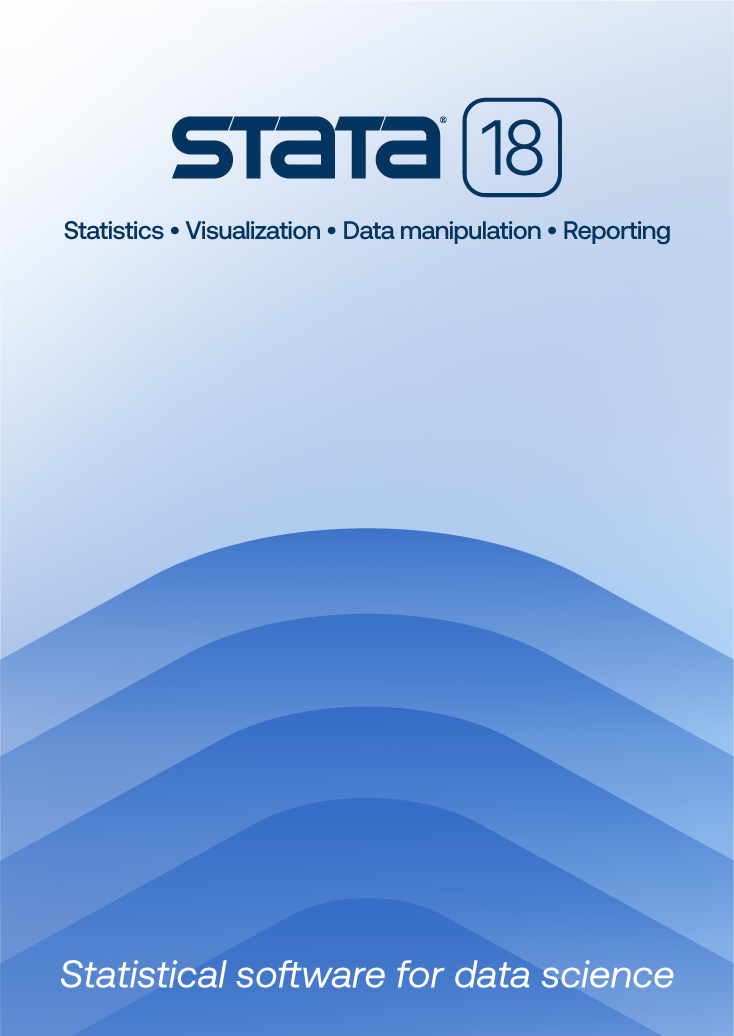
Econometric Analysis, Eighth Edition |
||||||||||||||||||||||||||||||||||||||
![]() Click to enlarge See the back cover |
![]()
As an Amazon Associate, StataCorp earns a small referral credit from
qualifying purchases made from affiliate links on our site.
eBook not available for this title
eBook not available for this title |
|
||||||||||||||||||||||||||||||||||||
Comment from the Stata technical groupWilliam Greene’s Econometric Analysis has been the standard reference for econometrics among economists, political scientists, and other social scientists for almost thirty years. As of 2016, the book had been cited more than 60,000 times; in 2014, it was part of Google Scholar’s list of 100 most cited works over all fields and for all time. The newly released eighth edition is certain to continue that tradition. This book’s abundance of examples and emphasis on putting econometric theory to practical use make it valuable not only to graduate students taking their first course in econometrics, but also to students and professionals who engage in empirical research. Part I of the book, chapters 1 to 6, covers regression modeling; properties of the least-squares estimator; inference and prediction; and tests for functional form and specification. Chapter 6 is of special interest. In this new edition, it introduces modern treatment effects concepts, such as regression discontinuity, as part of the basic analytical tool set in econometrics rather than a special topic to be presented in later chapters of the text. Part II of the book, chapters 7 to 11, covers extensions and deviations of the basic framework presented in Part I. Chapter 7 covers nonlinear models and contains a new discussion of interaction effects. Chapter 8 covers instrumental variables and endogeneity and has been revised to include more current methods and applications. Chapters 9 and 10 generalize the linear regression model to allow for heteroskedasticity. Then, with the generalized least-squares (GLS) estimator already discussed in the context of nonspherical disturbances, Greene presents fixed- and random-effects panel-data models as straightforward extensions of least squares. Chapter 11, which deals with panel data, has many revisions relevant to current research and applications, much like Chapter 8. Part III of the book, chapters 12 to 16, devotes one chapter to each of four popular estimation methods: the generalized method of moments, maximum likelihood, simulation, and Bayesian inference. Each chapter strikes a good balance between theoretical rigor and practical applications. Many newer discrete-choice models require evaluation of multivariate normal probabilities; to account for this, Chapter 15 includes a detailed discussion of the GHK simulator. Part IV of the book, chapters 17 to 19, covers advanced techniques for microeconometrics. Chapter 17 details binary choice models for both cross-sectional and panel data. Part IV also includes bivariate and multivariate probit models; models for count, multinomial, and ordered outcomes; and models for truncated data, duration data, and sample selection. Part V of the book, chapters 20 and 21, covers advanced techniques for macroeconometrics. Chapter 20, on stationary time series, describes estimation in the presence of serial correlation, tests for autocorrelation, lagged dependent variables, and ARCH models. Chapter 21, on nonstationary series, covers unit roots and cointegration. The chapters in Part V frequently use the results obtained in Part III on estimation. The book concludes with appendices on matrix algebra, probability, distribution theory, and optimization. These appendices are freely available at the book’s companion website. |
||||||||||||||||||||||||||||||||||||||
Table of contentsView table of contents >> Examples and Applications
Preface
Part I The Linear Regression Model
CHAPTER 1 Econometrics
1.1 Introduction
1.2 The Paradigm of Econometrics 1.3 The Practice of Econometrics 1.4 Microeconometrics and Macroeconometrics 1.5 Econometric Modeling 1.6 Plan of the Book 1.7 Preliminaries
1.7.1 Numerical Examples
1.7.2 Software and Replication 1.7.3 Notational Conventions CHAPTER 2 The Linear Regression Model
2.1 Introduction
2.2 The Linear Regression Model 2.3 Assumptions of the Linear Regression Model
2.3.1 Linearity of the Regression Model
2.4 Summary and Conclusions
