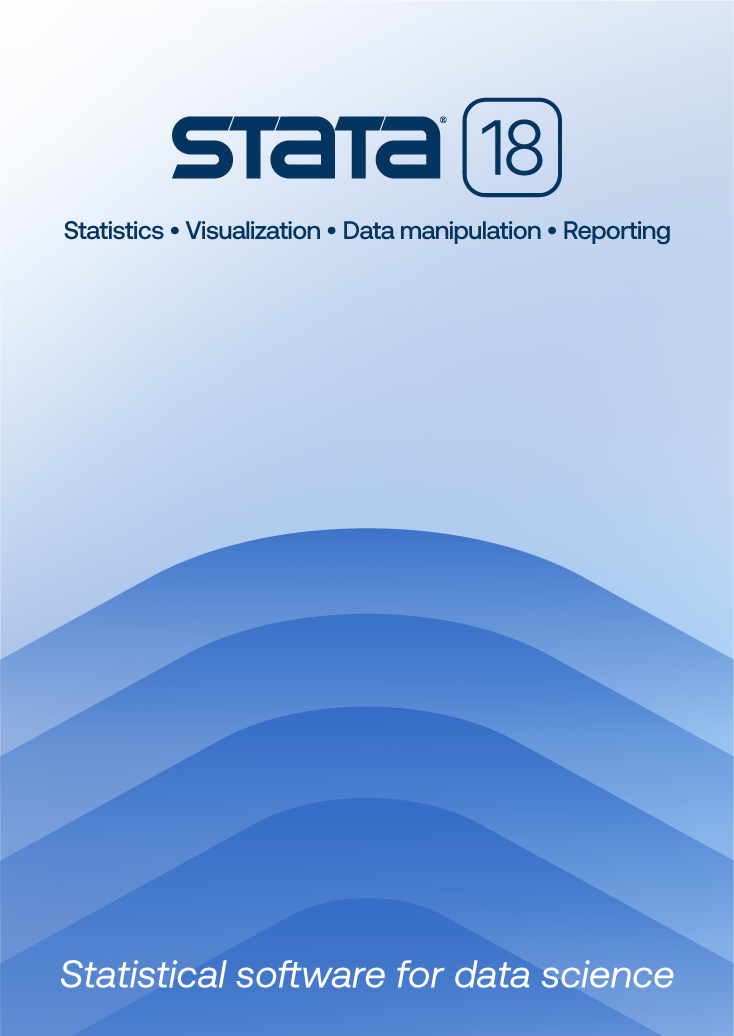
Cluster Randomised Trials, Second Edition |
||||||||||||||||||||||||||||||||
![]() Click to enlarge See the back cover |
![]()
As an Amazon Associate, StataCorp earns a small referral credit from
qualifying purchases made from affiliate links on our site.
eBook not available for this title
eBook not available for this title |
|
||||||||||||||||||||||||||||||
Comment from the Stata technical groupRandomized controlled trials are considered the "gold standard" for evaluating the effectiveness of medical interventions. Sometimes, instead of randomizing individuals, it is preferable to randomize groups. Cluster randomized trials (CRTs) are used when intervention must be applied at a group level, when logistics of randomizing individuals are too difficult, and when it protects from contamination between treatment arms. Cluster Randomised Trials, Second Edition thoroughly describes the aspects of CRT designs and the analysis of data from such trials. After presenting some basic concepts, Hayes and Moulton focus on the clustering aspect of the design. They cover between- and within-cluster variability and correlation, advantages and disadvantages of clustering, how to choose clusters, and other related topics. Middle chapters deal with matching, stratification, randomization procedures, sample-size calculations, and alternative designs. The authors then focus on statistical analyses such as calculations of rate differences and rate ratios, t-tests for means, nonparametric tests of equality between treatment groups, Cox regression, logistic regression, ordinal logistic regression, Poisson regression, GEE, and mixed-effects models. The authors close with miscellaneous topics including the ethical considerations of randomization, establishing stopping rules, and how to report and interpret results. All analyses are performed in Stata, and the data used are freely available, making the analyses easy to reproduce. |
||||||||||||||||||||||||||||||||
Table of contentsView table of contents >> Preface
Authors
Glossary of Notation
Section I Basic Concepts
1. Introduction
1.1 Randomised Trials
1.1.1 Randomising Clusters
1.1.2 Some Case Studies 1.1.3 Overview of Book 2. Variability between Clusters
2.1 Introduction
2.2 The Implications of Between-Cluster Variability: Some Examples 2.3 Measures of Between-Cluster Variability
2.3.1 Introduction
2.4 The Design Effect
2.3.1.1 Binary Outcomes and Proportions
2.3.2 Coefficient of Variation, k2.3.1.2 Event Data and Person-Years Rates 2.3.1.3 Quantitative Outcomes and Means 2.3.3 Intracluster Correlation Coefficient, ρ
2.3.3.1 Quantitative Outcomes
2.3.4 Relationship between k and ρ2.3.3.2 Binary Outcomes 2.3.3.3 Estimation of ρ
2.4.1 Binary Outcomes
2.5 Sources of Within-Cluster Correlation2.4.2 Quantitative Outcomes
2.5.1 Clustering of Population Characteristics
2.5.2 Variations in Response to Intervention 2.5.3 Correlation due to Interaction between Individuals 3. Choosing Whether to Randomise by Cluster
3.1 Introduction
3.2 Rationale for Cluster Randomisation
3.2.1 Type of Intervention
3.3 Using Cluster Randomisation to Capture Indirect Effects of Intervention3.2.2 Logistical Convenience and Acceptability 3.2.3 Contamination
3.3.1 Introduction
3.4 Disadvantages and Limitations of Cluster Randomisation3.3.2 Effects of an Intervention on Infectiousness 3.3.3 Mass Effects of Intervention 3.3.4 Direct, Indirect, Total and Overall Effects
3.4.1 Efficiency
3.4.2 Selection Bias 3.4.3 Imbalances between Study Arms 3.4.4 Generalisability Section II Design Issues
4. Choice of Clusters
4.1 Introduction
4.2 Types of Cluster
4.2.1 Geographical Clusters
