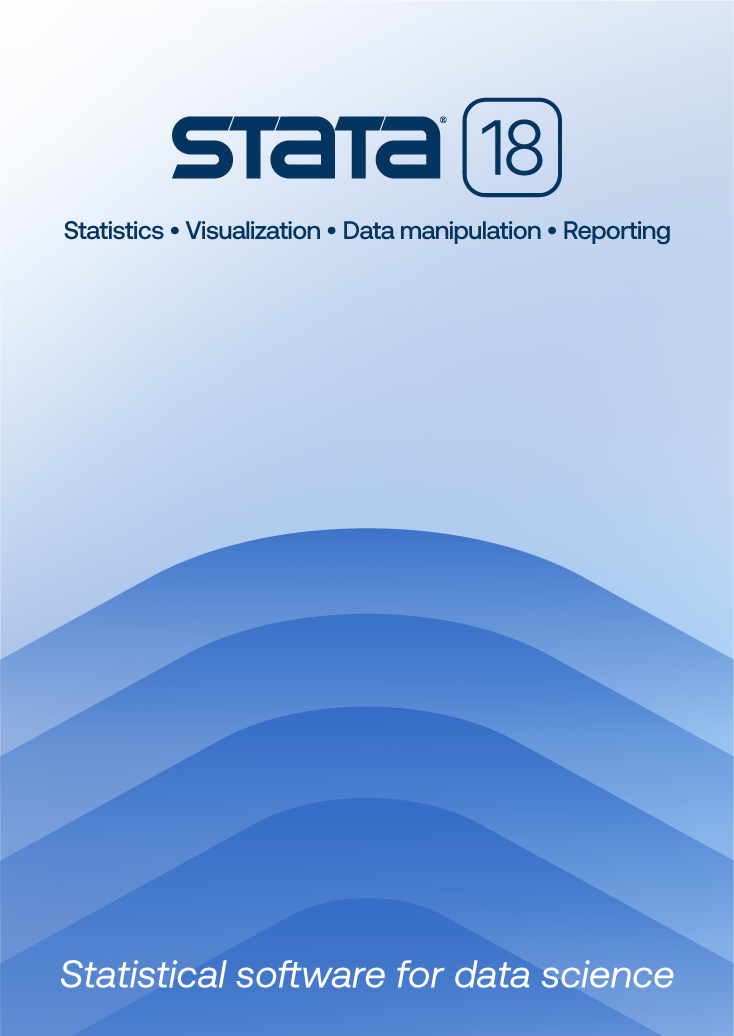
Econometrics in Theory and Practice |
||||||||||||||||||||||||||||||||
![]() Click to enlarge See the back cover |
![]()
As an Amazon Associate, StataCorp earns a small referral credit from
qualifying purchases made from affiliate links on our site.
eBook not available for this title
eBook not available for this title |
|
||||||||||||||||||||||||||||||
Comment from the Stata technical groupEconometrics in Theory and Practice by Panchanan Das is a book to learn how to use econometrics in practice. Unlike texts that focus on theory and place little weight on empirical applications, Das's aim is to emphasize how to apply the econometric tools. The instrument that ties theory and application of econometrics is statistical software. The instrument of choice— Stata. The book starts by introducing basic statistical and Stata concepts. It then proceeds to explain the linear regression model and talks about limited dependent variable models. These are the topics of the first two parts of the book. The third part books covers time-series analysis. The topics range from stationary to nonstationary time series and from univariate to multivariate time series. The third part also covers models for volatility and forecasting. The last section covers linear panel-data models and discusses nonstationary panel-data tests and dynamic panel-data models. This book is a helpful reference for those that want an introduction to econometric theory with an emphasis on how to apply these tools to their data. |
||||||||||||||||||||||||||||||||
Table of contentsView table of contents >> Part I Introductory Econometrics
1 Introduction to Econometrics and Statistical Software
1.1 Introduction
1.2 Economic Model and Econometric Model 1.3 Population Regression Function and Sample Regression Function 1.4 Parametric and Nonparametric or Semiparametric Model 1.5 Steps in Formulating an Econometric Model
1.5.1 Specification
1.6 Data1.5.2 Estimation 1.5.3 Testing of Hypothesis 1.5.4 Forecasting
1.6.1 Cross Section Data
1.7 Use of Econometric Software: Stata 15.11.6.2 Time Series Data 1.6.3 Pooled Cross Section 1.6.4 Panel Data
1.7.1 Data Management
1.8 Matrix Algebra1.7.2 Generating Variables 1.7.3 Describing Data 1.7.4 Graphs 1.7.5 Logical Operators in Stata 1.7.6 Functions Used in Stata
1.8.1 Matrix and Vector: Basic Operations
References
1.8.2 Partitioned Matrices 1.8.3 Rank of a Matrix 1.8.4 Inverse Matrix 1.8.5 Positive Definite Matrix 1.8.6 Trace of a Matrix 1.8.7 Orthogonal Vectors and Matrices 1.8.8 Eigenvalues and Eigenvectors 2 Linear Regression Model: Properties and Estimation
2.1 Introduction
2.2 The Simple Linear Regression Model 2.3 Multiple Linear Regression Model 2.4 Assumptions of Linear Regression Model
2.4.1 Non-stochastic Regressors
2.5 Methods of Estimation2.4.2 Linearity 2.4.3 Zero Unconditional Mean 2.4.4 Exogeneity 2.4.5 Homoscedasticity 2.4.6 Non-autocorrelation 2.4.7 Full Rank 2.4.8 Normal Distribution
2.5.1 The Method of Moments (MM)
2.6 Properties of the OLS Estimation2.5.2 The Method of Ordinary Least Squares (OLS) 2.5.3 Maximum Likelihood Method
2.6.1 Algebraic Properties
References
2.6.2 Statistical Properties 3 Linear Regression Model: Goodness of Fit and Testing of Hypothesis
3.1 Introduction
3.2 Goodness of Fit
3.2.1 The R2 as a Measure of Goodness of Fit
3.3 Testing of Hypothesis3.2.2 The Adjusted R2 as a Measure of Goodness of Fit
3.3.1 Sampling Distributions of the OLS Estimators
3.4 Linear Regression Model by Using Stata 15.13.3.2 Testing of Hypothesis for a Single Parameter 3.3.3 Use of P-Value 3.3.4 Interval Estimates 3.3.5 Testing of Hypotheses for More Than One Parameter: t Test 3.3.6 Testing Significance of the Regression: F Test 3.3.7 Testing for Linearity 3.3.8 Tests for Stability 3.3.9 Analysis of Variance 3.3.10 The Likelihood-Ratio, Wald and Lagrange Multiplier Test
