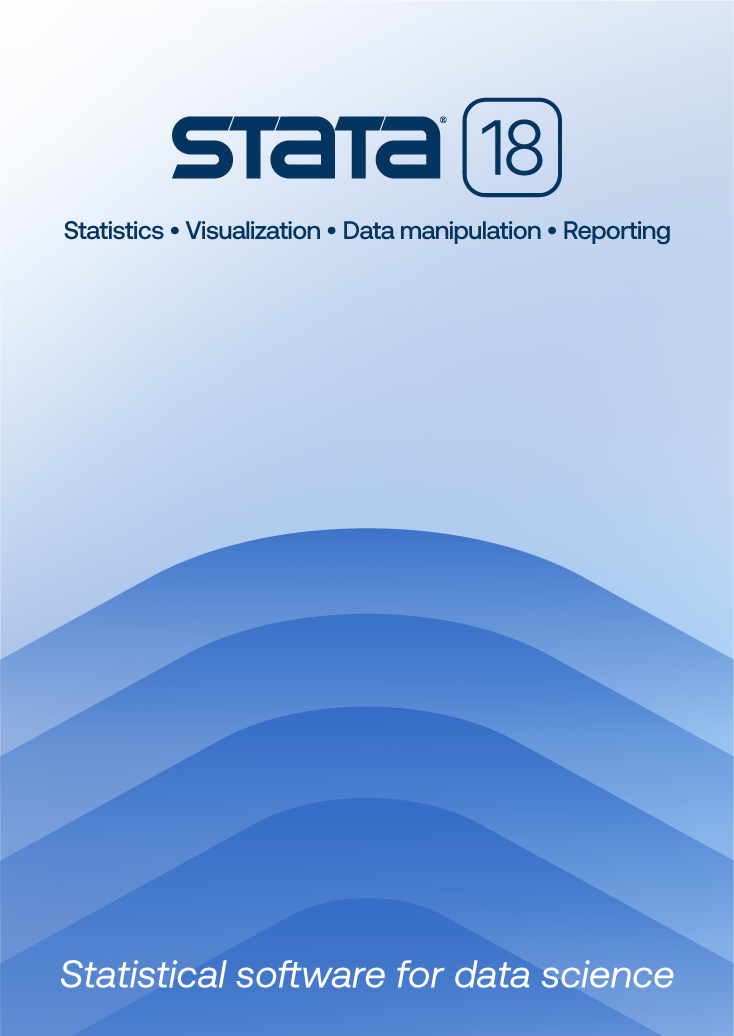
Applied Econometrics, Fourth Edition |
||||||||||||||||||||||||||||||||
![]() Click to enlarge See the back cover |
![]()
As an Amazon Associate, StataCorp earns a small referral credit from
qualifying purchases made from affiliate links on our site.
eBook not available for this title
eBook not available for this title |
|
||||||||||||||||||||||||||||||
Comment from the Stata technical groupApplied Econometrics, Fourth Edition, by Asteriou and Hall, provides a great introductory-level discussion of econometrics. All the topics emphasize the intuition and interpretation of results. The mathematical foundations are presented in the text, but familiarity with math is not a prerequesite to understand the concepts. The book talks about linear regression and other tools for cross-sectional data and then goes into time-series and panel-data analysis. All the chapters show the reader how to use statistical software to obtain the results using real data. This helps the authors present the motivations behind the different econometric tools. |
||||||||||||||||||||||||||||||||
Table of contentsView table of contents >> List of Figures
List of Tables
Preface
Acknowledgements
Part I Statistical Background and Basic Data Handling
1 Fundamental Concepts
Introduction
A simple example A statistical framework Properties of the sampling distribution of the mean Hypothesis testing and the central limit theorem
Central limit theorem
Conclusion2 The Structure of Economic Data and Basic Data Handling
Learning objectives
The structure of economic data
Cross-sectional data
Basic data handlingTime series data Panel data
Looking at raw data
QuestionsGraphical analysis Summary statistics Part II The Classical Linear Regression Model
3 Simple Regression
Learning objectives
Introduction to regression: the classical linear regression model (CLRM)
Why do we do regressions?
