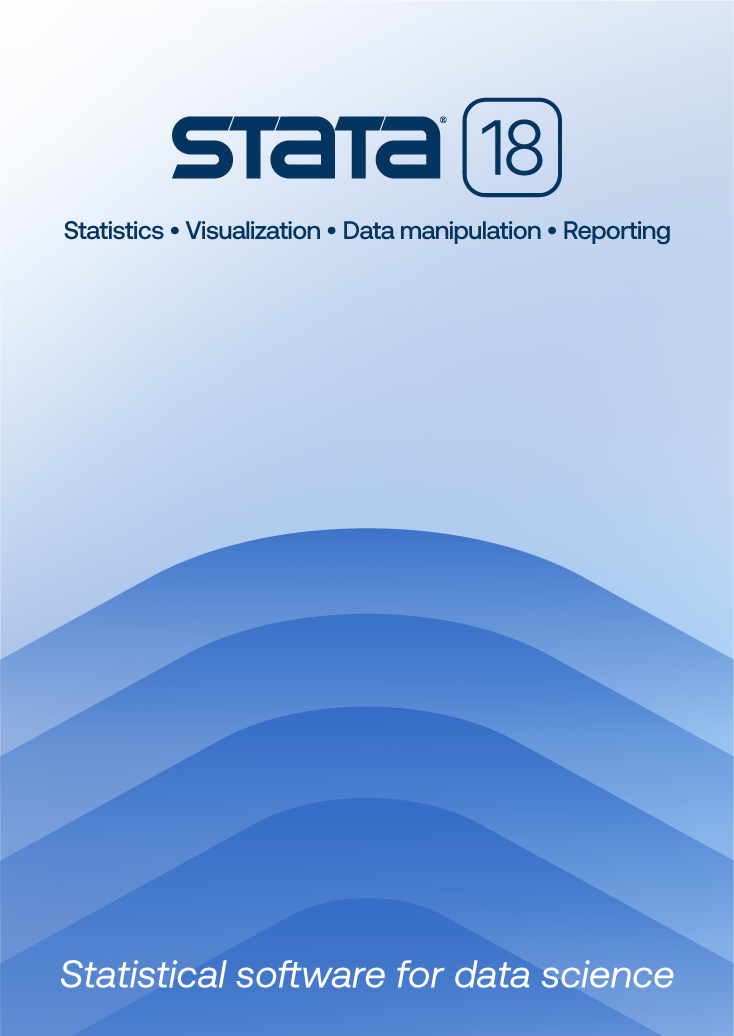
Title | Fitting ordered logistic and probit models with constraints | |
Author |
Mark Inlow, StataCorp Ronna Cong, StataCorp |
Consider a parameterization in which a constant is present, e.g., Greene’s formulation (Greene 2018, Chapter 18):
Pr(Y = 0) = F(−Xb) Pr(Y = 1) = F(u1 −Xb) − F(−Xb) Pr(Y = 2) = F(u2 −Xb) − F(u1 −Xb) ...
In the preceding, F is the cumulative distribution function (CDF), either the cumulative standard normal distribution for ordered probit regression or the cumulative logistic distribution for ordered logistic regression. Since Greene includes a constant in his Xb, we need to indicate this to make his notation and Stata’s ordered probit/logistic notation comparable:
Pr(Y = 0) = F(−Xb − con) Pr(Y = 1) = F(u1 − Xb − con) − F(−Xb − con) Pr(Y = 1) = F(u2 − Xb − con) − F(u1 −Xb − con) ...
Now, compare this with Stata’s no-constant model:
Pr(Y = 0) = F(/cut1 − Xb) Pr(Y = 1) = F(/cut2 − Xb) − F(/cut1 − Xb) Pr(Y = 2) = F(/cut3 − Xb) − F(/cut2 − Xb) ...
Examining the expressions for Pr(Y = 0), we see that
−Xb − con = /cut1 − Xb
so Greene’s constant equals –/cut1. Greene set the first cut point to zero, whereas Stata set the constant to zero.
Combining this observation with the expressions for Pr(Y = 1), we see that Greene’s u1 = /cut2 + con = /cut2 − /cut1. Doing the same for Pr(Y = 2), we see that u2 = /cut3 − /cut1. Thus to estimate Greene’s model using the coefficient estimates from Stata’s ordered probit/logistic regression commands we can use the following:
Greene's intercept = −/cut1 Greene's u1 = /cut2 − /cut1 Greene's u2 = /cut3 − /cut1 ...
After you fit your model using Stata, you can convert to Greene’s parameterization using lincom, which will provide both the coefficient estimate and the standard error as follows:
ologit/oprobit ... lincom _b[/cut2] - _b[/cut1] lincom _b[/cut3] - _b[/cut1] ...
To make things concrete, consider the following example using the auto dataset, which is shipped with Stata.
. sysuse auto, clear (1978 Automobile Data) . replace rep78 = 2 if rep78 == 1 | missing(rep78) (7 real changes made) . tabulate rep78
Repair | ||
Record 1978 | Freq. Percent Cum. | |
2 | 15 20.27 20.27 | |
3 | 30 40.54 60.81 | |
4 | 18 24.32 85.14 | |
5 | 11 14.86 100.00 | |
Total | 74 100.00 |
rep78 | Coefficient Std. err. z P>|z| [95% conf. interval] | |
price | .0000966 .0000515 1.88 0.061 -4.36e-06 .0001976 | |
weight | -.0007095 .0002013 -3.52 0.000 -.0011041 -.000315 | |
/cut1 | -2.468357 .5580629 -3.56214 -1.374573 | |
/cut2 | -1.276601 .5310947 -2.317528 -.2356748 | |
/cut3 | -.3720451 .5046055 -1.361054 .6169635 | |
Thus the intercept (constant) is −/cut1 = 2.47, and now we compute the point estimate and standard error of u1:
. lincom _b[/cut2] - _b[/cut1] ( 1) - [/]cut1 + [/]cut2 = 0
rep78 | Coefficient Std. err. z P>|z| [95% conf. interval] | |
(1) | 1.191755 .183964 6.48 0.000 .8311925 1.552318 | |
Our estimate of u1 is 1.19 with a standard error of 0.18. Finally we estimate u2:
. lincom _b[/cut3] - _b[/cut1] ( 1) - [/]cut1 + [/]cut3 = 0
rep78 | Coefficient Std. err. z P>|z| [95% conf. interval] | |
(1) | 2.096311 .2457135 8.53 0.000 1.614722 2.577901 | |
Thus our estimate of u2 is 2.096 with a standard error of .246.