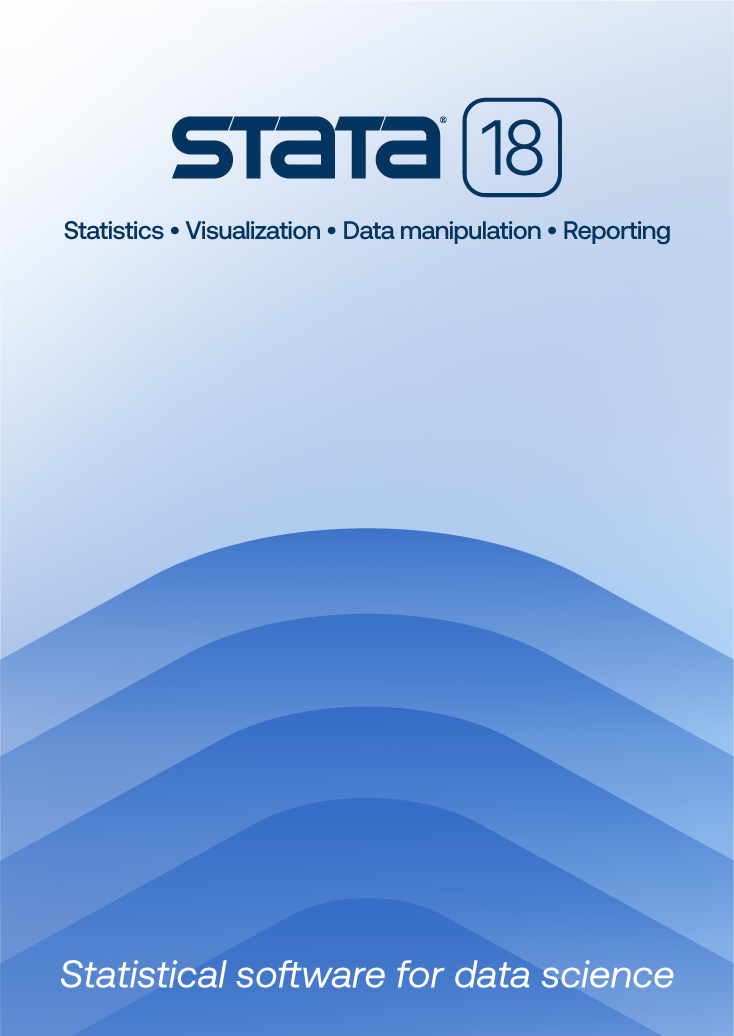
Applications of Regression Models in Epidemiology |
||||||||||||||||||||||||||||||||||||
![]() Click to enlarge See the back cover |
![]()
As an Amazon Associate, StataCorp earns a small referral credit from
qualifying purchases made from affiliate links on our site.
eBook not available for this title
eBook not available for this title |
|
||||||||||||||||||||||||||||||||||
Comment from the Stata technical groupApplications of Regression Models in Epidemiology, by Suárez et al., analyzes the main statistical tools to analyze data from epidemiologic designs, with emphasis in the analytical foundations. The book covers, among other topics, linear, logistic, and Poisson regression, generalized linear models, and hypothesis testing and shows examples where these techniques are applied using Stata. This text is suitable for a graduate level course in epidemiology or biostatistics; each chapter contains an applied exercise where the reader can implement the tools just covered on a practical problem and bibliographical references for those interested in exploring the topics more in depth. The last chapter implements the solutions to all the exercises in the book using Stata, as well as using other packages. |
||||||||||||||||||||||||||||||||||||
Table of contentsView table of contents >> Preface
Acknowledgments
About the Authors
1 Basic Concepts for Statistical Modeling
1.1 Introduction
1.2 Parameter Versus Statistic 1.3 Probability Definition 1.4 Conditional Probability 1.5 Concepts of Prevalence and Incidence 1.6 Random Variables 1.7 Probability Distributions 1.8 Centrality and Dispersion Parameters of a Random Variable 1.9 Independence and Dependence of Random Variables 1.10 Special Probability Distributions
1.10.1 Binomial Distribution
1.11 Hypothesis Testing1.10.2 Poisson Distribution 1.10.3 Normal Distribution 1.12 Confidence Intervals 1.13 Clinical Significance Versus Statistical Significance 1.14 Data Management
1.14.1 Study Design
1.15 Concept of Causality1.14.2 Data Collection 1.14.3 Data Entry 1.14.4 Data Screening 1.14.5 What to Do When Detecting a Data Issue 1.14.6 Impact of Data Issues and How to Proceed References 2 Introduction to Simple Linear Regression Models
2.1 Introduction
2.2 Specific Objectives 2.3 Model Definition 2.4 Model Assumptions 2.5 Graphic Representation 2.6 Geometry of the Simple Regression Model 2.7 Estimation of Parameters 2.8 Variance of Estimators 2.9 Hypothesis Testing About the Slope of the Regression Line
2.9.1 Using the Student's t-Distribution
2.10 Coefficient of Determination R22.9.2 Using ANOVA 2.11 Pearson Correlation Coefficient 2.12 Estimation of Regression Line Values and Prediction
2.12.1 Confidence Interval for the Regression Line
2.13 Example2.12.2 Prediction Interval of Actual Values of the Response 2.14 Predictions
2.14.1 Predictions with the Database Used by the Model
2.15 Conclusions2.14.2 Predictions with Data Not Used to Create the Model 2.14.3 Residual Analysis Practice Exercise References 3 Matrix Representation of the Linear Regression Model
3.1 Introduction
3.2 Specific Objectives 3.3 Definition
3.3.1 Matrix
3.4 Matrix Representation of a SLRM3.5 Matrix Arithmetic
3.5.1 Addition and Subtraction of Matrices
3.6 Matrix Multiplication3.7 Special Matrices 3.8 Linear Dependence 3.9 Rank of a Matrix 3.10 Inverse Matrix [A–1] 3.11 Application of an Inverse Matrix in a SLRM 3.12 Estimation of β Parameters in a SLRM 3.13 Multiple Linear Regression Model (MLRM) 3.14 Interpretation of the Coefficients in a MLRM 3.15 ANOVA in a MLRM 3.16 Using Indicator Variables (Dummy Variables) 3.17 Polynomial Regression Models 3.18 Centering 3.19 Multicollinearity 3.20 Interaction Terms 3.21 Conclusion Practice Exercise References 4 Evaluation of Partial Tests of Hypotheses in a MLRM
