teaching with Stata
© Copyright 1996–2025 StataCorp LLC. All rights reserved.
×
We use cookies to ensure that we give you the best experience on our website—to enhance site navigation, to analyze usage, and to assist in our marketing efforts. By continuing to use our site, you consent to the storing of cookies on your device and agree to delivery of content, including web fonts and JavaScript, from third party web services.
Cookie Settings
Last updated: 16 November 2022
StataCorp LLC (StataCorp) strives to provide our users with exceptional products and services. To do so, we must collect personal information from you. This information is necessary to conduct business with our existing and potential customers. We collect and use this information only where we may legally do so. This policy explains what personal information we collect, how we use it, and what rights you have to that information.
These cookies are essential for our website to function and do not store any personally identifiable information. These cookies cannot be disabled.
This website uses cookies to provide you with a better user experience. A cookie is a small piece of data our website stores on a site visitor's hard drive and accesses each time you visit so we can improve your access to our site, better understand how you use our site, and serve you content that may be of interest to you. For instance, we store a cookie when you log in to our shopping cart so that we can maintain your shopping cart should you not complete checkout. These cookies do not directly store your personal information, but they do support the ability to uniquely identify your internet browser and device.
Please note: Clearing your browser cookies at any time will undo preferences saved here. The option selected here will apply only to the device you are currently using.