3.4.1 OLS Estimation in Stata
References
3.4.2 Maximum Likelihood Estimation (MLE) in Stata 4 Linear Regression Model: Relaxing the Classical Assumptions
4.1 Introduction
4.2 Heteroscedasticity
4.2.1 Problems with Heteroscedastic Data
4.3 Autocorrelation4.2.2 Heteroscedasticity Robust Variance 4.2.3 Testing for Heteroscedasticity 4.2.4 Problem of Estimation 4.2.5 Illustration of Heteroscedastic Linear Regression by Using Stata
4.3.1 Linear Regression Model with Autocorrelated Error
References
4.3.2 Testing for Autocorrelation: Durbin—Watson Test 4.3.3 Consequences of Autocorrelation 4.3.4 Correcting for Autocorrelation 4.3.5 Illustration by Using Stata 5 Analysis of Collinear Data: Multicollinearity
5.1 Introduction
5.2 Multiple Correlation and Partial Correlation 5.3 Problems in the Presence of Multicollinearity 5.4 Detecting Multicollinearity
5.4.1 Determinant of (X'X)
5.5 Dealing with Multicollinearity5.4.2 Determinant of Correlation Matrix 5.4.3 Inspection of Correlation Matrix 5.4.4 Measure Based on Partial Regression 5.4.5 Theil's Measure 5.4.6 Variance Inflation Factor (VIF) 5.4.7 Eigenvalues and Condition Numbers 5.6 Illustration by Using Stata References Part II Advanced Analysis of Cross Section Data
6 Linear Regression Model: Qualitative Variables as Predictors
6.1 Introduction
6.2 Regression Model with Intercept Dummy
6.2.1 Dichotomous Factor
6.3 Regression Model with Interaction Dummy6.2.2 Polytomous Factors 6.4 Illustration by Using Stata 7 Limited Dependent Variable Model
7.1 Introduction
7.2 Linear Probability Model 7.3 Binary Response Models: Logit and Probit
7.3.1 The Logit Model
7.4 Maximum Likelihood Estimation of Logit and Probit Models7.3.2 The Probit Model 7.3.3 Difference Between Logit and Probit Models
7.4.1 Interpretation of the Estimated Coefficients
7.5 Regression Model with Truncated Distributions7.4.2 Goodness of Fit 7.4.3 Testing of Hypotheses 7.4.4 Illustration of Binary Response Model by Using Stata
7.5.1 Illustration of Truncated Regression by Using Stata
7.6 Problem of Censoring: Tobit Model
7.6.1 Illustration of Tobit Model by Using Stata
7.7 Models with Sample Selection Bias
7.7.1 Illustration of Sample Selection Model by Using Stata
7.8 Multinomial Logit Regression
7.8.1 Illustration by Using Stata
References
8 Multivariate Analysisl
8.1 Introduction
8.2 Displaying Multivariate Data
8.2.1 Multivariate Observations
8.3 Multivariate Normal Distribution8.2.2 Sample Mean Vector 8.2.3 Population Mean Vector 8.2.4 Covariance Matrix 8.2.5 Correlation Matrix 8.2.6 Linear Combination of Variables 8.4 Principal Component Anaylsis
8.4.1 Calculation of Principal Components
8.5 Factor Analysis8.4.2 Properties of Principal Components 8.4.3 Illustration by Using Stata
8.5.1 Orthogonal Factor Model
8.6 Multivariate Regression8.5.2 Estimation of Loadings and Communalities 8.5.3 Factor Loadings Are not Unique 8.5.4 Factor Rotation 8.5.5 Illustration by Using Stata
8.6.1 Structure of the Regression Model
References
8.6.2 Properties of Least Squares Estimators of B 8.6.3 Model Corrected for Means 8.6.4 Canonical Correlations Part III Analysis of Time Series Data
9 Time Series: Data Generating Process
9.1 Introduction
9.2 Data Generating Process (DGP)
9.2.1 Stationary Process
9.3 Methods of Time Series Analysis9.2.2 Nonstationary Process 9.4 Seasonality and Seasonal Adjustment 9.5 Creating a Time Variable by Using Stata References 10 Stationary Time Series
10.1 Introduction
10.2 Univariate Time Series Model 10.3 Autoregressive Process (AR)
10.3.1 The First-Order Autoregressive Process
