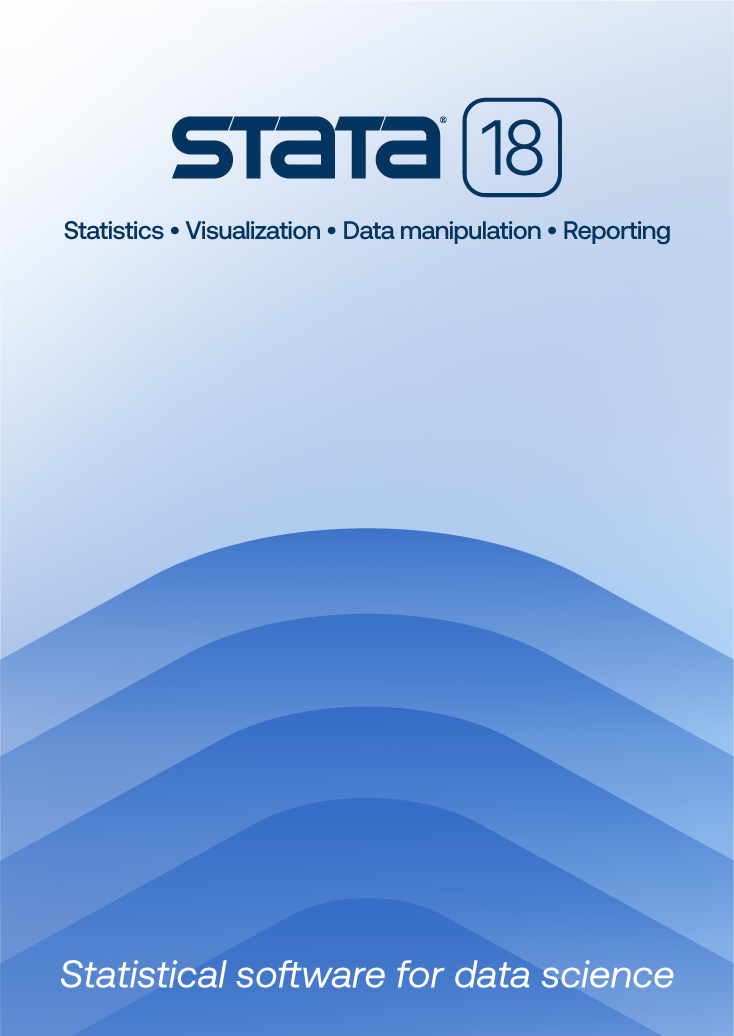
Applied Logistic Regression, Third Edition |
||||||||||||||||||||||||||||||||||||
![]() Click to enlarge See the back cover |
![]()
As an Amazon Associate, StataCorp earns a small referral credit from
qualifying purchases made from affiliate links on our site.
eBook not available for this title
eBook not available for this title |
|
||||||||||||||||||||||||||||||||||
Comment from the Stata technical groupThe third edition of Applied Logistic Regression, by David W. Hosmer, Jr., Stanley Lemeshow, and Rodney X. Sturdivant, is the definitive reference on logistic regression models. The book begins with chapters on fitting and interpreting binary logistic models as well as chapters on assessing model fit and selecting the appropriate covariates and transformations. Despite the modest title, however, the book goes much further. One chapter discusses different sampling schemes, including case–control data, cohort studies, and complex survey data; another chapter is dedicated to matched case–control studies. Later chapters discuss multinomial and ordinal logistic models for multiple-outcome responses and the analysis of correlated longitudinal data using population-average and cluster-specific models. The final chapter broaches advanced topics, including the use of logistic regression in propensity score methods, exact methods, Bayesian estimation, and mediation. Most of the analyses in the book were performed using Stata and can be replicated using Stata and the data from the text. Also noteworthy is the book's use of multinomial fractional polynomial models that can be fit using Stata's mfp command. |
||||||||||||||||||||||||||||||||||||
Table of contentsView table of contents >> Preface to the Third Edition
1 Introduction to the Logistic Regression Model
1.1 Introduction
1.2 Fitting the Logistic Regression Model 1.3 Testing for the Significance of the Coefficients 1.4 Confidence Interval Estimation 1.5 Other Estimation Methods 1.6 Data Sets Used in Examples and Exercises
1.6.1 The ICU Study
Exercises 1.6.2 The Low Birth Weight Study 1.6.3 The Global Longitudinal Study of Osteoporosis in Women 1.6.4 The Adolescent Placement Study 1.6.5 The Burn Injury Study 1.6.6 The Myopia Study 1.6.7 The NHANES Study 1.6.8 The Polypharmacy Study 2 The Multiple Logistic Regression Model
2.1 Introduction
2.2 The Multiple Logistic Regression Model 2.3 Fitting the Multiple Logistic Regression Model 2.4 Testing for the Significance of the Model 2.5 Confidence Interval Estimation 2.6 Other Estimation Methods Exercises 3 Interpretation of the Fitted Logistic Regression model
3.1 Introduction
3.2 Dichotomous Independent Variable 3.3 Polychotomous Independent Variable 3.4 Continuous Independent Variable 3.5 Multivariable Models 3.6 Presentation and Interpretation of the Fitted Values 3.7 A Comparison of Logistic Regression and Stratified Analysis for 2 × 2 Tables Exercises 4 Model-Building Strategies and Methods for Logistic Regression
4.1 Introduction
4.2 Purposeful Selection of Covariates
4.2.1 Methods to Examine the Scale of a Continuous Covariate in the Logit
4.3 Other Methods for Selecting Covariates 4.2.2 Examples of Purposeful Selection
4.3.1 Stepwise Selection of Covariates
4.4 Numerical Problems 4.3.2 Best Subsets Logistic Regression 4.3.3 Selecting Covariates and Checking their Scale Using Multivariable Fractional Polynomials Exercises 5 Assessing the Fit of the Model
5.1 Introduction
5.2 Summary Measures of Goodness of Fit
5.2.1 Pearson Chi-Square Statistic, Deviance, and Sum-of-Squares
5.3 Logistic Regression Diagnostics 5.2.2 The Hosmer–Lemeshow Tests 5.2.3 Classification Tables 5.2.4 Area Under the Receiver Operating Characteristic Curve 5.2.5 Other Summary Measures 5.4 Assessment of Fit via External Validation 5.5 Interpretation and Presentation of the Results from a Fitted Logistic Regression Model Exercises 6 Application of Logistic Regression with Different Sampling Models
6.1 Introduction
6.2 Cohort Studies 6.3 Case-Control Studies 6.4 Fitting Logistic Regression Models to Data from Complex Sample Surveys Exercises 7 Logistic Regression for Matched Case-Control Studies
7.1 Introduction
7.2 Methods For Assessment of Fit in a 1–M Matched Study 7.3 An Example Using the Logistic Regression Model in a 1–1 Matched Study 7.4 An Example Using the Logistic Regression Model in a 1–M Matched Study Exercises 8 Logistic Regression Models for Multinomial and Ordinal Outcomes