4.3 Size of Clusters
4.2.1.1 Communities
4.2.2 Institutional Clusters4.2.1.2 Administrative Units 4.2.1.3 Arbitrary Geographical Zones
4.2.2.1 Schools
4.2.3 Smaller Clusters4.2.2.2 Health Units 4.2.2.3 Workplaces
4.2.3.1 Households and Other Small Groups
4.2.3.2 Network-Defined Clusters 4.2.3.3 Individuals as Clusters
4.3.1 Introduction
4.4 Strategies to Reduce Contamination4.3.2 Statistical Considerations 4.3.3. Logistical Issues 4.3.4 Contamination
4.3.4.1 Contacts between Intervention and Control Clusters
4.3.5 Transmission Zones of Infectious Diseases4.3.4.2 Contacts between Intervention Clusters and the Wider Population 4.3.4.3 Contacts between Control Clusters and the Wider Population 4.3.4.4 Effects of Cluster Size on Contamination
4.4.1 Separation of Clusters
4.5 Levels of Randomisation, Intervention, Data Collection and Inference4.4.2 Buffer Zones 4.4.3 The Fried Egg Design 4.4.4 Assessment of Contamination 5. Matching and Stratification
5.1 Introduction
5.2 Rationale for Matching
5.2.1 Avoiding Imbalance between Treatment Arms
5.3 Disadvantages of Matching5.2.2 Improving Study Power and Precision
5.3.1 Loss of Degrees of Freedom
5.4 Stratification as an Alternative to Matching5.3.2 Drop-Out of Clusters 5.3.3 Limitations in Statistical Inference for Matched Trials
5.3.3.1 Adjustment for Covariates
5.3.3.2 Testing for Variation in Intervention Effect 5.3.3.3 Estimation of Intracluster Correlation Coefficient and Coefficient of Variation 5.5 Choice of Matching Variables
5.5.1 Estimating the Matching Correlation
5.6 Formal Algorithms for Forming Matched Pairs and Strata5.5.2 Matching on Baseline Values of Endpoint of Interest 5.5.3 Matching on Surrogate Variables 5.5.4 Matching on Multiple Variables 5.5.5 Matching on Location 5.7 Choosing Whether to Match or Stratify
5.7.1 Introduction
5.7.2 Trials with a Small Number of Clusters 5.7.3 Trials with a Larger Number of Clusters 6. Randomisation Procedures
6.1 Introduction
6.2 Restricted Randomisation
6.2.1 Basic Principles
6.3 Some Practical Aspects of Randomisation6.2.2 Using Restricted Randomisation to Achieve Overall Balance 6.2.3 Balance Criteria 6.2.4 Validity of Restricted Randomisation 6.2.5 Restricted Randomisation with More than Two Treatment Arms 6.2.6 Alternative Methods for Achieving Balance
6.3.1 Concealment of Allocation
6.3.2 Public Randomisation 7. Sample Size
7.1 Introduction
7.2 Sample Size for Unmatched Trials
7.2.1 Event Rates
7.3 Sample Size for Matched and Stratified Trials7.2.2 Proportions 7.2.3 Means
7.2.3.1 Arbitrary Scales and/or Scales with Negative Values
7.2.4 Sample Size Calculations Based on Intracluster Correlation Coefficient7.2.5 Variable Sample Size per Cluster
7.3.1 Matched Trials
7.4 Estimating the Between-Cluster Coefficient of Variation
7.3.1.1 Event Rates
7.3.2 Stratified Trials7.3.1.2 Proportions 7.3.1.3 Means
7.4.1 Unmatched Trials
7.5 Choice of Sample Size in Each Cluster
7.4.1.1 Event Rates
7.4.2 Matched and Stratified Trials7.4.1.2 Proportions 7.4.1.3 Means
7.4.2.1 Event Rates
7.4.3 Alternative Approach to Estimating Between-Cluster Variation7.4.2.2 Proportions and Means 7.6 Further Issues in Sample Size Calculation
7.6.1 Trials with More than Two Treatment Arms
7.6.2 Trials with Treatment Arms of Unequal Size 7.6.3 Equivalence Trials 7.6.4 Power and Precision 7.6.5 Assumptions about Intervention Effects 7.6.6 Accounting for Baseline Covariates 7.6.7 Sample Size for Examining Interaction 8. Alternative Study Designs
8.1 Introduction
8.2 Design Choices for Treatment Arms
8.2.1 Trials with Several Treatment Arms
8.3 Design Choices for Impact Evaluation8.2.2 Factorial Trials
8.2.2.1 Independent Effects