10.4 The Moving Average (MA) Process10.3.2 The Second-Order Autoregressive Process 10.3.3 The Autoregressive Process of Order p 10.3.4 General Linear Processes
10.4.1 The First-Order Moving Average Process
10.5 Autoregressive Moving Average (ARMA) Process10.4.2 The Second-Order Moving Average Process 10.4.3 The Moving Average Process of Order q 10.4.4 Invertibility in Moving Average Process 10.6 Autocorrelation Function
10.6.1 Autocorrelation Function for AR(1)
10.7 Partial Autocorrelation Function (PACF)10.6.2 Autocorrelation Function for AR(2) 10.6.3 Autocorrelation Function for AR(p) 10.6.4 Autocorrelation Function for MA(1) 10.6.5 Autocorrelation Function for MA(2) 10.6.6 Autocorrelation Function for MA(p) 10.6.7 Autocorrelation Function for ARMA Process
10.7.1 Partial Autocorrelation for AR Series
10.8 Sample Autocorrelation Function10.7.2 Partial Autocorrelation for MA Series
10.8.1 Illustration by Using Stata
References
11 Nonstationarity, Unit Root and Structural Break
11.1 Introduction
11.2 Analysis of Trend
11.2.1 Deterministic Function of Time
11.3 Concept of Unit Root11.2.2 Stochastic Function of Time 11.2.3 Stochastic and Deterministic Function of Time 11.4 Unit Root Test
11.4.1 Dickey—Fuller Unit Root Test
11.5 Testing for Structural Break11.4.2 Augmented Dickey—Fuller (ADF) Unit Root Test 11.4.3 Phillips—Perron Unit Root Test 11.4.4 Dickey—Fuller GLS Test 11.4.5 Stationarity Tests 11.4.6 Multiple Unit Roots 11.4.7 Some Problems with Unit Root Tests 11.4.8 Macroeconomic Implications of Unit Root
11.5.1 Tests with Known Break Points
11.6 Unit Root Test with Break11.5.2 Tests with Unknown Break Points
11.6.1 When Break Point is Exogenous
11.7 Seasonal Adjustment11.6.2 When Break Point is Endogenous
11.7.1 Unit Roots at Various Frequencies: Seasonal Unit Root
11.8 Decomposition of a Time Series into Trend and Cycle11.7.2 Generating Time Variables and Seasonal Dummies in Stata References 12 Cointegration, Error Correction and Vector Autoregression
12.1 Introduction
12.2 Regression with Trending Variables 12.3 Concept of Cointegration 12.4 Granger's Representation Theorem 12.5 Testing for Cointegration: Engle—Granger's Two-Step Method
12.5.1 Illustrations by Using Stata
12.6 Vector Autoregression (VAR)
12.6.1 Stationarity Restriction of a Var Process
12.7 Vector Moving Average Processes12.6.2 Autocovariance Matrix of a VAR Process 12.6.3 Estimation of a VAR Process 12.6.4 Selection of Lag Length of a VAR Model 12.6.5 Illustration by Using Stata 12.8 Impulse Response Function
12.8.1 Illustration by Using Stata
12.9 Variance Decomposition12.10 Granger Causality
12.10.1 Illustration by Using Stata
12.11 Vector Error Correction Model
12.11.1 Illustration by Using Stata
12.12 Estimation and Testing of Hypotheses of Cointegrated Systems
12.12.1 Illustration by Using Stata
References
13 Modelling Volatility Clustering
13.1 Introduction
13.2 Modelling Non-constant Conditional Variance 13.3 The ARCH Model 13.4 The GARCH Model 13.5 Asymmetric ARCH Models 13.6 ARCH-in-Mean Model 13.7 Testing and Estimation of a GARCH Model
13.7.1 Testing for ARCH Effect
13.8 The ARCH Regression Model in Stata13.7.2 Maximum Likelihood Estimation for GARCH (1, 1)
13.8.1 Illustration with Market Capitalisation Data
References
14 Time Series Forecasting
14.1 Introduction
14.2 Simple Exponential Smoothing 14.3 Forecasting—Univariate Model 14.4 Forecasting with General Linear Processes 14.5 Multivariate Forecasting 14.6 Forecasting of a VAR Model 14.7 Forecasting GARCH Processes 14.8 Time Series Forecasting by Using Stata References Part IV Analysis of Panel Data
15 Panel Data Analysis: Static Models
15.1 Introduction
15.2 Structure and Types of Panel Data
15.2.1 Data Description by Using Stata 15.1
