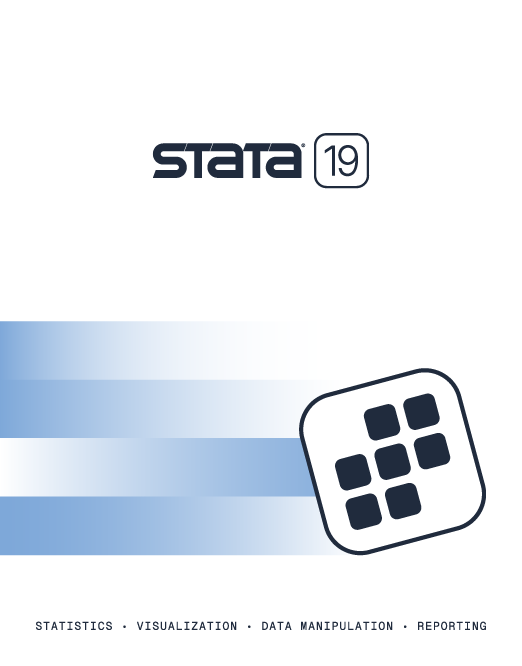
2025 Stata Conference • Nashville, TN • 31 July–01 August
Estimation and Inference in Econometrics |
||||||||||||||||||||||||||||||||||||
![]() Click to enlarge See the back cover |
![]()
As an Amazon Associate, StataCorp earns a small referral credit from
qualifying purchases made from affiliate links on our site.
eBook not available for this title
eBook not available for this title |
|
||||||||||||||||||||||||||||||||||
Comment from the Stata technical groupEstimation and Inference in Econometrics is a book that every serious student of econometrics should keep within arm’s reach. Davidson and MacKinnon provide a rather atypical insight into the theory and practice of econometrics. By itself, their exposition of the many uses of artificial regressions makes the book a valuable addition to any econometrician’s library. Suitable for graduate courses in econometrics, advanced self-study, or as a desk reference, this book receives our highest recommendation. |
||||||||||||||||||||||||||||||||||||
Table of contentsView table of contents >> 1 The Geometry of Least Square
1.1 Introduction
1.2 The Geometry of Least Squares 1.3 Restrictions and Reparametrizations 1.4 The Frisch-Waugh-Lovell Theorem 1.5 Computing OLS Estimates 1.6 Influential Observations and Leverage 1.7 Further Reading and Conclusion 2 Nonlinear Regression Models and Nonlinear Least Squares
2.1 Introduction
2.2 The Geometry of Nonlinear Least Squares 2.3 Identification in Nonlinear Regression Models 2.4 Models and Data-Generating Processes 2.5 Linear and Nonlinear Regression Functions 2.6 Error Terms 2.7 Conclusion 3 Inference in Nonlinear Regression Models
3.1 Introduction
3.2 Covariance Matrix Estimation 3.3 Confidence Intervals and Confidence Regions 3.4 Hypothesis Testing: Introduction 3.5 Hypothesis Testing in Linear Regression Models 3.6 Hypothesis Testing in Nonlinear Regression Models 3.7 Restrictions and Pretest Estimators Conclusion 4 Introduction to Asymptotic Theory and Methods
4.1 Introduction
4.2 Sequences, Limits, and Convergence 4.3 Rates of Convergence 4.4 Data-Generating Processes and Asymptotic Theory 4.5 Consistency and Laws of Large Numbers 4.6 Asymptotic Normality and Central Limit Theorems 4.7 Some Useful Results 4.8 Conclusion 5 Asymptotic Methods and Nonlinear Least Squares
5.1 Introduction
5.2 Asymptotic Identifiability 5.3 Consistency of the NLS Estimator 5.4 Asymptotic Normality of the NLS Estimator 5.5 Asymptotic Efficiency of Nonlinear Least Squares 5.6 Properties of Nonlinear Least Squares Residuals 5.7 Test Statistics Based on NLS Estimates 5.8 Further Reading and Conclusion 6 The Gauss–Newton Regression
6.1 Introduction
6.2 Computing Covariance Matrices 6.3 Collinearity in Nonlinear Regression Models 6.4 Testing Restrictions 6.5 Diagnostic Tests for Linear Regression Models 6.6 One-Step Efficient Estimation 6.7 Hypothesis Tests Using Any Consistent Estimates 6.8 Nonlinear Estimation Using the GNR 6.9 Further Reading 7 Instrumental Variables
7.1 Introduction
7.2 Errors in Variables 7.3 Simultaneous Equations 7.4 Instrumental Variables: The Linear Case 7.5 Two-Stage Least Squares 7.6 Instrumental Variables: The Nonlinear Case 7.7 Hypothesis Tests Based on the GNR 7.8 Identification and Overidentifying Restrictions 7.9 Durbin-Wu-Hausman Tests 7.10 Conclusion 8 The Method of Maximum Likelihood
8.1 Introduction
