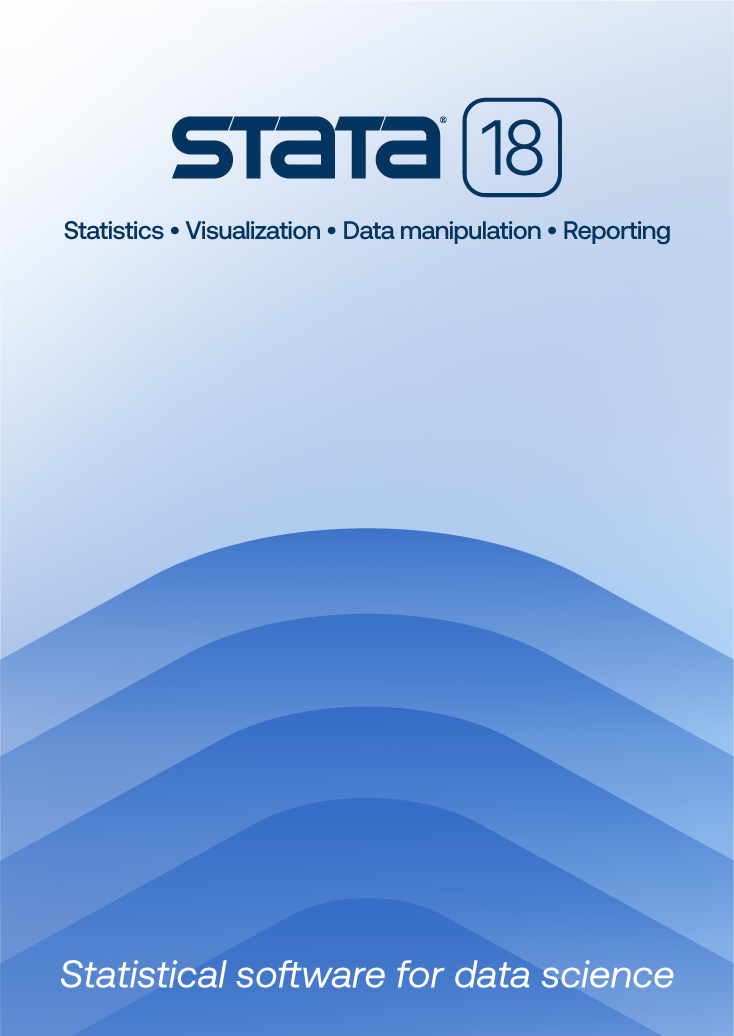
Guide pratique d’introduction à la régression en sciences sociales |
||||||||||||||||||||||||||||||||||||||
![]() Click to enlarge See the back cover |
![]()
As an Amazon Associate, StataCorp earns a small referral credit from
qualifying purchases made from affiliate links on our site.