2.3.2 Full Rank 2.3.3 Regression 2.3.4 Homoscedastic and Nonautocorrelated Disturbances 2.3.5 Data Generating Process for the Regressors 2.3.6 Normality 2.3.7 Independence and Exogeneity CHAPTER 3 Least Squares Regression
3.1 Introduction
3.2 Least Squares Regression
3.2.1 The Least Squares Coefficient Vector
3.3 Partitioned Regression and Partial Regression3.2.2 Application: An Investment Equation 3.2.3 Algebraic Aspects of the Least Squares Solution 3.2.4 Projection 3.4 Partial Regression and Partial Correlation Coefficients 3.5 Goodness of Fit and the Analysis of Variance
3.5.1 The Adjusted R-Squared and a Measure of Fit
3.6 Linearly Transformed Regression3.5.2 R-Squared and the Constant Term in the Model 3.5.3 Comparing Models 3.7 Summary and Conclusions CHAPTER 4 Estimating the Regression Model by Least Squares
4.1 Introduction
4.2 Motivating Least Squares
4.2.1 Population Orthogonality Conditions
4.3 Statistical Properties of the Least Squares Estimator4.2.2 Minimum Mean Squared Error Predictor 4.2.3 Minimum Variance Linear Unbiased Estimation
4.3.1 Unbiased Estimation
4.4 Asymptotic Properties of the Least Squares Estimator4.3.2 Omitted Variable Bias 4.3.3 Inclusion of Irrelevant Variables 4.3.4 Variance of the Least Squares Estimator 4.3.5 The Gauss–Markov Theorem 4.3.6 The Normality Assumption
4.4.1 Consistency of the Least Squares Estimator of Β
4.5 Robust Estimation and Inference4.4.2 The Estimator of Asy. Var[b] 4.4.3 Asymptotic Normality of the Least Squares Estimator 4.4.4 Asymptotic Efficiency 4.4.5 Linear Projections
4.5.1 Consistency of the Least Squares Estimator
4.6 Asymptotic Distribution of a Function of b:The Delta Method4.5.2 A Heteroscedasticity Robust Covariance Matrix for Least Squares 4.5.3 Robustness to Clustering 4.5.4 Bootstrapped Standard Errors with Clustered Data 4.7 Interval Estimation
4.7.1 Forming a Confidence Interval for a Coefficient
4.8 Prediction and Forecasting4.7.2 Confidence Interval for a Linear Combination of Coefficients: the Oaxaca Decomposition
4.8.1 Prediction Intervals
4.9 Data Problems4.8.2 Predicting y when the Regression Model Describes Log y 4.8.3 Prediction Interval for y when the Regression Model Describes Log y 4.8.4 Forecasting
4.9.1 Multicollinearity
4.10 Summary and Conclusions4.9.2 Principal Components 4.9.3 Missing Values and Data Imputation 4.9.4 Measurement Error 4.9.5 Outliers and Influential Observations CHAPTER 5 Hypothesis Tests and Model Selection
5.1 Introduction
5.2 Hypothesis Testing Methodology
5.2.1 Restrictions and Hypotheses
5.3 Three Approaches to Testing Hypotheses5.2.2 Nested Models 5.2.3 Testing Procedures 5.2.4 Size, Power, and Consistency of a Test 5.2.5 A Methodological Dilemma: Bayesian Versus Classical Testing
5.3.1 Wald Tests Based on the Distance Measure
5.4 Large-Sample Tests and Robust Inference
5.3.1.a Testing of a Hypothesis about a Coefficient
5.3.2 Tests Based on the Fit of the Regression5.3.1.b The F Statistic
5.3.2.a The Restricted Least Squares Estimator
5.3.3 Lagrange Multiplier Tests5.3.2.b The Loss of Fit from Restricted Least Squares 5.3.2.c Testing the Significance of the Regression 5.3.2.d Solving Out the Restrictions and a Caution about R2 5.5 Testing Nonlinear Restrictions 5.6 Choosing Between Nonnested Models
5.6.1 Testing Nonnested Hypotheses
5.7 A Specification Test5.6.2 An Encompassing Model 5.6.3 Comprehensive Approach — The J Test 5.8 Model Building — A General Guide to Simple Strategy
5.8.1 Model Selection Criteria
5.9 Summary and Conclusions5.8.2 Model Selection 5.8.3 Classical Model Selection 5.8.4 Bayesian Model Averaging CHAPTER 6 Functional Form, Difference in Differences, and Structural Change
6.1 Introduction
6.2 Using Binary Variables
6.2.1 Binary Variables in Regression
6.3 Difference in Differences Regression6.2.2 Several Categories 6.2.3 Modeling Individual Heterogeneity 6.2.4 Sets of Categories 6.2.5 Threshold Effects and Categorical Variables 6.2.6 Transition Tables
6.3.1 Treatment Effects
6.4 Using Regression Kinks and Discontinuities to Analyze Social Policy6.3.2 Examining the Effects of Discrete Policy Changes
6.4.1 Regression Kinked Design
6.5 Nonlinearity in the Variables6.4.2 Regression Discontinuity Design
6.5.1 Functional Forms
6.6 Structural Break and Parameter Variation6.5.2 Interaction Effects 6.5.3 Identifying Nonlinearity 6.5.4 Intrinsically Linear Models
6.6.1 Different Parameter Vectors