4.1 Introduction
4.2 Specific Objectives 4.3 Definition of Partial Hypothesis 4.4 Evaluation Process of Partial Hypotheses 4.5 Special Cases 4.6 Examples 4.7 Conclusion Practice Exercise References 5 Selection of Variables in a Multiple Linear Regression Model
5.1 Introduction
5.2 Specific Objectives 5.3 Selection of Variables According to the Study Objectives 5.4 Criteria for Selecting the Best Regression Model
5.4.1 Coefficient of Determination, R2
5.5 Stepwise Method in Regression5.4.2 Adjusted Coefficient of Determination, R2A 5.4.3 Mean Square Error (MSE) 5.4.4 Mallows's Cp 5.4.5 Akaike Information Criterion 5.4.6 Bayesian Information Criterion 5.4.7 All Possible Models
5.5.1 Forward Selection
5.6 Limitations of Stepwise Methods5.5.2 Backward Elimination 5.5.3 Stepwise Selection 5.7 Conclusion Practice Exercise References 6 Correlation Analysis
6.1 Introduction
6.2 Specific Objectives 6.3 Main Correlation Coefficients Based on SLRM
6.3.1 Pearson Correlation Coefficient ρ
6.4 Major Correlation Coefficients Based on MLRM6.3.2 Relationship Between r and β1
6.4.1 Pearson Correlation Coefficient of Zero Order
6.5 Partial Correlation Coefficient6.4.2 Multiple Correlation Coefficient
6.5.1 Partial Correlation Coefficient of the First Order
6.6 Significance Tests6.5.2 Partial Correlation Coefficient of the Second Order 6.5.3 Semipartial Correlation Coefficient 6.7 Suggested Correlations 6.8 Example 6.9 Conclusion Practice Exercise References 7 Strategies for Assessing the Adequacy of the Linear Regression Model
7.1 Introduction
7.2 Specific Objectives 7.3 Residual Definition 7.4 Initial Exploration 7.5 Initial Considerations 7.6 Standardized Residual 7.7 Jackknife Residuals (R-Student Residuals) 7.8 Normality of the Errors 7.9 Correlation of Errors 7.10 Criteria for Detecting Outliers, Leverage, and Influential Points 7.11 Leverage Values 7.12 Cook's Distance 7.13 COV RATIO 7.14 DFBETAS 7.15 DFFITS 7.16 Summary of the Results 7.17 Multicollinearity 7.18 Transformation of Variables 7.19 Conclusion Practice Exercise References 8 Weighted Least-Squares Linear Regression
8.1 Introduction
8.2 Specific Objectives 8.3 Regression Model with Transformation into the Original Scale of Y 8.4 Matrix Notation of the Weighted Linear Regression Model 8.5 Application of the WLS Model with Unequal Number of Subjects
8.5.1 Design without Intercept
8.6 Applications of the WLS Model When Variance Increases8.5.2 Model with Intercept and Weighting Factor
8.6.1 First Alternative
8.7 Conclusions8.6.2 Second Alternative Practice Exercise References 9 Generalized Linear Models
9.1 Introduction
9.2 Specific Objectives 9.3 Exponential Family of Probability Distributions
9.3.1 Binomial Distribution
9.4 Exponential Family of Probability Distributions with Dispersion9.3.2 Poisson Distribution 9.5 Mean and Variance in EF and EDF 9.6 Definition of a Generalized Linear Model 9.7 Estimation Methods 9.8 Deviance Calculation 9.9 Hypothesis Evaluation 9.10 Analysis of Residuals 9.11 Model Selection 9.12 Bayesian Models 9.13 Conclusions References 10 Poisson Regression Models for Cohort Studies
10.1 Introduction
10.2 Specific Objectives 10.3 Incidence Measures
10.3.1 Incidence Density