eBook not available for this title
eBook not available for this title |
|
||||||||||||||||||||||||||||||||||||
Comment from the Stata technical groupGuide pratique d’introduction à la régression en sciences sociales, by François Pétry and François Gélineau, offers a brief introduction to exploratory data analysis and applies examples to illustrate the empirical elements associated with linear and logistic regressions. Chapters 1 and 2 review some of the aspects involved in describing data and preparing them for regression analysis. A number of graphs implemented in Stata are used to examine the behavior of the principal variables associated with the empirical examples. In chapters 3 and 4, the authors work with data on infant mortality to illustrate the use of simple and multivariate linear regression models. In addition to presenting a series of regression outputs produced in Stata, Pétry and Gélineau describe diagnostic tools that are normally used in standard linear regression. Chapter 5 provides a short description of the basic elements involved in time-series regression analysis. It discusses the detection of autocorrelation, along with the Prais–Winsten approach to fitting models with autocorrelated errors of order 1. The second part of the chapter describes a case in which government expenses are a function of gross domestic product (GDP). Through the case study, the authors introduce the basic aspects associated with the treatment of nonstationary series in regression analysis. Stata is used to produce most of the graphs and regression outputs contained in this chapter and those that follow. Chapters 6 and 7 show a case that regards people’s participation in general elections. In chapter 6, the authors fit a logistic model for a binary dependent variable that indicates whether the person voted. The application is extended in chapter 7 by fitting a multinomial model for a categorical dependent variable that is constructed on the basis of participation in the current and previous elections. The empirical examples and the inclusion of graphs, regression outputs, and summary tables provide smooth reading for the introduction of the technical concepts contained in the text. |
||||||||||||||||||||||||||||||||||||||
Table of contentsView table of contents >> Table des matières
Préface
Avant-propos de la deuxième édition
Chapitre 1 : Comment construire une recherche empirique
1.1 Introduction
1.2 Formulation du problème
1.2.1 Un problème doit être motivé
1.3 Cadre opératoire1.2.2 Un problème s’énonce sous forme de question
1.3.1 L’hypothèse
1.4 Structuration de la preuve1.3.2 L’unité d’analyse 1.3.3 La variable 1.3.4 L’indicateur 1.5 Cueillette des données 1.6 Analyse des données 1.7 Lectures recommandées
1.7.1 Méthodologie de la recherche
1.7.2 Manuels d’introduction aux statistiques Chapitre 2 : L’analyse exploratoire univariée
2.1 Introduction
2.2 La mise en ordre des observations 2.3 Les sommaires numériques
2.3.1 Les mesures de tendance centrale
2.4 La représentation graphique des données univariées2.3.2 Les mesures de position 2.3.3 Les mesures de dispersion 2.3.4 Les mesures de la forme d’une distribution
2.4.1 La tableau de distribution de fréquences (histogramme)
2.5 Mise en garde à propos des variables binaires et des séries temporelles2.4.2 Les diagrammes en « boîte à moustaches » 2.4.3 Le diagramme quantile 2.4.4 Quelques principes de représentation graphique 2.6 La transformation des données et l’étude exploratoire des données transformées
2.6.1 Règles générales de la transformation
2.7 Approche confirmatoire2.6.2 Règles particulières de la transformation 2.6.3 Logarithmes dans la base 10 2.6.4 La division par paquets des données transformées
2.7.1 Propriétés d’une distribution normale
2.8 Lectures recommandées2.7.2 Scores standardisés (scores Z) 2.7.3 Distributions d’échantillonnages 2.7.4 Intervalles de confiance Chapitre 3 : La régression linéaire simple
3.1 Introduction
3.2 Constatations préliminaires
3.2.1 Association ne veut pas dire causalité
3.3 Concepts de l’analyse exploratoire bivariée et de la régression linéaire simple3.2.2 Relation inexacte nécessitant un ajustement 3.2.3 Relation linéaire
3.3.1 La droite des moindres carrés
3.4 Exemple d’ajustement linéaire : la relation entre mortalité infantile et dépenses en santé3.3.2 Coefficient de détermination (R2) 3.3.3 Le coefficient de corrélation