6.7 Summary and Conclusions6.6.2 Robust Tests of Structural Break with Unequal Variances 6.6.3 Pooling Regressions CHAPTER 7 Nonlinear, Semiparametric, and Nonparametric Regression Models
7.1 Introduction
7.2 Nonlinear Regression Models
7.2.1 Assumptions of the Nonlinear Regression Model
7.3 Median and Quantile Regression7.2.2 The Nonlinear Least Squares Estimator 7.2.3 Large-Sample Properties of the Nonlinear Least Squares Estimator 7.2.4 Robust Covariance Matrix Estimation 7.2.5 Hypothesis Testing and Parametric Restrictions 7.2.6 Applications 7.2.7 Loglinear Models 7.2.8 Computing the Nonlinear Least Squares Estimator
7.3.1 Least Absolute Deviations Estimation
7.4 Partially Linear Regression7.3.2 Quantile Regression Models 7.5 Nonparametric Regression 7.6 Summary and Conclusions CHAPTER 8 Endogeneity and Instrumental Variable Estimation
8.1 Introduction
8.2 Assumptions of the Extended Model 8.3 Instrumental Variables Estimation
8.3.1 Least Squares
8.4 Two-Stage Least Squares, Control Functions, and Limited Information Maximum Likelihood8.3.2 The Instrumental Variables Estimator 8.3.3 Estimating the Asymptotic Covariance Matrix 8.3.4 Motivating the Instrumental Variables Estimator
8.4.1 Two-Stage Least Squares
8.5 Endogenous Dummy Variables: Estimating Treatment Effects8.4.2 A Control Function Approach 8.4.3 Limited Information Maximum Likelihood
8.5.1 Regression Analysis of Treatment Effects
8.6 Hypothesis Tests8.5.2 Instrumental Variables 8.5.3 A Control Function Estimator 8.5.4 Propensity Score Matching
8.6.1 Testing Restrictions
8.7 Weak Instruments and LIML8.6.2 Specification Tests 8.6.3 Testing for Endogeneity: The Hausman and Wu Specification Tests 8.6.4 A Test for Overidentification 8.8 Measurement Error
8.8.1 Least Squares Attenuation
8.9 Nonlinear Instrumental Variables Estimation8.8.2 Instrumental Variables Estimation 8.8.3 Proxy Variables 8.10 Natural Experiments and the Search for Causal Effects 8.11 Summary and Conclusions Part II Generalized Regression Model and Equation Systems
CHAPTER 9 The Generalized Regression Model and Heteroscedasticity
9.1 Introduction
9.2 Robust Least Squares Estimation and Inference 9.3 Properties of Least Squares and Instrumental Variables
9.3.1 Finite-Sample Properties of Least Squares
9.4 Efficient Estimation by Generalized Least Squares9.3.2 Asymptotic Properties of Least Squares 9.3.3 Heteroscedasticity and Var[b | X] 9.3.4 Instrumental Variable Estimation
9.4.1 Generalized Least Squares (GLS)
9.5 Heteroscedasticity and Weighted Least Squares9.4.2 Feasible Generalized Least Squares (FGLS)
9.5.1 Weighted Least Squares
9.6 Testing for Heteroscedasticity9.5.2 Weighted Least Squares with Known Ω 9.5.3 Estimation When Ω Contains Unknown Parameters
9.6.1 White’s General Test
9.7 Two Applications9.6.2 The Lagrange Multiplier Test
9.7.1 Multiplicative Heteroscedasticity
9.8 Summary and Conclusions9.7.2 Groupwise Heteroscedasticity CHAPTER 10 Systems of Regression Equations
10.1 Introduction
10.2 The Seemingly Unrelated Regressions Model
10.2.1 Ordinary Least Squares And Robust Inference
10.3 Systems of Demand Equations: Singular Systems10.2.2 Generalized Least Squares 10.2.3 Feasible Generalized Least Squares 10.2.4 Testing Hypotheses 10.2.5 The Pooled Model
10.3.1 Cobb–Douglas Cost Function
10.4 Simultaneous Equations Models10.3.2 Flexible Functional Forms: The Translog Cost Function
10.4.1 Systems of Equations
10.5 Summary and Conclusions10.4.2 A General Notation for Linear Simultaneous Equations Models 10.4.3 The Identification Problem 10.4.4 Single Equation Estimation and Inference 10.4.5 System Methods of Estimation CHAPTER 11 Models for Panel Data
11.1 Introduction
11.2 Panel Data Modeling
11.2.1 General Modeling Framework for Analyzing Panel Data
11.3 The Pooled Regression Model11.2.2 Model Structures 11.2.3 Extensions 11.2.4 Balanced and Unbalanced Panels 11.2.5 Attrition and Unbalanced Panels 11.2.6 Well-Behaved Panel Data
11.3.1 Least Squares Estimation of the Pooled Model
11.4 The Fixed Effects Model11.3.2 Robust Covariance Matrix Estimation and Bootstrapping 11.3.3 Clustering and Stratification 11.3.4 Robust Estimation Using Group Means 11.3.5 Estimation with First Differences 11.3.6 The Within- and Between-Groups Estimators
11.4.1 Least Squares Estimation
11.5 Random Effects11.4.2 A Robust Covariance Matrix for bLSDV 11.4.3 Testing the Significance of the Group Effects 11.4.4 Fixed Time and Group Effects 11.4.5 Reinterpreting the Witin Estimator: Instrumental Variables and Control Functions 11.4.6 Parameter Heterogeneity
