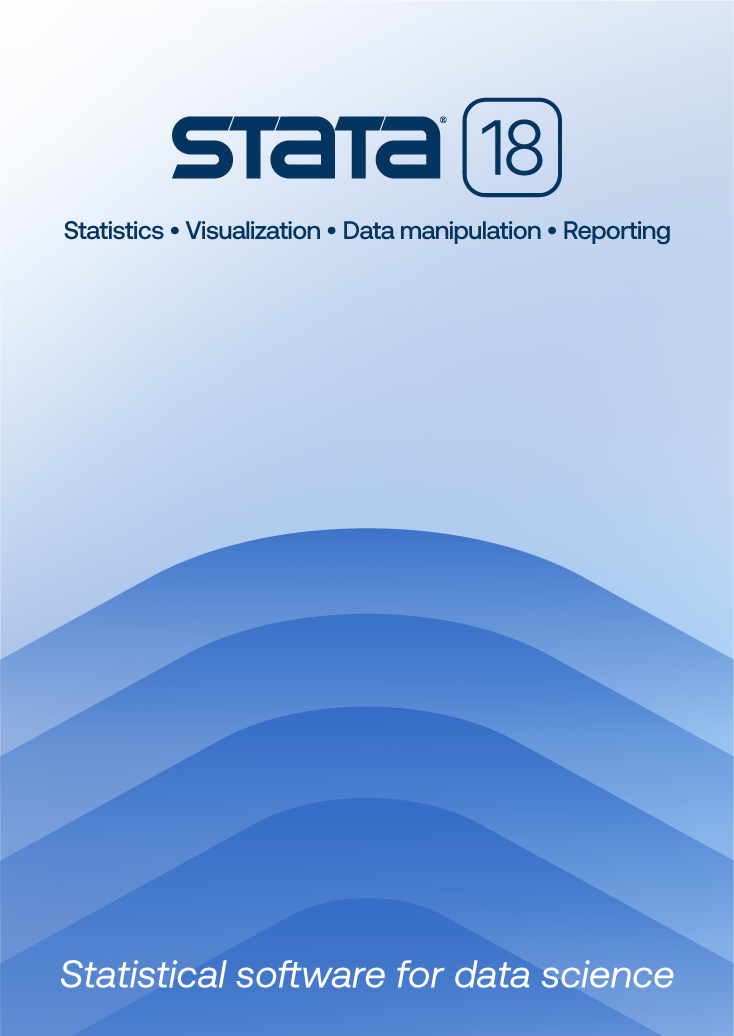
7:10–8:50 | Session 1dstat: A new command for the analysis of distributions Abstract: In this talk, I will present a new Stata command that unites a variety of methods to describe (univariate) statistical distributions. Covered are density estimation, histograms, cumulative distribution functions, probability distributions, quantile functions, Lorenz curves, percentile shares, and a large collection of summary statistics, such as classical and robust measures of location, scale, skewness, and kurtosis, as well as inequality, concentration, and poverty measures.Particular features of the command are that it provides consistent standard errors supporting complex sample designs for all covered statistics and that the simultaneous analysis of multiple statistics across multiple variables and subpopulations is possible. Furthermore, the command supports covariate balancing based on reweighting techniques (inverse probability weighting and entropy balancing), including appropriate correction of standard errors. Standard error estimation is implemented in terms of influence functions, which can be stored for further analysis, for example, in RIF regressions or counterfactual decompositions.
Additional information: Ben Jann
University of Bern
distcomp: Comparing distributions Abstract: I developed the distcomp command to help Stata users compare distributions using recent methodology from the econometrics literature (Goldman and Kaplan 2018; https://doi.org/10.1016/j.jeconom.2018.04.003). Goodness-of-fit tests like ksmirnov simply test the null hypothesis that two distributions are identical; the test can only reject or accept. Providing more information, distcomp identifies specific intervals on which the distributions' difference is statistically significant while still controlling the false-positive rate appropriately. A secondary benefit of distcomp is its improved power in the tails compared with ksmirnov.