The Ordinary Least Squares (OLS) method of estimationThe classical linear regression model
Alternative expressions for \(\hat{\beta}\)
The assumptions of the CLRM
General
Properties of the OLS estimatorsThe assumptions Violations of the assumptions
Linearity
The overall goodness of fitUnbiasedness Efficiency and BLUEness Consistency
Problems associated with R2
Hypothesis testing and confidence intervals
Testing the significance of the OLS coefficients
How to estimate a simple regression in EViews and StataConfidence intervals
Simple regression in EViews
Presentation of regression resultsSimple regression in Stata Reading the Stata simple regression results output Reading the EViews simple regression results output Economic theory applicants
Application 1: the demand function
Computer example: the Keynesian consumption functionApplication 2: the production function Application 3: Okun's law Application 4: the Keynesian consumption function
Solution
Questions and exercises4 Multiple Regression
Learning objectives
Introduction Derivation of multiple regression coefficients
The three-variable model
Properties of multiple regression model OLS estimatorsThe k-variables case Derivation of the coefficients with matrix algebra The structure of the X'X and X'Y matrices The assumptions of the multiple regression model The variance–covariance matrix of the errors
Linearity
R2 and adjusted R2Unbiasedness Consistency BLUEness General criteria for model selection Multiple regression estimation in EViews and Stata
Multiple regression in EViews
Hypothesis testingMultiple regression in Stata Reading the EViews multiple regression results output
Testing individual coefficients
The F-form of the likelihood ratio testTesting linear restrictions Testing the joint significance of the Xs
F-test for overall significance in EViews
Adding or deleting explanatory variables
Omitted and redundant variables test in EViews
The t-test (a special case of the Wald procedure)How to perform the Wald test in EViews The Lagrange multiplier (LM) test
The LM test in EViews
Computer example: Wald, omitted and redundant variables tests
A Wald test of coefficients restrictions
Financial econometrics application: the capital asset pricing model in actionA redundant variable test An omitted variable test Computer example: commands for Stata
A few theoretical remarks regarding the CAPM
Questions and exercisesThe empirical application of the CAPM EViews programming and the CAPM application Advanced EViews programming and the CAPM application Part III Violating the Assumptions of the CLRM
5 Multicollinearity
Learning objectives
Introduction Perfect multicollinearity Consequences of perfect multicollinearity Imperfect multicollinearity Consequences of imperfect multicollinearity Detecting problematic multicollinearity
Simple correlation coefficient
Computer examplesR2 from auxiliary regressions
Example 1: induced multicollinearity
Questions and exercisesExample 2: with the use of real economic data
Questions
6 Heteroskedasticity
Learning objectives
Introduction: what is heteroskedasticity? Consequences of heteroskedasticity for OLS estimators
A general approach
Detecting heteroskedasticityA mathematical approach
The informal way
Criticism of the LM testsThe Breusch–Pagan LM test The Glesjer LM test The Harvey–Godfrey LM test The Park LM test
The Goldfeld–Quandt test
Computer example: heteroskedasticity testsWhite's test
The Breusch–Pagan test
Resolving heteroskedasticityThe Glesjer test The Harvey–Godfrey test The Park test The Goldfeld–Quandt test White's test Commands for the computer example in Stata Engle's ARCH test Computer example of the ARCH-LM test
Generalized (or weighted) least squares
Computer example: resolving heteroskedasticityQuestions and exercises 7 Autocorrelation
Learning objectives
Introduction: what is autocorrelation? What causes autocorrelation? First- and higher-order autocorrelation Consequences of autocorrelation for the OLS estimators
A general approach
Detecting autocorrelationA more mathematical approach
The graphical method
Resolving autocorrelationExample: detecting autocorrelation using the graphical method The Durbin–Watson test Computer example of the DW test The Breusch–Godfrey LM test for serial correlation Computer example of the Breusch–Godfrey test Durbin's h-test in the presence of lagged dependent variables Computer example of Durbin's h-test
When ρ is known
Questions and exercisesComputer example of the generalized differencing approach When ρ is unknown Computer example of the iterative procedure Resolving autocorrelation in Stata Appendix 8 Misspecification: Wrong Regressors, Measurement Errors and Wrong Functional Forms
Learning objectives
Questions and exercisesIntroduction Omitting influential or including non-influential explanatory variables
Consequences of omitting influential variables
Various functional formsIncluding a non-influential variable Omission and inclusion of relevant and irrelevant variables at the same time The plug-in solution in the omitted variable bias
Introduction
Measurement errorsLinear-log functional form Reciprocal functional form Polynomial functional form Functional form including interaction terms Log-linear functional form The double-log functional form The Box–Cox transformation
Measurement error in the dependent variable
Tests for misspecificationMeasurement error in the explanatory variable
Normality of residuals
Computer example: the Box–Cox transformation in EViewsThe Ramsey RESET test for general misspecification Tests for non-nested models Approaches in choosing an appropriate model The traditional view: average economic regression The Hendry 'general to specific approach' Part IV Topics in Econometrics