8.2.3 Crossover Design8.2.2.2 Non-Independent Effects 8.2.4 Stepped Wedge Design
8.2.4.1 Introduction
8.2.5 Parallel Designs with Multiple Baselines8.2.4.2 Efficiency 8.2.4.3 Design Variants 8.2.4.4 Interpretation Difficulties
8.3.1 Introduction
8.3.2 Repeated Cross-Sectional Samples 8.3.3 Cohort Follow-Up Section III Analytical Methods
9. Basic Principles of Analysis
9.1 Introduction
9.2 Experimental and Observational Units 9.3 Parameters of Interest
9.3.1 Event Rates
9.4 Approaches to Analysis9.3.2 Proportions
9.3.2.1 Cluster-Specific Odds Ratio
9.3.3 Means9.3.2.2 Population-Average Odds Ratio 9.3.4 More Complex Parameters
9.4.1 Cluster-Level Analysis
9.5 Baseline Analysis9.4.2 Individual-Level Analysis 10. Analysis Based on Cluster-Level Summaries
10.1 Introduction
10.2 Point Estimates of Intervention Effects
10.2.1 Point Estimates Based on Cluster Summaries
10.3 Statistical Inference Based on the t Distribution10.2.2 Point Estimates Based on Individual Values 10.2.3 Using the Logarithmic Transformation 10.2.4 Case Studies
10.3.1 Unpaired t-Test
10.4 Statistical Inference Based on a Quasi-Likelihood Approach10.3.2 Confidence Intervals Based on Cluster Summaries
10.3.2.1 Rate Difference
10.3.3 Case Studies10.3.2.2 Rate Ratio 10.3.4 Using the Logarithmic Transformation 10.3.5 The Weighted t-Test 10.5 Adjusting for Covariates
10.5.1 Stage 1: Obtaining Covariate-Adjusted Residuals
10.6 Analysis of Ordinal Outcomes
10.5.1.1 Event Rates
10.5.2 Stage 2: Using the Covariate-Adjusted Residuals10.5.1.2 Proportions 10.5.1.3 Means
10.5.2.1 Ratio Measures of Effect
10.5.3 Case Study10.5.2.2 Difference Measures of Effect
10.6.1 Introduction
10.7 Nonparametric Methods10.6.2 Cluster Summaries Approach to the Ordinal Logit Model 10.6.3 Adjusting the Ordinal Logit Model for Covariates 10.6.4 Case Study
10.7.1 Introduction
10.8 Analysing for Effect Modification10.7.2 Rank Sum Test 10.7.3 Permutation Tests 11. Regression Analysis Based on Individual-Level Data
11.1 Introduction
11.2 Random Effects Models
11.2.1 Poisson and Cox Regressions with Random Effects
11.3 Generalised Estimating Equations
11.2.1.1 Poisson Regression with Random Effects
11.2.2 Mixed Effects Linear Regression11.2.1.2 Cox Regression with Random Effects 11.2.3 Logistic Regression with Random Effects
11.3.1 GEE Models for Binary Data
11.4 Choice of Analytical Method11.3.2 GEE for Other Types of Outcome
11.4.1 Small Numbers of Clusters
11.5 Analysing for Effect Modification11.4.2 Larger Numbers of Clusters 11.6 More Complex Analyses
11.6.1 Controlling for Baseline Values
11.6.2 Repeated Measures during Follow-Up 11.6.3 Repeated Episodes 12. Analysis of Trials with More Complex Designs
12.1 Introduction
12.2 Analysis of Pair-Matched Trials
12.2.1 Introduction
12.3 Analysis of Stratified Trials12.2.2 Analysis Based on Cluster-Level Summaries 12.2.3 Adjusting for Covariates 12.2.4 Regression Analysis Based on Individual-Level Data
12.3.1 Introduction
12.4 Analysis of Other Study Designs12.3.2 Analysis Based on Cluster-Level Summaries 12.3.3 Regression Analysis Based on Individual-Level Data
12.4.1 Trials with More than Two Treatment Arms
12.4.2 Factorial Trials 12.4.3 Stepped Wedge Trials Section IV Miscellaneous Topics
13. Ethical Considerations
13.1 Introduction
13.2 General Principles
13.2.1 Beneficence
13.3 Ethical Issues in Group Allocation13.2.2 Equity 13.2.3 Autonomy 13.4 Informed Consent in Cluster Randomised Trials
13.4.1 Consent for Randomisation
13.5 Other Ethical Issues
13.4.1.1 Political Authorities
13.4.2 Consent for Participation13.4.1.2 Village Heads 13.4.1.3 Community Representatives 13.4.1.4 Medical Practitioners