8.1 The Multinomial Logistic Regression Model
8.1.1 Introduction to the Model and Estimation of Model Parameters
8.2 Ordinal Logistic Regression Models 8.1.2 Interpreting and Assessing the Significance of the Estimated Coefficients 8.1.3 Model-Building Strategies for Multinomial Logistic Regression 8.1.4 Assessment of Fit and Diagnostic Statistics for the Multinomial Logistic Regression Model
8.2.1 Introduction to the Models, Methods for Fitting, and Interpretation of Model Parameters
Exercises 8.2.2 Model Building Strategies for Ordinal Logistic Regression Models 9 Logistic Regression Models for the Analysis of Correlated Data
9.1 Introduction
9.2 Logistic Regression Models for the Analysis of Correlated Data 9.3 Estimation Methods for Correlated Data Logistic Regression Models 9.4 Interpretation of Coefficients from Logistic Regression Models for the Analysis of Correlated Data
9.4.1 Population Average Model
9.5 An Example of Logistic Regression Modeling with Correlated Data 9.4.2 Cluster-Specific Model 9.4.3 Alternative Estimation Methods for the Cluster-Specific Model 9.4.4 Comparison of Population Average and Cluster-Specific Model
9.5.1 Choice of Model for Correlated Data Analysis
9.6 Assessment of Model Fit 9.5.2 Population Average Model 9.5.3 Cluster-Specific Model 9.5.4 Additional Points to Consider when Fitting Logistic Regression Models to Correlated Data
9.6.1 Assessment of Population Average Model Fit
Exercises 9.6.2 Assessment of Cluster-Specific Model Fit 9.6.3 Conclusions 10 Special Topics
10.1 Introduction
10.2 Application of Propensity Score Methods in Logistics Regression Modeling 10.3 Exact Methods for Logistic Regression Models 10.4 Missing Data 10.5 Sample Size Issues when Fitting Logistic Regression Models 10.6 Bayesian Methods for Logistic Regression
10.6.1 The Bayesian Logistic Regression Model
10.7 Other Link Functions for Binary Regression Models 10.6.2 MCMC Simulation 10.6.3 An Example of a Bayesian Analysis and Its Interpretation 10.8 Mediation
10.8.1 Distinguishing Mediators from Confounders
10.9 More About Statistical Interaction 10.8.2 Implications for the Interpretation of an Adjusted Logistic Regression Coefficient 10.8.3 Why Adjust for a Mediator 10.8.4 Using Logistic Regression to Assess Mediation: Assumptions
10.9.1 Additive versus Multiplicative Scale–Risk Difference versus Odds Ratios
Exercises 10.9.2 Estimating and Testing Additive Interaction References
Index
|
Learn
Free webinars
NetCourses
Classroom and web training
Organizational training
Video tutorials
Third-party courses
Web resources
Teaching with Stata
© Copyright 1996–2025 StataCorp LLC. All rights reserved.
×
We use cookies to ensure that we give you the best experience on our website—to enhance site navigation, to analyze usage, and to assist in our marketing efforts. By continuing to use our site, you consent to the storing of cookies on your device and agree to delivery of content, including web fonts and JavaScript, from third party web services.
Cookie Settings
Last updated: 16 November 2022
StataCorp LLC (StataCorp) strives to provide our users with exceptional products and services. To do so, we must collect personal information from you. This information is necessary to conduct business with our existing and potential customers. We collect and use this information only where we may legally do so. This policy explains what personal information we collect, how we use it, and what rights you have to that information.
These cookies are essential for our website to function and do not store any personally identifiable information. These cookies cannot be disabled.
This website uses cookies to provide you with a better user experience. A cookie is a small piece of data our website stores on a site visitor's hard drive and accesses each time you visit so we can improve your access to our site, better understand how you use our site, and serve you content that may be of interest to you. For instance, we store a cookie when you log in to our shopping cart so that we can maintain your shopping cart should you not complete checkout. These cookies do not directly store your personal information, but they do support the ability to uniquely identify your internet browser and device.
Please note: Clearing your browser cookies at any time will undo preferences saved here. The option selected here will apply only to the device you are currently using.