8.2 Fundamental Concepts and Notation 8.3 Transformations and Reparametrizations 8.4 Consistency 8.5 The Asymptotic Distribution of the ML Estimator 8.6 The Information Matrix Equality 8.7 Concentrating the Loglikelihood Function 8.8 Asymptotic Efficiency of the ML Estimator 8.9 The Three Classical Test Statistics 8.10 Nonlinear Regression Models 8.11 Conclusion 9 Maximum Likelihood and Generalized Least Squares
9.1 Introduction
9.2 Generalized Least Squares 9.3 The Geometry of GLS 9.4 The Gauss-Newton Regression 9.5 Feasible Generalized Least Squares 9.6 Maximum Likelihood and GNLS 9.7 Introduction to Multivariate Regression Models 9.8 GLS Estimation of Multivariate Regression Models 9.9 ML Estimation of Multivariate Regression Models 9.10 Modeling Time-Series/Cross-Section Data 9.11 Conclusion 10 Serial Correlation
10.1 Introduction
10.2 Serial Correlation and Least Squares Estimation 10.3 Estimating Regression Models with AR(1) Errors 10.4 Standard Errors and Covariance Matrices 10.5 Higher-Order AR Processes 10.6 Initial Observations in Models with AR Errors 10.7 Moving Average and ARMA Processes 10.8 Testing for Serial Correlation 10.9 Common Factor Restrictions 10.10 Instrumental Variables and Serial Correlation 10.11 Serial Correlation and Multivariate Models 10.12 Conclusion 11 Tests Based on the Gauss-Newton Regression
11.1 Introduction
11.2 Tests for Equality of Two Parameter Vectors 11.3 Testing Nonnested Regression Models 11.4 Tests Based on Comparing Two Sets of Estimates 11.5 Testing for Heteroskedasticity 11.6 A Heteroskedasticity-Robust Version of the GNR 11.7 Conclusion 12 Interpreting Tests in Regression Directions
12.1 Introduction
12.2 Size and Power 12.3 Drifting DGPs 12.4 The Asymptotic Distribution of Test Statistics 12.5 The Geometry of Test Power 12.6 Asymptotic Relative Efficiency 12.7 Interpreting Test Statistics that Reject the Null 12.8 Test Statistics that Do Not Reject the Null 12.9 Conclusion 13 The Classical Hypothesis Tests
13.1 Introduction
13.2 The Geometry of the Classical Test Statistics 13.3 Asymptotic Equivalence of the Classical Tests 13.4 Classical Tests and Linear Regression Models 13.5 Alternative Covariance Matrix Estimators 13.6 Classical Test Statistics 13.7 The Outer-Product-of-the-Gradient Regression 13.8 Further Reading and Conclusion 14 Transforming the Dependent Variable
14.1 Introduction
14.2 The Box-Cox Transformation 14.3 The Role of Jacobian Terms in ML Estimation 14.4 Double-Length Artificial Regressions 14.5 The DLR and Models Involving Transformations 14.6 Testing Linear and Loglinear Regression Models 14.7 Other Transformations 14.8 Conclusion 15 Qualitative and Limited Dependent Variables
15.1 Introduction
15.2 Binary Response Models 15.3 Estimation of Binary Response Models 15.4 An Artificial Regression 15.5 Models for More than Two Discrete Responses 15.6 Models for Truncated Data 15.7 Models for Censored Data 15.8 Sample Selectivity 15.9 Conclusion 16 Heteroskedasticity and Related Topics
16.1 Introduction
16.2 Least Squares and Heteroskedasticity 16.3 Covariance Matrix Estimation 16.4 Autoregressive Conditional Heteroskedasticity 16.5 Testing for Heteroskedasticity 16.6 Skedastic Directions and Regression Directions 16.7 Tests for Skewness and Excess Kurtosis 16.8 Conditional Moment Tests 16.9 Information Matrix Tests 16.10 Conclusion 17 The Generalized Method of Moments
17.1 Introduction and Definitions
17.2 Criterion Functions and M-Estimators 17.3 Efficient GMM Estimators 17.4 Estimation with Conditional Moments 17.5 Covariance Matrix Estimation 17.6 Inference with GMM Models 17.7 Conclusion 18 Simultaneous Equations Models
18.1 Introduction