11.5.1 Least Squares Estimation
11.6 Nonspherical Disturbances and Robust Covariance Matrix Estimation11.5.2 Generalized Least Squares 11.5.3 Feasible Generalized Least Squares Estimation of the Random Effects Model when Σ is Unknown 11.5.4 Robust Inference and Feasible Generalized Least Squares 11.5.5 Testing for Random Effects 11.5.6 Hausman’s Specification Test for the Random Effects Model 11.5.7 Extending the Unobserved Effects Model: Mundlak’s Approach 11.5.8 Extending the Random and Fixed Effects Models: Chamberlain’s Approach
11.6.1 Heteroscedasticity in the Random Effects Model
11.7 Spatial Autocorrelation11.6.2 Autocorrelation in Panel Data Models 11.8 Endogeneity
11.8.1 Instrumental Variable Estimation
11.9 Nonlinear Regression with Panel Data11.8.2 Hausman and Taylor’s Instrumental Variables Estimator 11.8.3 Consistent Estimation of Dynamic Panel Data Models: Anderson and Hsiao’s Iv Estimator 11.8.4 Efficient Estimation of Dynamic Panel Data Models:The Arellano/Bond Estimators 11.8.5 Nonstationary Data and Panel Data Models
11.9.1 A Robust Covariance Matrix for Nonlinear Least Squares
11.10 Parameter Heterogeneity11.9.2 Fixed Effects in Nonlinear Regression Models 11.9.3 Random Effects
11.10.1 A Random Coefficients Model
11.11 Summary and Conclusions11.10.2 A Hierarchical Linear Model 11.10.3 Parameter Heterogeneity and Dynamic Panel Data Models Part III Estimation Methodology
CHAPTER 12 Estimation Frameworks in Econometrics
12.1 Introduction
12.2 Parametric Estimation and Inference
12.2.1 Classical Likelihood-Based Estimation
12.3 Semiparametric Estimation12.2.2 Modeling Joint Distributions with Copula Functions
12.3.1 GMM Estimation in Econometrics
12.4 Nonparametric Estimation12.3.2 Maximum Empirical Likelihood Estimation 12.3.3 Least Absolute Deviations Estimation and Quantile Regression 12.3.4 Kernel Density Methods 12.3.5 Comparing Parametric and Semiparametric Analyses
12.4.1 Kernel Density Estimation
12.5 Properties of Estimators
12.5.1 Statistical Properties of Estimators
12.6 Summary and Conclusions12.5.2 Extremum Estimators 12.5.3 Assumptions for Asymptotic Properties of Extremum Estimators 12.5.4 Asymptotic Properties of Estimators 12.5.5 Testing Hypotheses CHAPTER 13 Minimum Distance Estimation and the Generalized Method of Moments
13.1 Introduction
13.2 Consistent Estimation: The Method of Moments
13.2.1 Random Sampling and Estimating the Parameters of Distributions
13.3 Minimum Distance Estimation13.2.2 Asymptotic Properties of the Method of Moments Estimator 13.2.3 Summary—The Method of Moments 13.4 The Generalized Method of Moments (GMM) Estimator
13.4.1 Estimation Based on Orthogonality Conditions
13.5 Testing Hypotheses in the GMM Framework13.4.2 Generalizing the Method of Moments 13.4.3 Properties of the GMM Estimator
13.5.1 Testing the Validity of the Moment Restrictions
13.6 GMM Estimation of Econometric Models13.5.2 GMM Wald Counterparts to the Wald, LM, and LR Tests
13.6.1 Single-Equation Linear Models
13.7 Summary and Conclusions13.6.2 Single-Equation Nonlinear Models 13.6.3 Seemingly Unrelated Regression Equations 13.6.4 GMM Estimation of Dynamic Panel Data Models CHAPTER 14 Maximum Likelihood Estimation
14.1 Introduction
14.2 The Likelihood Function and Identification of the Parameters 14.3 Efficient Estimation: The Principle of Maximum Likelihood 14.4 Properties of the Maximum Likelihood Estimators
14.4.1 Regularity Conditions
14.5 Conditional Likelihoods and Econometric Models14.4.2 Properties of Regular Densities 14.4.3 The Likelihood Equation 14.4.4 The Information Matrix Equality 14.4.5 Asymptotic Properties of the Maximum Likelihood Estimator
14.4.5.a Consistency
14.4.6 Estimating the Asymptotic Variance of the Maximum Likelihood Estimator14.4.5.b Asymptotic Normality 14.4.5.c Asymptotic Efficiency 14.4.5.d Invariance 14.4.5.e Conclusion 14.6 Hypothesis and Specification Tests and Fit Measures
14.6.1 The Likelihood Ratio Test
14.7 Two-Step Maximum Likelihood Estimation14.6.2 The Wald Test 14.6.3 The Lagrange Multiplier Test 14.6.4 An Application of the Likelihood-Based Test Procedures 14.6.5 Comparing Models and Computing Model Fit 14.6.6 Vuong’s Test and the Kullback–Leibler Information Criterion 14.8 Pseudo-Maximum Likelihood Estimation and Robust Asymptotic Covariance Matrices
14.8.1 A Robust Covariance Matrix Estimator for the MLE
14.9 Maximum Likelihood Estimation of Linear Regression Models14.8.2 Cluster Estimators