9 Dummy Variables
Learning objectives
Introduction: the nature of qualitative information The use of dummy variables
Constant dummy variables
Computer example of the use of dummy variablesSlope dummy variables The combined effect of intercept and slope dummies
Using a constant dummy
Special cases of the use of dummy variablesUsing a slope dummy Using both dummies together
Using dummy variables with multiple categories
Computer example of dummy variables with multiple categoriesUsing more than one dummy variable Using seasonal dummy variables Financial econometrics application: the January effect in emerging stock markets Tests for structural stability
The dummy variable approach
Financial econometrics application: the day-of-the-week effect in actionThe Chow test for structural stability How to create daily dummies in Stata Questions and exercises 10 Dynamic Econometric Models
Learning objectives
Introduction Distributed lag models
The Koyck transformation
Autoregressive modelsThe Almon transformation Other models of lag structures
The partial adjustment model
ExercisesComputer example of the partial adjustment model The adaptive expectations model Tests of autocorrelation in autoregressive models 11 Simultaneous Equation Models
Learning objectives
Introduction: basic definitions Consequences of ignoring simultaneity The identification problem
Basic definitions
Estimation of simultaneous equation modelsConditions for identification Example of the identification procedure A second example: the macroeconomic model of a closed economy
Estimation of an exactly identified equation: the ILS method
Computer example: the IS–LM modelEstimation of an over-identified equation: the TSLS method
Estimation of simultaneous equations in Stata
Exercises12 Limited Dependent Variable Regression Models
Learning objectives
The Tobit modelIntroduction The linear probability model Problems with the linear probability model
\(\hat{D}\)i is not bounded by the (0,1) range
The logit modelNon-normality and heteroskedasticity of the disturbances The coefficient of determination as a measure of overall fit
A general approach
The probit modelInterpretation of the estimates in logit models Goodness of fit A more mathematical approach A general approach A more mathematical approach Multinomial and ordered logit and probit models Multinomial logit and probit models Ordered logit and probit models Computer example: probit and logit models in EViews and Stata
Logit and probit models in EViews
ExercisesLogit and probit models in Stata Part V Time Series Econometrics
13 ARIMA Models and the Box–Jenkins Methodology
Learning objectives
An introduction to time series econometrics ARIMA models Stationarity Autoregressive time series models
The AR(1) model
Moving average modelsThe AR(p) model Properties of the AR models
The MA(1) model
ARMA modelsThe MA(q) model Invertibility in MA models Properties of the MA models Integrated processes and the ARIMA models
An integrated series
Box–Jenkins model selectionExample of an ARIMA model
Identification
Computer example: the Box–Jenkins approachEstimation Diagnostic checking The Box–Jenkins approach step by step
The Box–Jenkins approach in EViews
Questions and exercisesThe Box–Jenkins approach in Stata 14 Modelling the Variance: ARCH–GARCH Models
Learning objectives
Introduction The ARCH model
The ARCH(1) model
The GARCH modelThe ARCH(q) model Testing for ARCH effects Estimation of ARCH models by iteration Estimating ARCH models in EViews A more mathematical approach
The GARCH(p,q) model
Alternative specificationsThe GARCH(1,1) model as an infinite ARCH process Estimating GARCH models in EViews
The GARCH in mean, or GARCH-M, model
Application: a GARCH model of UK GDP and the effect of socio-political instabilityEstimating GARCH-M models in EViews The threshold GARCH (TGARCH) model Estimating TGARCH models in EViews The exponential GARCH (EGARCH) model Estimating EGARCH models in EViews Adding explanatory variables in the mean equation Adding explanatory variables in the variance equation Estimating ARCH/GARCH-type models in Stata Advanced EViews programming for the estimation of GARCH-type models Questions and exercises 15 Vector Autoregressive (VAR) Models and Causality Tests
Learning objectives
Vector autoregressive (VAR) models
The VAR model
Causality testsPros and cons of the VAR models
The Granger causality test
Financial econometrics application: financial development and economic growth – what is the causal relationship?The Sims causality test Estimating VAR models and causality tests in EViews and Stata
Estimating VAR models in EViews
ExercisesEstimating VAR models in Stata 16 Non-Stationarity and Unit-Root Tests
Learning objectives
Introduction Unit roots and spurious regressions
What is a unit root?
Testing for unit rootsSpurious regressions Explanation of the spurious regression problem
Testing for the order of integration
Unit-root tests in EViews and StataThe simple Dickey–Fuller (DF) test for unit roots The augmented Dickey–Fuller (ADF) test for unit roots The Phillips–Perron (PP) test
Performing unit-root tests in EViews
Application: unit-root tests on various macroeconomic variablesPerforming unit-root tests in Stata Financial econometrics application: unit-root tests for the financial development and economic growth case Questions and exercises 17 Cointegration and Error-Correction Models
Learning objectives
Introduction: what is cointegration?