18.2 Exogeneity and Causality 18.3 Identification in Simultaneous Equations Models 18.4 Full-Information Maximum Likelihood 18.5 Limited-Information Maximum Likelihood 18.6 Three-Stage Least Squares 18.7 Nonlinear Simultaneous Equations Models 18.8 Conclusion 19 Regression Models for Time-Series Data
19.1 Introduction
19.2 Spurious Regressions 19.3 Distributed Lags 19.4 Dynamic Regression Models 19.5 Vector Autoregressions 19.6 Seasonal Adjustment 19.7 Modeling Seasonality 19.8 Conclusion 20 Unit Roots and Cointegration
20.1 Introduction
20.2 Testing for Unit Roots 20.3 Asymptotic Theory for Unit Root Tests 20.4 Serial Correlation and Other Problems 20.5 Cointegration 20.6 Testing for Cointegration 20.7 Model-Building with Cointegrated Variables 20.8 Vector Autoregressions and Cointegration 20.9 Conclusion 21 Monte Carlo Experiments
21.1 Introduction
21.2 Generating Pseudo-Random Numbers 21.3 Generating Pseudo-Random Variates 21.4 Designing Monte Carlo Experiments 21.5 Variance Reduction: Antithetic Variates 21.6 Variance Reduction: Control Variates 21.7 Response Surfaces 21.8 The Bootstrap and Related Methods 21.9 Conclusion Appendices
A Matrix Algebra
A.1 Introduction
A.2 Elementary Facts about Matrices A.3 The Geometry of Vectors A.4 Matrices as Mappings of Linear Spaces A.5 Partitioned Matrices A.6 Determinants A.7 Positive Definite Matrices A.8 Eigenvalues and Eigenvectors B Results from Probability Theory
B.1 Introduction
B.2 Random Variables and Probability Distributions B.3 Moments of Random Variables B.4 Some Standard Probability Distributions References
Author Index
Subject Index
|
Learn
Free webinars
NetCourses
Classroom and web training
Organizational training
Video tutorials
Third-party courses
Web resources
Teaching with Stata
© Copyright 1996–2025 StataCorp LLC. All rights reserved.
×
We use cookies to ensure that we give you the best experience on our website—to enhance site navigation, to analyze usage, and to assist in our marketing efforts. By continuing to use our site, you consent to the storing of cookies on your device and agree to delivery of content, including web fonts and JavaScript, from third party web services.
Cookie Settings
Last updated: 16 November 2022
StataCorp LLC (StataCorp) strives to provide our users with exceptional products and services. To do so, we must collect personal information from you. This information is necessary to conduct business with our existing and potential customers. We collect and use this information only where we may legally do so. This policy explains what personal information we collect, how we use it, and what rights you have to that information.
These cookies are essential for our website to function and do not store any personally identifiable information. These cookies cannot be disabled.
This website uses cookies to provide you with a better user experience. A cookie is a small piece of data our website stores on a site visitor's hard drive and accesses each time you visit so we can improve your access to our site, better understand how you use our site, and serve you content that may be of interest to you. For instance, we store a cookie when you log in to our shopping cart so that we can maintain your shopping cart should you not complete checkout. These cookies do not directly store your personal information, but they do support the ability to uniquely identify your internet browser and device.
Please note: Clearing your browser cookies at any time will undo preferences saved here. The option selected here will apply only to the device you are currently using.