Additional information: David Kaplan
University of Missouri
Averaged shifted histograms (ASHs) or weighted averaging of rounded points (WARPs): Efficient methods to calculate kernel density estimators for circular data Abstract: By solving the histograms' problems of origin dependency and discontinuity and by having guidance to choose the best bandwidth and the feasibility of variable bandwidth procedures, the kernel density estimators (KDEs) are powerful tools to explore and analyze data distributions. However, an important drawback of these methods is that they require a considerable number of calculations, which may require a long time to obtain the result, even using fast processors and moderate sample sizes. A way to overcome this problem is through ASHs, a procedure later recognized as being a part of the more general procedure. On the other hand, the information with a circular measure scale commonly occurs in diverse human activities. Circular data distribution must be understood to properly interpret its message. The rose diagram is the histogram equivalent, sharing the same drawbacks along with others derived from the circular scale. In this talk, I present a new program that permits the calculation of kernel density estimators for circular data with different weight functions by means of the ASH–WARP procedure with an impressive calculation time (from minutes to less than a second) when analyzing big datasets.
Additional information: Isaías Hazarmabeth Salgado-Ugarte
FES Zaragoza, Universidad Nacional Autónoma de México
Beyond histograms and box plots: Some commands for univariate distribution graphics Abstract: Whatever we do in statistical science should be rooted in careful and comprehensive description and exploration of the data. This presentation surveys various commands by the author for plotting univariate distributions, without neglecting the need for concise and genuinely informative numerical summaries. Graphical highlights include qplot (SJ) and multqplot (SJ) for quantile plots, the complementary distplot (SJ) for (empirical [cumulative]) distribution plots, multidensity (SSC) for density function estimates, stripplot (SSC) for strip plots and much more, and transplot (SSC) for trying out transformations. Numerical highlights include moments (SSC) as a convenience wrapper for summarize results and (last but not least) lmoments (SSC) for the greatly underappreciated L-moments and derived statistics. SJ = Stata Journal; SSC = Statistical Software Components.
Additional information: Nicholas J. Cox
Durham University
|
9:00–11:15 | Session 2StataCorp presentation: Customizable tables Abstract: Presenting results effectively is a crucial step in statistical analyses, and creating tables is an important part of this step. In this presentation, I will introduce two new features in Stata 17—the updated table command and the new collect suite—that you can use to create, customize, and export tables. With table, you can now easily create cross-tabulations, tables of summary statistics, tables of regression results, and more. With the collect suite, you can create and customize tables of results returned by any Stata command.I will demonstrate how you can create table styles with your favorite customizations and apply them to any tables you create in the future. After creating and customizing a table, you can export to Word, Excel, LaTeX, PDF, Markdown, HTML, SMCL, and plain text. I will also show how you can incorporate your customized tables into complete reports containing formatted text, graphs, and other Stata results.
Additional information: Kristin MacDonald
StataCorp
Incorporating Stata into reproducible documents during survey data management for intervention projects in Africa Abstract: The introduction of Stata 15 commands like putdocx and several community-contributed commands has enabled the automation and use of other software (QGIS, Microsoft Word) for nontechnical data users to get a quick report and analysis of field data. The automation of the process and the reproducibility of the customized report is of great essence.
Additional information: Jerry Agulehi
One Acre Fund Nigeria
xtbreak: Estimation and tests for structural breaks in time series and panel data Abstract: The recent events that have plagued the global economy, such as the 2008 financial crisis or the 2020 COVID-19 outbreak, hint to multiple structural breaks in economic relationships. I present xtbreak, which implements the estimation of single and multiple break points and testing for structural breaks in time series and panel data. The estimation and the tests follow the methodologies developed in Andrews (1993, Econometrica), Bai and Perron (1998, Econometrica), and Ditzen, Karavias, and Westerlund (2021). For both time-series and panel-data regressions, five tools are provided: (i) a test of no structural change against the alternative of a specific number of changes, (ii) a test of the null hypothesis of no structural change against the alternative of an unknown number of structural changes, (iii) a test of the null of s changes against the alternative of s-1 changes, (iv) consistent break date estimators, and (v) asymptotically valid confidence intervals for the break dates.