14.9.1 Linear Regression Model with Normally Distributed Disturbances
14.10 The Generalized Regression Model14.9.2 Some Linear Models with Nonnormal Disturbances 14.9.3 Hypothesis Tests for Regression Models
14.10.1 GLS With Known Ω
14.11 Nonlinear Regression Models and Quasi-Maximum Likelihood Estimation14.10.2 Iterated Feasible GLS With Estimated Ω 14.10.3 Multiplicative Heteroscedasticity 14.10.4 The Method of Scoring
14.11.1 Maximum Likelihood Estimation
14.12 Systems of Regression Equations14.11.2 Quasi-Maximum Likelihood Estimation
14.12.1 The Pooled Model
14.13 Simultaneous Equations Models14.12.2 The SUR Model 14.14 Panel Data Applications
14.14.1 ML Estimation of the Linear Random Effects Model
14.15 Latent Class and Finite Mixture Models14.14.2 Nested Random Effects 14.14.3 Clustering Over More than One Level 14.14.4 Random Effects in Nonlinear Models: MLE Using Quadrature 14.14.5 Fixed Effects in Nonlinear Models: The Incidental Parameters Problem
14.15.1 A Finite Mixture Model
14.16 Summary and Conclusions14.15.2 Modeling the Class Probabilities 14.15.3 Latent Class Regression Models 14.15.4 Predicting Class Membership and βi 14.15.5 Determining the Number of Classes 14.15.6 A Panel Data Application 14.15.7 A Semiparametric Random Effects Model CHAPTER 15 Simulation-Based Estimation and Inference and Random Parameter Models
15.1 Introduction
15.2 Random Number Generation
15.2.1 Generating Pseudo-Random Numbers
15.3 Simulation-Based Statistical Inference: The Method of Krinsky and Robb15.2.2 Sampling from a Standard Uniform Population 15.2.3 Sampling from Continuous Distributions 15.2.4 Sampling from a Multivariate Normal Population 15.2.5 Sampling from Discrete Populations 15.4 Bootstrapping Standard Errors and Confidence Intervals
15.4.1 Types of Bootstraps
15.5 Monte Carlo Studies15.4.2 Bias Reduction with Bootstrap Estimators 15.4.3 Bootstrapping Confidence Intervals 15.4.4 Bootstrapping with Panel Data: The Block Bootstrap
15.5.1 A Monte Carlo Study: Behavior of a Test Statistic
15.6 Simulation-Based Estimation15.5.2 A Monte Carlo Study: The Incidental Parameters Problem
15.6.1 Random Effects in a Nonlinear Model
15.7 A Random Parameters Linear Regression Model15.6.2 Monte Carlo Integration
15.6.2.a Halton Sequences and Random Draws for Simulation-Based Integration
15.6.3 Simulation-Based Estimation of Random Effects Models15.6.2.b Computing Multivariate Normal Probabilities Using the GHK Simulator 15.8 Hierarchical Linear Models 15.9 Nonlinear Random Parameter Models 15.10 Individual Parameter Estimates 15.11 Mixed Models and Latent Class Models 15.12 Summary and Conclusions CHAPTER 16 Bayesian Estimation and Inference
16.1 Introduction
16.2 Bayes’ Theorem and the Posterior Density 16.3 Bayesian Analysis of the Classical Regression Model
16.3.1 Analysis with a Noninformative Prior
16.4 Bayesian Inference16.3.2 Estimation with an Informative Prior Density
16.4.1 Point Estimation
16.5 Posterior Distributions and the Gibbs Sampler16.4.2 Interval Estimation 16.4.3 Hypothesis Testing 16.4.4 Large-Sample Results 16.6 Application: Binomial Probit Model 16.7 Panel Data Application: Individual Effects Models 16.8 Hierarchical Bayes Estimation of a Random Parameters Model 16.9 Summary and Conclusions Part IV Cross Sections, Panel Data, and Microeconometrics
CHAPTER 17 Binary Outcomes and Discrete Choices
17.1 Introduction
17.2 Models for Binary Outcomes
17.2.1 Random Utility
17.3 Estimation and Inference for Binary Choice Models17.2.2 The Latent Regression Model 17.2.3 Functional Form and Probability 17.2.4 Partial Effects in Binary Choice Models 17.2.5 Odds Ratios in Logit Models 17.2.6 The Linear Probability Model
17.3.1 Robust Covariance Matrix Estimation
17.4 Measuring Goodness of Fit for Binary Choice Models17.3.2 Hypothesis Tests 17.3.3 Inference for Partial Effects
17.3.3.a The Delta Method
17.3.4 Interaction Effects17.3.3.b An Adjustment to the Delta Method 17.3.3.c The Method of Krinsky and Robb 17.3.3.d Bootstrapping
17.4.1 Fit Measures Based on the Fitting Criterion
17.5 Specification Analysis17.4.2 Fit Measures Based on Predicted Values 17.4.3 Summary of Fit Measures
17.5.1 Omitted Variables
17.6 Treatment Effects and Endogenous Variables in Binary Choice Models17.5.2 Heteroscedasticity 17.5.3 Distributional Assumptions 17.5.4 Choice-Based Sampling
17.6.1 Endogenous Treatment Effect
