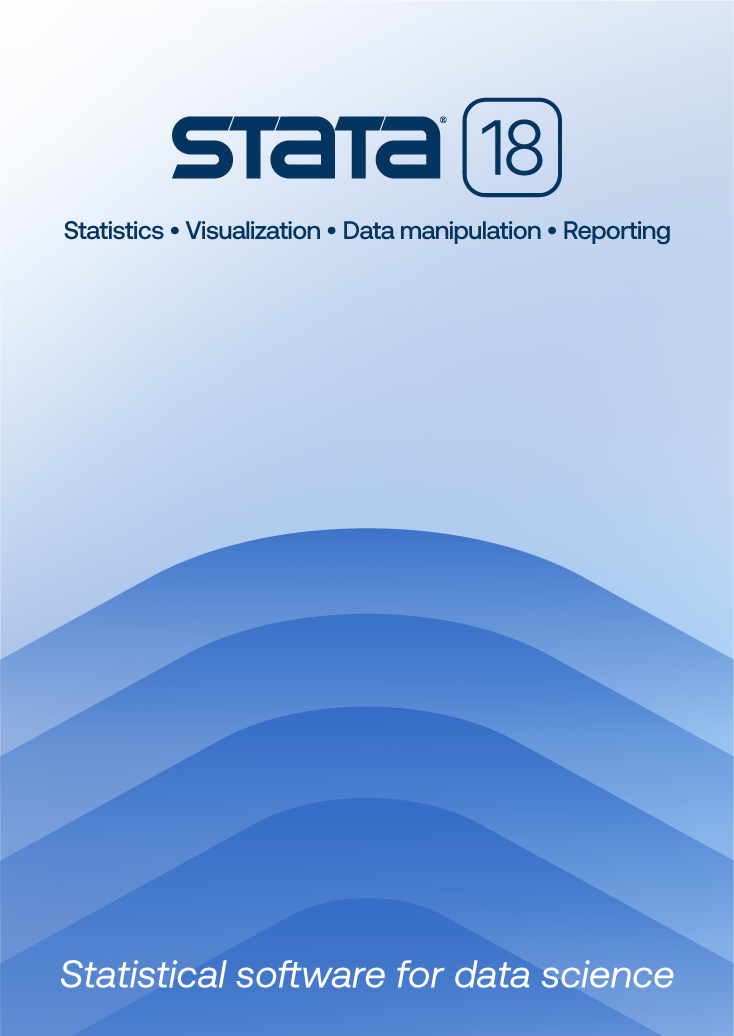
Competing Risks: A Practical Perspective |
||||||||||||||||||||||||||||||||||||
![]() Click to enlarge See the back cover |
![]()
As an Amazon Associate, StataCorp earns a small referral credit from
qualifying purchases made from affiliate links on our site.
eBook not available for this title
eBook not available for this title |
|
||||||||||||||||||||||||||||||||||
Comment from the Stata technical groupIn survival analysis, competing risks refers to events that impede the failure event of interest—death from unrelated causes during a study involving the recurrence of breast cancer, for example. Competing risks analysis is becoming an increasingly popular topic in medical research because practitioners have discovered that the standard assumptions made when treating competing events as censored are often violated in practice. Further confusing the issue is the fact that competing events sometimes can be treated as censored, which is more a computational device than an acceptance of the assumptions. This text introduces competing risks from first principles and definitions, and it covers the two primary methods for dealing with competing-risks analysis: cause-specific analysis and the calculation and modeling of cumulative incidence functions. For the latter, the concepts of subdistribution functions and subhazard functions are introduced. Both nonparametric analysis and semiparametric regression models are considered. The text uses a series of real datasets from the medical literature throughout, and most of the analyses can be reproduced in Stata 11. |
||||||||||||||||||||||||||||||||||||
Table of contentsView table of contents >> Preface
Acknowledgements
1 Introduction
1.1 Historical notes
1.2 Defining competing risks 1.3 Use of the Kaplan–Meier method in the presence of competing risks 1.4 Testing in the competing risk framework 1.5 Sample size calculation 1.6 Examples
1.6.1 Tamoxifen trial
1.6.2 Hypoxia study 1.6.3 Follicular cell lymphoma study 1.6.4 Bone marrow transplant study 1.6.5 Hodgkin’s disease study 2 Survival—basic concepts
2.1 Introduction
2.2 Definitions and background formulae
2.2.1 Introduction
2.3 Estimation and hypothesis testing 2.2.2 Basic mathematical formulae 2.2.3 Common parametric distributions 2.2.4 Censoring and assumptions
2.3.1 Estimating the hazard and survivor functions
2.4 Software for survival analysis 2.3.2 Nonparametric testing: log-rank and Wilcoxon tests 2.3.3 Proportional hazards model 2.5 Closing remarks 3 Competing risks—definitions
3.1 Recognizing competing risks
3.1.1 Practical approaches
3.2 Two mathematical definitions 3.1.2 Common endpoints in medical research
3.2.1 Competing risks as bivariate random variable
3.3 Fundamental concepts 3.2.2 Competing risks as latent failure times
3.3.1 Competing risks as bivariate random variable
3.4 Closing remarks 3.3.2 Competing risks as latent failure times 3.3.3 Discussion of the two approaches 4 Descriptive methods for competing risks data
4.1 Product-limit estimator and competing risks
4.2 Cumulative incidence function
4.2.1 Heuristic estimation of the CIF
4.3 Software and examples 4.2.2 Nonparametric maximum likelihood estimation of the CIF 4.2.3 Calculating the CIF estimator 4.2.4 Variance and confidence interval for the CIF estimator
4.3.1 Using R
4.4 Closing remarks 4.3.2 Using SAS 5 Testing a covariate
5.1 Introduction
5.2 Testing a covariate
5.2.1 Gray’s method
5.3 Software and examples 5.2.2 Pepe and Mori’s method
5.3.1 Using R
5.4 Closing remarks 5.3.2 Using SAS 6 Modelling in the presence of competing risks
6.1 Introduction
6.2 Modelling the hazard of the cumulative incidence function
6.2.1 Theoretical details
6.3 Cox model and competing risks 6.2.2 Model-based estimation of the CIF 6.2.3 Using R 6.4 Checking the model assumptions
6.4.1 Proportionality of the cause-specific hazards
6.5 Closing remarks 6.4.2 Proportionality of the hazards of the CIF 6.4.3 Linearity assumption 7 Calculating the power in the presence of competing risks
7.1 Introduction
7.2 Sample size calculation when competing risks are not present 7.3 Calculating power in the presence of competing risks
7.3.1 General formulae
7.4 Examples 7.3.2 Comparing cause-specific hazards 7.3.3 Comparing hazards of the subdistributions 7.3.4 Probability of event when the exponential distribution is not a valid assumption
7.4.1 Introduction