Additional information: Jan Ditzen
Free University of Bolzano-Bozen
An LM test for the mean stationarity assumption in dynamic panel-data models Abstract: I present the new Stata command xttestms for computation of the LM test for verifying the assumptions underlying the system GMM estimation in the context of dynamic panel-data models. The test has been proposed by Magazzini and Calzolari (2020), who show its better performance with respect to testing procedures customarily employed in empirical research (that is, the Sargan–Hansen test checking the whole set of moment conditions of the system GMM approach and the difference-in-Sargan–Hansen, which compares the value of the minimized criterion function of the system and difference GMM approaches). The command can be run after system GMM estimation by using either the Stata command xtdpdsys or the command xtabond2 by Roodman (2009) to verify that the additional moment conditions that characterize the system GMM estimator are satisfied; that is, it verifies the validity of the mean stationarity assumption for the initial conditions. A set of Monte Carlo experiments will be performed to further assess the properties of the testing procedure, and two examples will be considered to show how the proposed command can be applied in empirical research.
Additional information: Laura Magazzini
Institute of Economics, Sant'Anna School of Advanced Studies
|
11:25–12:35 | Session 3One weird trick for better inference in experimental designs Abstract: A long line of research debates the merits of statistical adjustment for baseline or pretreatment characteristics for random assignment designs (Fisher 1935; Freedman 2008a, 2008b; Lin 2013; Kallus 2018). A related literature explores better methods to conduct statistical adjustment for potential confounders in nonexperimental designs. I present the results of a simulation showing large potential improvements in inference for random assignment designs attainable using commands designed to adjust for potential confounders newly available in Stata 16.
Additional information: Austin Nichols
Abt Associates
A “CACE” in point: Estimating causal effects via a latent class approach in RCTs with noncompliance using Stata Abstract: In randomized control trials (RCT), intention-to-treat (ITT) analysis is customarily used to estimate the effect of the trial; however, in the presence of noncompliance, this can often lead to biased estimates because ITT completely ignores varying levels of actual treatment received. This is a known issue that can be overcome by adopting the complier average causal effect (CACE) approach, which estimates the effect the trial had on the individuals who complied with the protocol. This can be obtained via a latent class specification when compliance is unobserved in the control group, under certain reasonable assumptions, for example, randomization, exclusion restriction, and ignorable missingness. This model is fit as a mixture model for the outcome of interest with two latent classes: a) compliers and b) noncompliers. This presentation will briefly introduce the issues around noncompliance and the assumptions of the CACE model. It will then illustrate the use of the gsem command in Stata 15 onward to estimate this effect with open access data and compare across other commonly used software packages. Finally, results using this approach in the context of a recent school-based RCT in England, the Good Behaviour Game (GBG), will be discussed.
Additional information: Patricio Troncoso
Heriot-Watt University
Using the package hettreatreg to interpret OLS estimates under treatment-effect heterogeneity Abstract: This presentation describes hettreatreg, a Stata package to compute diagnostics for linear regression when treatment effects are heterogeneous. Following my recent paper, "Interpreting OLS Estimands When Treatment Effects Are Heterogeneous: Smaller Groups Get Larger Weights" (forthcoming, Review of Economics and Statistics), every OLS estimate of the coefficient on a binary variable ("treatment") in a linear model with additive effects can be represented as a weighted average of two other estimates, corresponding with average treatment effects on the treated (ATT) and untreated (ATU). Surprisingly, the weights on these estimates are inversely related to the proportion of observations in each group. Thus, when there are very few treated (untreated) observations, OLS estimates are similar to those of the ATT (ATU). When the sample is roughly balanced, OLS estimates are similar to those of the average treatment effect (ATE). The package hettreatreg estimates the OLS weights on ATT and ATU, computes the associated model diagnostics, and reports the implicit OLS estimates of ATE, ATT, and ATU. I illustrate the use of hettreatreg with empirical examples.
Additional information: Tymon Słoczyński
Brandeis University
|
12:45–2:25 | Session 4StataCorp presentation: Estimation, inference, and diagnostics for difference in differences Abstract: Stata 17 introduced two commands to fit difference-in-differences (DID) and difference-in-differences-in-differences (DDD) models. One of the commands is applicable to repeated cross-sectional data, didregress, and the other to panel/longitudinal data, xtdidregress. I will talk briefly about the theory behind DID and DDD models and then show how to fit the models by using the new commands. I will spend some time discussing the standard errors that are appropriate to use in different scenarios. I also discuss graphical diagnostics and tests that are relevant for DID and DDD specifications. Finally, I discuss new areas of development in the DID literature.