10.4 Confounding Variable10.3.2 Cumulative Incidence 10.5 Stratified Analysis 10.6 Poisson Regression Model 10.7 Definition of Adjusted Relative Risk 10.8 Interaction Assessment 10.9 Relative Risk Estimation 10.10 Implementation of the Poisson Regression Model 10.11 Conclusion Practice Exercise References 11 Logistic Regression in Case–Control Studies
11.1 Introduction
11.2 Specific Objectives 11.3 Graphical Representation 11.4 Definition of the Odds Ratio 11.5 Confounding Assessment 11.6 Effect Modification 11.7 Stratified Analysis 11.8 Unconditional Logistic Regression Model 11.9 Types of Logistic Regression Models
11.9.1 Binary Case
11.10 Computing the ORcrude11.9.2 Binomial Case 11.11 Computing the Adjusted OR 11.12 Inference on OR 11.13 Example of the Application of ULR Model: Binomial Case 11.14 Conditional Logistic Regression Model 11.15 Conclusions Practice Exercise References 12 Regression Models in a Cross-Sectional Study
12.1 Introduction
12.2 Specific Objectives 12.3 Prevalence Estimation Using the Normal Approach 12.4 Definition of the Magnitude of the Association 12.5 POR Estimation
12.5.1 Woolf's Method
12.6 Prevalence Ratio12.5.2 Exact Method 12.7 Stratified Analysis 12.8 Logistic Regression Model
12.8.1 Modeling Prevalence Odds Ratio
12.9 Conclusions12.8.2 Modeling Prevalence Ratio Practice Exercise References 13 Solutions to Practice Exercises
Chapter 2 Practice Exercise
Chapter 3 Practice Exercise Chapter 4 Practice Exercise Chapter 5 Practice Exercise Chapter 6 Practice Exercise Chapter 7 Practice Exercise Chapter 8 Practice Exercise Chapter 10 Practice Exercise Chapter 11 Practice Exercise Chapter 12 Practice Exercise Index
|
Learn
Free webinars
NetCourses
Classroom and web training
Organizational training
Video tutorials
Third-party courses
Web resources
Teaching with Stata
© Copyright 1996–2025 StataCorp LLC. All rights reserved.
×
We use cookies to ensure that we give you the best experience on our website—to enhance site navigation, to analyze usage, and to assist in our marketing efforts. By continuing to use our site, you consent to the storing of cookies on your device and agree to delivery of content, including web fonts and JavaScript, from third party web services.
Cookie Settings
Last updated: 16 November 2022
StataCorp LLC (StataCorp) strives to provide our users with exceptional products and services. To do so, we must collect personal information from you. This information is necessary to conduct business with our existing and potential customers. We collect and use this information only where we may legally do so. This policy explains what personal information we collect, how we use it, and what rights you have to that information.
These cookies are essential for our website to function and do not store any personally identifiable information. These cookies cannot be disabled.
This website uses cookies to provide you with a better user experience. A cookie is a small piece of data our website stores on a site visitor's hard drive and accesses each time you visit so we can improve your access to our site, better understand how you use our site, and serve you content that may be of interest to you. For instance, we store a cookie when you log in to our shopping cart so that we can maintain your shopping cart should you not complete checkout. These cookies do not directly store your personal information, but they do support the ability to uniquely identify your internet browser and device.
Please note: Clearing your browser cookies at any time will undo preferences saved here. The option selected here will apply only to the device you are currently using.