15.3 Benefits of Panel Data15.4 Sources of Variation in Panel Data 15.5 Unrestricted Model with Panel Data 15.6 Fully Restricted Model: Pooled Regression
15.6.1 Illustration by Using Stata
15.7 Error Component Model15.8 First-Differenced (FD) Estimator
15.8.1 Illustration by Using Stata
15.9 One-Way Error Component Fixed Effects Model
15.9.1 The "Within" Estimation
15.10 One-Way Error Component Random Effects Model15.9.2 Least Squares Dummy Variable (LSDV)
15.10.1 The GLS Estimation
References
15.10.2 Maximum Likelihood Estimation 15.10.3 Illustration by Using Stata 16 Panel Data Static Model: Testing of Hypotheses
16.1 Introduction
16.2 Measures of Goodness of Fit 16.3 Testing for Pooled Regression 16.4 Testing for Fixed Effects
16.4.1 Illustration by Using Stata
16.5 Testing for Random Effects
16.5.1 Illustration by Using Stata
16.6 Fixed or Random Effect: Hausman Test
16.6.1 Illustration by Using Stata
References
17 Panel Unit Root Test
17.1 Introduction
17.2 First-Generation Panel Unit Root Tests
17.2.1 Wu (1996) Unit Root Test
17.3 Stationarity Tests17.2.2 Levin, Lin and Chu Unit Root Test 17.2.3 Im, Pesaran and Shin (IPS) Unit Root Test 17.2.4 Fisher-Type Unit Root Tests
17.3.1 Illustration by Using Stata
17.4 Second-Generation Panel Unit Root Tests
17.4.1 The Covariance Restrictions Approach
References
17.4.2 The Factor Structure Approach 18 Dynamic Panel Model
18.1 Introduction
18.2 Linear Dynamic Model 18.3 Fixed and Random Effects Estimation
18.3.1 Illustration by Using Stata
18.4 Instrumental Variable Estimation
18.4.1 Illustration by Using Stata
18.5 Arellano—Bond GMM Estimator
18.5.1 Illustration by Using Stata
18.6 System GMM Estimator
18.6.1 Illustration by Using Stata
Appendix: Generalised Method of MomentsReferences |
Learn
Free webinars
NetCourses
Classroom and web training
Organizational training
Video tutorials
Third-party courses
Web resources
Teaching with Stata
© Copyright 1996–2024 StataCorp LLC. All rights reserved.
×
We use cookies to ensure that we give you the best experience on our website—to enhance site navigation, to analyze usage, and to assist in our marketing efforts. By continuing to use our site, you consent to the storing of cookies on your device and agree to delivery of content, including web fonts and JavaScript, from third party web services.
Cookie Settings
Last updated: 16 November 2022
StataCorp LLC (StataCorp) strives to provide our users with exceptional products and services. To do so, we must collect personal information from you. This information is necessary to conduct business with our existing and potential customers. We collect and use this information only where we may legally do so. This policy explains what personal information we collect, how we use it, and what rights you have to that information.
These cookies are essential for our website to function and do not store any personally identifiable information. These cookies cannot be disabled.
This website uses cookies to provide you with a better user experience. A cookie is a small piece of data our website stores on a site visitor's hard drive and accesses each time you visit so we can improve your access to our site, better understand how you use our site, and serve you content that may be of interest to you. For instance, we store a cookie when you log in to our shopping cart so that we can maintain your shopping cart should you not complete checkout. These cookies do not directly store your personal information, but they do support the ability to uniquely identify your internet browser and device.
Please note: Clearing your browser cookies at any time will undo preferences saved here. The option selected here will apply only to the device you are currently using.