17.7 Panel Data Models17.6.2 Endogenous Continuous Variable
17.6.2.a IV and GMM Estimation
17.6.3 Endogenous Sampling17.6.2.b Partial ML Estimation 17.6.2.c Full Information Maximum Likelihood Estimation 17.6.2.d Residual Inclusion and Control Functions 17.6.2.e A Control Function Estimator
17.7.1 The Pooled Estimator
17.8 Spatial Binary Choice Models17.7.2 Random Effects 17.7.3 Fixed Effects
17.7.3.a A Conditional Fixed Effects Estimator
17.7.4 Dynamic Binary Choice Models17.7.3.b Mundlak’s Approach, Variable Addition, and Bias Reduction 17.7.5 A Semiparametric Model for Individual Heterogeneity 17.7.6 Modeling Parameter Heterogeneity 17.7.7 Nonresponse, Attrition, and Inverse Probability Weighting 17.9 The Bivariate Probit Model
17.9.1 Maximum Likelihood Estimation
17.10 A Multivariate Probit Model17.9.2 Testing for Zero Correlation 17.9.3 Partial Effects 17.9.4 A Panel Data Model for Bivariate Binary Response 17.9.5 A Recursive Bivariate Probit Model 17.11 Summary and Conclusions CHAPTER 18 Multinomial Choices and Event Counts
18.1 Introduction
18.2 Models for Unordered Multiple Choices
18.2.1 Random Utility Basis of the Multinomial Logit Model
18.3 Random Utility Models for Ordered Choices18.2.2 The Multinomial Logit Model 18.2.3 The Conditional Logit Model 18.2.4 The Independence from Irrelevant Alternatives Assumption 18.2.5 Alternative Choice Models
18.2.5.a Heteroscedastic Extreme Value Model
18.2.6 Modeling Heterogeneity18.2.5.b Multinomial Probit Model 18.2.5.c The Nested Logit Model
18.2.6.a The Mixed Logit Model
18.2.7 Estimating Willingness to Pay18.2.6.b A Generalized Mixed Logit Model 18.2.6.c Latent Classes 18.2.6.d Attribute Nonattendance 18.2.8 Panel Data and Stated Choice Experiments
18.2.8.a The Mixed Logit Model
18.2.9 Aggregate Market Share Data — The BLP Random Parameters Model18.2.8.b Random Effects and the Nested Logit Model 18.2.8.c A Fixed Effects Multinomial Logit Model
18.3.1 The Ordered Probit Model
18.4 Models for Counts of Events18.3.2 A Specification Test for the Ordered Choice Model 18.3.3 Bivariate Ordered Probit Models 18.3.4 Panel Data Applications
18.3.4.a Ordered Probit Models with Fixed Effects
18.3.5 Extensions of the Ordered Probit Model18.3.4.b Ordered Probit Models with Random Effects
18.3.5.a Threshold Models — Generalized Ordered Choice Models
18.3.5.b Thresholds and Heterogeneity — Anchoring Vignettes
18.4.1 The Poisson Regression Model
18.5 Summary and Conclusions18.4.2 Measuring Goodness of Fit 18.4.3 Testing for Overdispersion 18.4.4 Heterogeneity and the Negative Binomial Regression Model 18.4.5 Functional Forms for Count Data Models 18.4.6 Truncation and Censoring in Models for Counts 18.4.7 Panel Data Models
18.4.7.a Robust Covariance Matrices for Pooled Estimators
18.4.8 Two-Part Models: Zero-Inflation and Hurdle Models18.4.7.b Fixed Effects 18.4.7.c Random Effects 18.4.9 Endogenous Variables and Endogenous Participation CHAPTER 19 Limited Dependent Variables–Truncation, Censoring, and Sample Selection
19.1 Introduction
19.2 Truncation
19.2.1 Truncated Distributions
19.3 Censored Data19.2.2 Moments of Truncated Distributions 19.2.3 The Truncated Regression Model 19.2.4 The Stochastic Frontier Model
19.3.1 The Censored Normal Distribution
19.4 Sample Selection and Incidental Truncation19.3.2 The Censored Regression (Tobit) Model 19.3.3 Estimation 19.3.4 Two-Part Models and Corner Solutions 19.3.5 Specification Issues
19.3.5.a Endogenous Right-Hand-Side Variables
19.3.6 Panel Data Applications19.3.5.b Heteroscedasticity 19.3.5.c Nonnormality
19.4.1 Incidental Truncation in a Bivariate Distribution
19.5 Models for Duration19.4.2 Regression in a Model of Selection 19.4.3 Two-Step and Maximum Likelihood Estimation 19.4.4 Sample Selection in Nonlinear Models 19.4.5 Panel Data Applications of Sample Selection Models
19.4.5.a Common Effects in Sample Selection Models
19.4.5.b Attrition
19.5.1 Models for Duration Data
19.6 Summary and Conclusions19.5.2 Duration Data 19.5.3 A Regression-Like Approach: Parametric Models of Duration
19.5.3.a Theoretical Background
19.5.4 Nonparametric and Semiparametric Approaches19.5.3.b Models of the Hazard Function 19.5.3.c Maximum Likelihood Estimation 19.5.3.d Exogenous Variables 19.5.3.e Heterogeneity Part V Time Series and Macroeconometrics
CHAPTER 20 Serial Correlation
20.1 Introduction
20.2 The Analysis of Time-Series Data 20.3 Disturbance Processes
20.3.1 Characteristics of Disturbance Processes