Additional information: Enrique Pinzón
StataCorp
Estimation of average treatment effects in staggered difference-in-differences designs Abstract: In this presentation, I discuss the att_gt command, which implements three semiparametric estimators for a family of average treatment-effects parameters in difference-in-differences (DID) setups with multiple time periods discussed in Callaway and Sant’Anna (2020, https://doi.org/10.1016/j.jeconom.2020.12.001). The first estimator models the outcome evolution of the comparison group, the second is a properly reweighted inverse-probability weighted estimator, and the third is a doubly robust estimator that relies on less stringent modeling assumptions. Our implementation allows for the use of different comparison groups (“never-treated” or “not-yet-treated” units) and also allows for limited treatment anticipation. Our inference procedures account for multiple-testing problems. We discuss postestimation approaches that can be used in conjunction with our main implementation. We illustrate the program and provide a simulation study assessing the finite-sample performance of the inference procedures.
Additional information: Pedro Sant'Anna
Vanderbilt University
Trusting difference-in-difference estimates more: An approximate permutation test Abstract: Researchers use difference-in-differences models to evaluate the causal effects of policy changes. Because the empirical correlation across firms and time can be ambiguous, estimating consistent standard errors is difficult, and statistical inferences may be biased. I apply an approximate permutation test using simulated interventions to reveal the empirical error distribution of estimated policy effects. In contrast to existing econometric corrections, such as single or double clustering, this approach does not impose a specific parametric form on the residuals. In comparison with alternative parametric tests, this procedure maintains correct size with simulated and real-world interventions. Simultaneously, it improves power.
Additional information: Sebastian Bunnenberg
Reutlingen University, ESB Business School
allsynth: Synthetic control bias-corrections utilities for Stata Abstract: The synthetic control method has become a widely adopted empirical approach for estimating counterfactuals and treatment effects. The synth module written for Stata (Abadie, Diamond, and Hainmueller 2010) is widely used by practitioners and serves as the foundation for the synth_runner utilities package (Galiani and Quistorff 2018), which enhances functionality. An active literature has proposed numerous modifications to the "classic" approach, including a bias-correction procedure (Abadie and L'Hour 2020), analogous to that in Abadie and Imbens (2011) for matching estimators, to remove bias that results from differences in the predictor variables between a treated unit and its synthetic control donors. allsynth adds functionality to the synth module, which implements this bias-correction procedure and automates extension of the procedure to placebo runs for in-space randomization inference and graphing.
Additional information: Justin Wiltshire
University of California, Davis
|
2:35–4:35 | Session 5netivreg: Estimation of peer effects in endogenous social networks Abstract: I present the netivreg command, which implements the generalized three-stage least-squares (G3SLS) estimator for the endogenous linear-in-means model developed in Estrada et al. (2020, “On the Identification and Estimation of Endogenous Peer Effects in Multiplex Networks"). The G3SLS procedure utilizes full observability of a two-layered multiplex network data structure using Stata 16's new multiframes capabilities and Python integration. Implementations of the command utilizing simulated data as well as three years' worth of data on peer-reviewed articles published in top general-interest journals in economics in Estrada et al. (2020) are also included.
Additional information: Juan Estrada
Emory University
Network regressions in Stata Abstract: Network analysis has become critical to the study of social sciences. While several Stata programs are available for analyzing network structures, programs that execute regression analysis with a network structure are currently lacking. We fill this gap by introducing the nwxtregress command. Building on spatial econometric methods (LeSage and Pace 2009), nwxtregress uses MCMC estimation to produce estimates of endogenous peer effects, as well as own-node (direct) and cross-node (indirect) partial effects, where nodes correspond with cross-sectional units of observation, such as firms, and edges correspond with the relations between nodes. Unlike existing spatial regression commands (for example, spxtregress), nwxtregress is designed to handle unbalanced panels of economic and social networks as in Grieser et al. (2021). Networks can be directed or undirected with weighted or unweighted edges, and they can be imported in a list format that does not require a shapefile or a Stata spatial weight matrix set by spmatrix. Finally, the command allows for the inclusion or exclusion of contextual effects. To improve speed, the command transforms the spatial weighting matrix into a sparse matrix. Future work will be targeted toward improving sparse matrix routines, as well as introducing a framework that allows for multiple networks.