13.5.1 Scientific Validity
13.6 Conclusion13.5.2 Phased Intervention Designs 13.5.3 Trial Monitoring 13.5.4 Non-Participants in Clusters 14. Data Monitoring
14.1 Introduction
14.2 Data Monitoring Committees
14.2.1 Review of DMC Responsibilities
14.3 Interim Analyses14.2.2 When are DMCs Necessary for CRTs?
14.2.2.1 Likelihood of Adverse Events
14.2.3 Monitoring for Adverse Events14.2.2.2 Seriousness or Severity of Outcome Measures 14.2.2.3 Timing of Data Collection 14.2.4 Monitoring for Efficacy 14.2.5 Monitoring Adequacy of Sample Size 14.2.6 Assessing Comparability of Treatment Arms 14.2.7 Approving the Analytical Plan 14.2.8 Presentation of Data to the DMC
14.3.1 Introduction
14.3.2 Timing of Interim Analyses 14.3.3 Stopping Rules
14.3.3.1 Event Rates
14.3.4 Disadvantages of Premature Stopping14.3.3.2 Proportions 14.3.3.3 Means 15. Reporting and Interpretation
15.1 Introduction
15.2 Reporting of Cluster Randomised Trials
15.2.1 Overview
15.3 Interpretation and Generalisability
15.2.1.1 Extended CONSORT Statement
15.2.2 Reporting of Methods15.2.1.2 Publication Bias
15.2.2.1 Rationale for Cluster Randomisation
15.2.3 Reporting of Results15.2.2.2 Description of Clusters and Interventions 15.2.2.3 Sample Size 15.2.2.4 Matching, Stratification and Randomisation 15.2.2.5 Blinding and Allocation Concealment 15.2.2.6 Definition of Primary Endpoints 15.2.2.7 Statistical Methods
15.2.3.1 Flow Diagram
15.2.3.2 Baseline Comparisons 15.2.3.3 Analysis of Endpoints 15.2.3.4 Data Display 15.2.3.5 Subgroup Analyses 15.2.3.6 Contamination 15.2.3.7 Estimates of Between-Cluster Variability
15.3.1 Interpretation
15.3.2 Generalisability 15.3.3 Systematic Reviews References
Appendix
Index
|
Learn
Free webinars
NetCourses
Classroom and web training
Organizational training
Video tutorials
Third-party courses
Web resources
Teaching with Stata
© Copyright 1996–2024 StataCorp LLC. All rights reserved.
×
We use cookies to ensure that we give you the best experience on our website—to enhance site navigation, to analyze usage, and to assist in our marketing efforts. By continuing to use our site, you consent to the storing of cookies on your device and agree to delivery of content, including web fonts and JavaScript, from third party web services.
Cookie Settings
Last updated: 16 November 2022
StataCorp LLC (StataCorp) strives to provide our users with exceptional products and services. To do so, we must collect personal information from you. This information is necessary to conduct business with our existing and potential customers. We collect and use this information only where we may legally do so. This policy explains what personal information we collect, how we use it, and what rights you have to that information.
These cookies are essential for our website to function and do not store any personally identifiable information. These cookies cannot be disabled.
This website uses cookies to provide you with a better user experience. A cookie is a small piece of data our website stores on a site visitor's hard drive and accesses each time you visit so we can improve your access to our site, better understand how you use our site, and serve you content that may be of interest to you. For instance, we store a cookie when you log in to our shopping cart so that we can maintain your shopping cart should you not complete checkout. These cookies do not directly store your personal information, but they do support the ability to uniquely identify your internet browser and device.
Please note: Clearing your browser cookies at any time will undo preferences saved here. The option selected here will apply only to the device you are currently using.