20.4 Some Asymptotic Results for Analyzing Time-Series Data20.3.2 Ar(1) Disturbances
20.4.1 Convergence of Moments — The Ergodic Theorem
20.5 Least Squares Estimation20.4.2 Convergence to Normality — A Central Limit Theorem
20.5.1 Asymptotic Properties of Least Squares
20.6 GMM Estimation20.5.2 Estimating the Variance of the Least Squares Estimator 20.7 Testing for Autocorrelation
20.7.1 Lagrange Multiplier Test
20.8 Efficient Estimation when Ω is Known20.7.2 Box And Pierce’s Test and Ljung’s Refinement 20.7.3 The Durbin–Watson Test 20.7.4 Testing in the Presence of a Lagged Dependent Variable 20.7.5 Summary of Testing Procedures 20.9 Estimation when Ω is Unknown
20.9.1 Ar(1) Disturbances
20.10 Autoregressive Conditional Heteroscedasticity20.9.2 Application: Estimation of a Model with Autocorrelation 20.9.3 Estimation with a Lagged Dependent Variable
20.10.1 The ARCH(1) Model
20.11 Summary and Conclusions20.10.2 ARCH(q), ARCH-In-Mean, and Generalized ARCH Models 20.10.3 Maximum Likelihood Estimation of the GARCH Model 20.10.4 Testing for GARCH Effects 20.10.5 Pseudo-Maximum Likelihood Estimation CHAPTER 21 Nonstationary Data
21.1 Introduction
21.2 Nonstationary Processes and Unit Roots
21.2.1 The Lag and Difference Operators
21.3 Cointegration21.2.2 Integrated Processes and Differencing 21.2.3 Random Walks, Trends, and Spurious Regressions 21.2.4 Tests for Unit Roots in Economic Data 21.2.5 The Dickey–Fuller Tests 21.2.6 The KPSS Test of Stationarity
21.3.1 Common Trends
21.4 Nonstationary Panel Data21.3.2 Error Correction and Var Representations 21.3.3 Testing for Cointegration 21.3.4 Estimating Cointegration Relationships 21.3.5 Application: German Money Demand
21.3.5.a Cointegration Analysis and a Long-Run Theoretical Model
21.3.5.b Testing for Model Instability 21.5 Summary and Conclusions References
Index
Part VI Online Appendices
Appendix A Matrix Algebra
A.1 Terminology
A.2 Algebraic Manipulation of Matrices
A.2.1 Equality of Matrices
A.3 Geometry of MatricesA.2.2 Transposition A.2.3 Vectorization A.2.4 Matrix Addition A.2.5 Vector Multiplication A.2.6 A Notation for Rows and Columns of a Matrix A.2.7 Matrix Multiplication and Scalar Multiplication A.2.8 Sums of Values A.2.9 A Useful Idempotent Matrix
A.3.1 Vector Spaces
A.4 Solution of a System of Linear EquationsA.3.2 Linear Combinations of Vectors and Basis Vectors A.3.3 Linear Dependence A.3.4 Subspaces A.3.5 Rank of a Matrix A.3.6 Determinant of a Matrix A.3.7 A Least Squares Problem
A.4.1 Systems of Linear Equations
A.5 Partitioned MatricesA.4.2 Inverse Matrices A.4.3 Nonhomogeneous Systems of Equations A.4.4 Solving the Least Squares Problems
A.5.1 Addition and Multiplication of Partitioned Matrices
A.6 Characteristic Roots and VectorsA.5.2 Determinants of Partitioned Matrices A.5.3 Inverses of Partitioned Matrices A.5.4 Deviations from Means A.5.5 Kronecker Products
A.6.1 The Characteristic Equation
A.7 Quadratic Forms and Definite MatricesA.6.2 Characteristic Vectors A.6.3 General Results for Characteristic Roots and Vectors A.6.4 Diagonalization and Spectral Decomposition of a Matrix A.6.5 Rank of a Matrix A.6.6 Condition Number of a Matrix A.6.7 Trace of a Matrix A.6.8 Determinant of a Matrix A.6.9 Powers of a Matrix A.6.10 Idempotent Matrices A.6.11 Factoring a Matrix: The Cholesky Decomposition A.6.12 Singular Value Decomposition A.6.13 Qr Decomposition A.6.14 The Generalized Inverse of a Matrix
A.7.1 Nonnegative Definite Matrices
A.8 Calculus and Matrix AlgebraA.7.2 Idempotent Quadratic Forms A.7.3 Comparing Matrices
A.8.1 Differentiation and the Taylor Series
A.8.2 Optimization A.8.3 Constrained Optimization A.8.4 Transformations Appendix B Probability and Distribution Theory
B.1 Introduction
B.2 Random Variables
B.2.1 Probability Distributions
B.3 Expectations of a Random VariableB.2.2 Cumulative Distribution Function B.4 Some Specific Probability Distributions
B.4.1 The Normal and Skew Normal Distributions
B.5 The Distribution of a Function of a Random VariableB.4.2 The Chi-Squared, t, and F Distributions B.4.3 Distributions with Large Degrees of Freedom B.4.4 Size Distributions: The Lognormal Distribution B.4.5 The Gamma and Exponential Distributions B.4.6 The Beta Distribution B.4.7 The Logistic Distribution B.4.8 The Wishart Distribution B.4.9 Discrete Random Variables B.6 Representations of a Probability Distribution B.7 Joint Distributions
B.7.1 Marginal Distributions