3.4.1 Le diagramme de dispersion
3.5 Validation du modèle de régression par l’étude des résidus3.4.2 Droites d’ajustement 3.4.3 Élasticité
3.5.1 Présentation graphique des résidus de régression
3.6 Estimation des paramètres et inférence3.5.2 Analyse des résidus 3.5.3 Que faire des valeurs extrêmes ?
3.6.1 Test de signification du coefficient de régression
Lectures recommandées3.6.2 Test de signification simplifié 3.6.3 Test de signification du coefficient de corrélation 3.6.4 Interprétation des résultats Chapitre 4 : Régression linéaire multiple
4.1 Introduction
4.2 Principes de régression multiple
4.2.1 Exemple avec trois variables
4.3 Combien de variables indépendantes ?4.2.2 Étude de la relation causale 4.2.3 Contrôle statistique
4.3.1 Exemple avec quatre variables
4.4 Interaction entre variables explicatives 4.3.2 Le R2 adjusté 4.3.3 Mesure de l’impact relatif des variables indépendantes : le coefficient de régression standardisé 4.3.4 Multicolinéarité 4.3.5 Sélection automatique des variables d’un modèle
4.4.1 Différentes techniques pour tester les effets d’interaction
4.5 Notions avancées4.4.2 Impact d’une variable dichotomique sur l’ordonnée à l’origine 4.4.3 Impact d’une variable dichotomique sur la pente de régression 4.4.4 Impacts d’une variable dichotomique à la fois sur la pente et sur l’ordonnée à l’origine
4.5.1 Modèles avec variables instrumentales et systèmes d’équations
4.6 Lectures recommandées4.5.2 Bootstrapping 4.5.3 Modélisation des relations non-linéaires Chapitre 5 : Séries temporelles
5.1 Introduction
5.2 L’autocorrélation
5.2.1 Comment diagnostiquer l’autocorrélation ?
5.3 La stationnarité5.2.2 Modèle autorégressif
5.3.1 Comment vérifier le postulat de stationnarité
5.4 Exemple : la croissance économique et les dépenses du gouvernement fédéral canadien5.3.2 Transformation des données par la méthode des différences
5.4.1 Transformation des données par la méthode Prais-Winsten
5.5 Notions avancées
5.5.1 Variables à la traîne
5.6 Données avec variation spatio-longitudinale5.5.2 Modèles autorégressifs d’ordre supérieur 5.5.3 Prévisions (forecasting) 5.5.4 Co-intégration 5.7 Lectures recommandées Chapitre 6 : L’analyse de régression logistique
6.1 Introduction
6.2 Problèmes avec la régression linéaire 6.3 La transformation logit 6.4 La régression logistique binaire
6.4.1 Coefficients de régression logistique
6.5 La régression logistique multinomiale6.4.2 Tests d’adéquation d’ensemble du modèle 6.4.3 Analyse des résidus 6.6 La régression logistique ordonnée 6.7 Notions avancées
6.7.1 La régression logistique conditionnelle
6.8 Lectures recommandées6.7.2 L’indépendance des solutions non pertinentes 6.7.3 Les autres modèles de maximum de vraisemblance Annexes : Tableaux statistiques
Annexe 1. Distribution du t
Annexe 2. Distribution du F Annexe 3. Distribution du chi-carré Annexe 4. Distribution normale (Z) Annexe 5. Durbin-Watson Annexe 6. Table de Dickey-Fuller Index
|
Learn
Free webinars
NetCourses
Classroom and web training
Organizational training
Video tutorials
Third-party courses
Web resources
Teaching with Stata
© Copyright 1996–2025 StataCorp LLC. All rights reserved.
×
We use cookies to ensure that we give you the best experience on our website—to enhance site navigation, to analyze usage, and to assist in our marketing efforts. By continuing to use our site, you consent to the storing of cookies on your device and agree to delivery of content, including web fonts and JavaScript, from third party web services.
Cookie Settings
Last updated: 16 November 2022
StataCorp LLC (StataCorp) strives to provide our users with exceptional products and services. To do so, we must collect personal information from you. This information is necessary to conduct business with our existing and potential customers. We collect and use this information only where we may legally do so. This policy explains what personal information we collect, how we use it, and what rights you have to that information.
These cookies are essential for our website to function and do not store any personally identifiable information. These cookies cannot be disabled.
This website uses cookies to provide you with a better user experience. A cookie is a small piece of data our website stores on a site visitor's hard drive and accesses each time you visit so we can improve your access to our site, better understand how you use our site, and serve you content that may be of interest to you. For instance, we store a cookie when you log in to our shopping cart so that we can maintain your shopping cart should you not complete checkout. These cookies do not directly store your personal information, but they do support the ability to uniquely identify your internet browser and device.
Please note: Clearing your browser cookies at any time will undo preferences saved here. The option selected here will apply only to the device you are currently using.