Cointegration: a general approach
Cointegration and the error-correction mechanism (ECM): a general approachCointegration: a more mathematical approach
The problem
Cointegration and the error-correction mechanism: a more mathematical approachCointegration (again) The error-correction model (ECM) Advantages of the ECM
A simple model for only one lagged term of X and Y
Testing for cointegrationA more general model for large numbers of lagged terms
Cointegration in single equations: the Engle–Granger approach
Financial econometrics application: cointegration tests for the financial development and economic growth caseDrawbacks of the EG approach The EG approach in EViews and Stata Cointegration in multiple equations and the Johansen approach Advantages of the multiple-equation approach The Johansen approach (again) The steps of the Johansen approach in practice The Johansen approach in EViews and Stata
Monetization ratio
Questions and exercisesTurnover ratio Claims and currency ratios A model with more than one financial development proxy variable 18 Identification in Standard and Cointegrated Systems
Learning objectives
Introduction Identification in the standard case The order condition The rank condition
Identification in cointegrated systems
ConclusionsA worked example Computer example of identification Questions and exercises 19 Solving Models
Learning objectives
Introduction Solution procedures Model add factors Simulation and impulse responses Stochastic model analysis Setting up a model in EViews Conclusion Exercises 20 Time-Varying Coefficient Models: A New Way of Estimating Bias-Free Parameters
Learning objectives
Introduction TVC estimation
Theorem 1
Coefficient drivers
Assumption 1 (auxiliary information)
Choosing coefficient driversAssumption 2
First requirement: selecting the complete driver set
Financial econometrics application: rating agencies' decisions and the sovereign bond spread between Greece and GermanySecond requirement: splitting the driver set Conclusion Questions and exercises Part VI Panel Data Econometrics
21 Traditional Panel Data Models
Learning objectives
Introduction: the advantages of panel data The linear panel data model Different methods of estimation
The common constant method
Computer examples with panel dataThe fixed effects method The random effects method The Hausman test
Inserting panel data in EViews
Estimating a panel data regression in EViews The Hausman test in EViews Estimating a panel data regression in Stata The Hausman test in Stata 22 Dynamic Heterogeneous Panels
Learning objectives
Introduction Bias in dynamic panels
Bias in the simple OLS estimator
Solutions to the bias problem (caused by the dynamic nature of the panel)Bias in the fixed effects model Bias in the random effects model Bias of heterogeneous slope parameters Solutions to heterogeneity bias: alternative methods of estimation
The mean group (MG) estimator
Application: the effects of uncertainty in economic growth and investmentThe pooled mean group (PMG) estimator
Evidence from traditional panel data estimation
Mean group and pooled mean group estimates 23 Non-Stationary Panels
Learning objectives
Introduction Panel unit-root tests
The Levin and Lin (LL) test
Panel cointegration testsThe Im, Pesaran and Shin (IPS) test The Maddala and Wu (MW) test Computer examples of panel unit-root tests
Introduction
Computer examples of panel cointegration testsThe Kao test The McCoskey and Kao test The Pedroni tests The Larsson et al. test Part VII Using Econometric Software
24 Practicalities of Using EViews and Stata
About EViews
Starting up with EViews
About StataCreating a workfile and importing data Copying and pasting data Verifying and saving the data Examining the data Commands, operators and functions
Starting up with Stata
Cross-sectional and time series data in StataThe Stata menu and buttons Creating a file when importing data Copying/pasting data
First way – time series data with no time variable
Saving dataSecond way – time series data with time variable Time series – daily frequency Time series – monthly frequency All frequencies
Basic commands in Stata
Understanding command syntax in Stata Appendix: Statistical Tables
Bibliography
Index
|
Learn
Free webinars
NetCourses
Classroom and web training
Organizational training
Video tutorials
Third-party courses
Web resources
Teaching with Stata
© Copyright 1996–2024 StataCorp LLC. All rights reserved.
×
We use cookies to ensure that we give you the best experience on our website—to enhance site navigation, to analyze usage, and to assist in our marketing efforts. By continuing to use our site, you consent to the storing of cookies on your device and agree to delivery of content, including web fonts and JavaScript, from third party web services.
Cookie Settings
Last updated: 16 November 2022
StataCorp LLC (StataCorp) strives to provide our users with exceptional products and services. To do so, we must collect personal information from you. This information is necessary to conduct business with our existing and potential customers. We collect and use this information only where we may legally do so. This policy explains what personal information we collect, how we use it, and what rights you have to that information.
These cookies are essential for our website to function and do not store any personally identifiable information. These cookies cannot be disabled.
This website uses cookies to provide you with a better user experience. A cookie is a small piece of data our website stores on a site visitor's hard drive and accesses each time you visit so we can improve your access to our site, better understand how you use our site, and serve you content that may be of interest to you. For instance, we store a cookie when you log in to our shopping cart so that we can maintain your shopping cart should you not complete checkout. These cookies do not directly store your personal information, but they do support the ability to uniquely identify your internet browser and device.
Please note: Clearing your browser cookies at any time will undo preferences saved here. The option selected here will apply only to the device you are currently using.