B.8 Conditioning in a Bivariate DistributionB.7.2 Expectations in a Joint Distribution B.7.3 Covariance and Correlation B.7.4 Distribution of a Function of Bivariate Random Variables
B.8.1 Regression: The Conditional Mean
B.9 The Bivariate Normal DistributionB.8.2 Conditional Variance B.8.3 Relationships among Marginal and Conditional Moments B.8.4 The Analysis of Variance B.8.5 Linear Projection B.10 Multivariate Distributions
B.10.1 Moments
B.11 The Multivariate Normal DistributionB.10.2 Sets of Linear Functions B.10.3 Nonlinear Functions: The Delta Method
B.11.1 Marginal and Conditional Normal Distributions
B.11.2 The Classical Normal Linear Regression Model B.11.3 Linear Functions of a Normal Vector B.11.4 Quadratic Forms in a Standard Normal Vector B.11.5 The F Distribution B.11.6 A Full Rank Quadratic Form B.11.7 Independence of a Linear and a Quadratic Form Appendix C Estimation and Inference
C.1 Introduction
C.2 Samples and Random Sampling C.3 Descriptive Statistics C.4 Statistics as Estimators — Sampling Distributions C.5 Point Estimation of Parameters
C.5.1 Estimation in a Finite Sample
C.6 Interval EstimationC.5.2 Efficient Unbiased Estimation C.7 Hypothesis Testing
C.7.1 Classical Testing Procedures
C.7.2 Tests Based on Confidence Intervals C.7.3 Specification Tests Appendix D Large-Sample Distribution Theory
D.1 Introduction
D.2 Large-Sample Distribution Theory
D.2.1 Convergence in Probability
D.3 Asymptotic DistributionsD.2.2 Other forms of Convergence and Laws of Large Numbers D.2.3 Convergence of Functions D.2.4 Convergence to a Random Variable D.2.5 Convergence in Distribution: Limiting Distributions D.2.6 Central Limit Theorems D.2.7 The Delta Method
D.3.1 Asymptotic Distribution of a Nonlinear Function
D.4 Sequences and the Order of a SequenceD.3.2 Asymptotic Expectations Appendix E Computation and Optimization
E.1 Introduction
E.2 Computation in Econometrics
E.2.1 Computing Integrals
E.3 OptimizationE.2.2 The Standard Normal Cumulative Distribution Function E.2.3 The Gamma and Related Functions E.2.4 Approximating Integrals by Quadrature
E.3.1 Algorithms
E.4 ExamplesE.3.2 Computing Derivatives E.3.3 Gradient Methods E.3.4 Aspects of Maximum Likelihood Estimation E.3.5 Optimization with Constraints E.3.6 Some Practical Considerations E.3.7 The EM Algorithm
E.4.1 Function of one Parameter
E.4.2 Function of two Parameters: The Gamma Distribution E.4.3 A Concentrated Log-Likelihood Function Appendix F Data Sets Used in Applications
|
Learn
Free webinars
NetCourses
Classroom and web training
Organizational training
Video tutorials
Third-party courses
Web resources
Teaching with Stata
© Copyright 1996–2024 StataCorp LLC. All rights reserved.
×
We use cookies to ensure that we give you the best experience on our website—to enhance site navigation, to analyze usage, and to assist in our marketing efforts. By continuing to use our site, you consent to the storing of cookies on your device and agree to delivery of content, including web fonts and JavaScript, from third party web services.
Cookie Settings
Last updated: 16 November 2022
StataCorp LLC (StataCorp) strives to provide our users with exceptional products and services. To do so, we must collect personal information from you. This information is necessary to conduct business with our existing and potential customers. We collect and use this information only where we may legally do so. This policy explains what personal information we collect, how we use it, and what rights you have to that information.
These cookies are essential for our website to function and do not store any personally identifiable information. These cookies cannot be disabled.
This website uses cookies to provide you with a better user experience. A cookie is a small piece of data our website stores on a site visitor's hard drive and accesses each time you visit so we can improve your access to our site, better understand how you use our site, and serve you content that may be of interest to you. For instance, we store a cookie when you log in to our shopping cart so that we can maintain your shopping cart should you not complete checkout. These cookies do not directly store your personal information, but they do support the ability to uniquely identify your internet browser and device.
Please note: Clearing your browser cookies at any time will undo preferences saved here. The option selected here will apply only to the device you are currently using.