7.5 Closing remarks 7.4.2 Comparing the cause-specific hazard 7.4.3 Comparing the hazard of the subdistribution 8 Other issues in competing risks
8.1 Conditional probability function
8.1.1 Introduction
8.2 Comparing two types of risk in the same population 8.1.2 Nonparametric estimation of the CP function 8.1.3 Variance of the CP function estimator 8.1.4 Testing a covariate 8.1.5 Using R 8.1.6 Using SAS
8.2.1 Theoretical background
8.3 Identifiability and testing independence8.2.2 Using R 8.2.3 Discussion 8.4 Parametric modelling
8.4.1 Introduction
8.4.2 Modelling the marginal distribution 8.4.3 Modelling the Weibull distribution 9 Food for thought
Problem 1: Estimation of the probability of the event of interest
Problem 2: Testing a covariate Problem 3: Comparing the event of interest between two groups when the competing risks are different for each group Problem 4: Information needed for sample size calculations Problem 5: The effect of the size of the incidence of competing risks on the coefficient obtained in the model Problem 6: The KLY test and the non-proportionality of hazards Problem 7: The KLY and Wilcoxon tests A: Theoretical background
A.1 Nonparametric maximum likelihood estimation for the survivor function in the discrete case
A.2 Confidence interval for survivor function A.3 The Variance for Gray's test A.4 Derivation of the parameters for the exponential latent failure time model A.5 Likelihood of a mixture of exponentials in the bivariate approach B: Analysing competing risks data using R and SAS
B.1 The R software and cmprsk package
B.1.1 Downloading and installation
B.2 Importing datasets in SASB.1.2 Getting help B.1.3 Operators in R B.1.4 Objects in R B.1.5 The cmprsk package and datasets B.3 Other programs written for R
B.3.1 CIF variance based on the delta method
B.4 SAS macros for competing risk analysis
B.3.2 Pepe–Mori test for the difference between two CIFs B.3.3 Conditional probability and its variance B.3.4 Plotting the conditional probability B.3.5 Testing the conditional probability B.3.6 Calculating the conditional probability of observing the event of interest in a time period knowing that the patient was free of any event at the beginning of the period B.3.7 Comparing two types of risk in the same population B.3.8 Calculating the power
B.4.1 Cumulative incidence and conditional probability
B.4.2 Pepe–Mori test for the comparison of two CIFs B.4.2 Pepe–Mori test for the comparison of two CPs References
Index
|
Learn
Free webinars
NetCourses
Classroom and web training
Organizational training
Video tutorials
Third-party courses
Web resources
Teaching with Stata
© Copyright 1996–2024 StataCorp LLC. All rights reserved.
×
We use cookies to ensure that we give you the best experience on our website—to enhance site navigation, to analyze usage, and to assist in our marketing efforts. By continuing to use our site, you consent to the storing of cookies on your device and agree to delivery of content, including web fonts and JavaScript, from third party web services.
Cookie Settings
Last updated: 16 November 2022
StataCorp LLC (StataCorp) strives to provide our users with exceptional products and services. To do so, we must collect personal information from you. This information is necessary to conduct business with our existing and potential customers. We collect and use this information only where we may legally do so. This policy explains what personal information we collect, how we use it, and what rights you have to that information.
These cookies are essential for our website to function and do not store any personally identifiable information. These cookies cannot be disabled.
This website uses cookies to provide you with a better user experience. A cookie is a small piece of data our website stores on a site visitor's hard drive and accesses each time you visit so we can improve your access to our site, better understand how you use our site, and serve you content that may be of interest to you. For instance, we store a cookie when you log in to our shopping cart so that we can maintain your shopping cart should you not complete checkout. These cookies do not directly store your personal information, but they do support the ability to uniquely identify your internet browser and device.
Please note: Clearing your browser cookies at any time will undo preferences saved here. The option selected here will apply only to the device you are currently using.