Additional information: Morad Zekhnini
Michigan State University
glasso: Graphical lasso for learning sparse inverse-covariance matrices Abstract: In modern multivariate statistics, where high-dimensional datasets are ubiquitous, learning large inverse-covariance matrices is a fundamental problem. A popular approach is to apply a penalty on the Gaussian log-likelihood and solve the convex optimization problem. Graphical lasso (Glasso) (Friedman et al. 2008) is one of the efficient and popular algorithms for imposing sparsity on the inverse covariance matrix. In this article, we introduce a corresponding new command, glasso and explore the details of the algorithm. Moreover, we discuss widely used criteria for tuning parameter selection, such as the extended Bayesian information criterion (eBIC) and cross-validation (CV), and introduce corresponding commands. Simulation results and real data analysis illustrate the use of the Glasso.
Additional information: Aramayis Dallakyan
Texas A&M University
Calprotectin, an emerging biomarker of interest in COVID-19: Meta-analysis using Stata Abstract: COVID-19 has been shown to present with a varied clinical course, hence the need for more specific diagnostic tools that could identify severe cases and predict outcomes during COVID-19 infection. Recent evidence has shown an expanded potential role for calprotectin, both as a diagnostic tool and as a stratifying tool in COVID-19 patients in terms of severity. Therefore, this systematic review and meta-analysis aims to evaluate the levels of calprotectin in severe and nonsevere COVID-19 and also identify the implication of raised calprotectin levels. Databases searched include MEDLINE, EMBASE, the Cochrane Library, Web of Science, and MedRxiv. Stata was employed in meta-analysis to compare the serum/fecal levels of calprotectin between severe and nonsevere COVID-19 infections. A pooled analysis of data in the eight quantitative studies from 613 patients who were RT-PCR positive for COVID-19 (average age = 55 years; 52% males) showed an overall estimate as 1.34 (95% CI: [0.77, 1.91]). Stata was further employed to carry out an in-depth investigation of the in-between study heterogeneity. In conclusion, calprotectin levels have been demonstrated to be significantly elevated in COVID-19 patients who develop the severe form of the disease, and it also has prognostic importance.
Additional information: Raphael Udeh
University of Newcastle, Australia
|
7:10–9:00 | Session 6Hunting for the missing score functions Abstract: Specific econometric models—such as the Cox regression, conditional logistic regression, and panel-data models—have likelihood functions that do not meet the so-called linear-form requirement. That means that the model's overall log-likelihood function does not correspond with the sum of each observation's log-likelihood contribution. Stata's ml command can fit said models using a particular group of evaluators: the d-family evaluators. Unfortunately, they have some limitations; one is that we cannot directly produce the score functions from the postestimation command predict.This missing feature triggers the need for tailored computational routines from developers that might need those functions to compute, for example, robust variance–covariance matrices. In this talk, I present a way to compute the score functions numerically using Mata's deriv() function with minimum extra programming other than the log-likelihood function. The procedure is exemplified by replicating the robust variance–covariance matrix produced by the clogit command using simulated data. The results show negligible numerical differences (e-09) between the clogit robust variance–covariance matrix and the numerically approximated one using Mata's deriv() function.
Additional information: Álvaro A. Gutiérrez-Vargas
Research Centre for Operations Research and Statistics, KU Leuven
rbprobit: Recursive bivariate probit estimation and decomposition of marginal effects Abstract: This article describes a new Stata command, rbprobit, for fitting recursive bivariate probit models, which differ from bivariate probit models in allowing the first dependent variable to appear on the right-hand side of the second dependent variable. Although the estimation of model parameters does not differ from the bivariate case, the existing commands biprobit and cmp do not consider the structural model’s recursive nature for postestimation commands. rbprobit estimates the model parameters, computes treatment effects of the first dependent variable, and gives the marginal effects of independent variables. In addition, marginal effects can be decomposed into direct and indirect effects if covariates appear in both equations. Moreover, the postestimation commands incorporate the two community-contributed goodness-of-fit tests scoregof and bphltest. Dependent variables of the recursive probit model may be binary, ordinal, or a mixture of both. I present and explain the rbprobit command and the available postestimation commands using data from the European Social Survey. Finally, I show an application of the difference-in-differences methodology if there is an interaction term between the first dependent variable and a group variable.
Additional information: Mustafa Coban
Institute for Employment Research (IAB)
Estimation of ordered probit model with endogenous switching between two latent regimes Abstract: Ordinal responses can be generated, in the time-series context, by different latent regimes or, in the cross-sectional context, by different unobserved groups of population. These latent classes or states can distort the inference in a traditional single-equation model. Finite-mixture or regime-switching models surmount the problem of unobserved heterogeneity or clustering through their flexible form. The available Stata command for finite mixture of ordered probit models, fmm: oprobit, does not allow for endogenous switching when the unobservables in the switching equation are correlated with the unobservables in the outcome equations. We introduce two new commands, swopit and swopitc, that fit a switching ordered probit model for ordered choices with exogenous and endogenous switching between two unobserved regimes or groups. We provide a battery of postestimation commands, access the small-sample performance of the maximum likelihood estimator of the parameters and the bootstrap estimator of standard errors by Monte Carlo experiments, and apply the new commands to model the policy interest rates and health status responses.
Additional information: Jan Willem Nijenhuis
University of Amsterdam
Canceled Abstract: Latent variables are used in economics to represent measures that influence the behavior or capture the traits of economic agents. Inference with latent variables often requires classifying individuals based on estimates of these variables to make analyses more tractable and easier to convey one’s findings to a wider audience. While classifying individuals is often straightforward, requiring estimates of their latent variables and their corresponding standard errors, and cutpoints, relatively few instruments are without measurement error. In many cases, this measurement error is transferred onto the estimates of individuals’ latent variables, which may result in individuals being misclassified (Rudner 2001; Lee 2020; Lathrop 2015). Methodology has been developed to assess the extent of misclassification under item response theory (IRT). These methods rely on two indices, classification accuracy and classification consistency, to describe the quality of classification decisions. The former is a measure of the validity, while the latter is a measure of the reliability of classifications. In this presentation, I motivate the study of misclassification under IRT, introduce Stata users to a novel community-contributed estimation command based on the Rudner method (Rudner 2001, 2005), irtacc, and provide an empirical example of an application of this command.
Matthew P. Rabbitt
U.S. Department of Agriculture
Censored demand system estimation Abstract: We introduce the command quaidsce, a modified version of the estimation command provided by Poi (2008) to estimate the Almost Ideal Demand System proposed by Deaton and Muellbauer (1980) and extended by Banks et al. (1997) to allow for nonlinear demographic effects (through a price deflator for total expenditure) and nonlinear Engel curves (through a quadratic term of total expenditure). The command of Poi (2008) to estimate the Quadratic Almost Ideal Demand System (QUAIDS) is extended to a two-step censoring demand system. Postestimation tools calculate expenditure and price elasticities.
Additional information: Grace Melo
University of Chile
|
9:10–11:00 | Session 7Making Stata estimation commands faster through automatic differentiation and integration with Python Abstract: Fitting complex statistical models to very large datasets can be frustratingly slow. This is particularly problematic if multiple models need to be fit, for example, when using bootstrapping, cross-validation, or multiple imputation. I will introduce the mlad command, as an alternative to Stata's ml command, to estimate parameters using maximum likelihood. Rather than writing a Stata or Mata function to calculate the likelihood, mlad requires this to be written in Python.A key advantage is that there is no need to derive the gradient vector or the Hessian matrix because these are obtained through automatic differentiation using the Python Jax module. In addition, the functions for the likelihood, gradients, and Hessian matrix are compiled and able to use multiple processors. This makes maximizing likelihoods using mlad easier to implement and substantially faster than using ml with the advantage that all results are returned to Stata. Implementing mlad on the author’s own estimation commands leads to speed improvements of 70–98% compared with ml. The syntax of mlad is almost identical to that of ml, making it easy for programmers to add an option to their estimation command so that users using large datasets can benefit from the speed improvements.
Additional information: Paul Lambert
University of Leicester / Karolinska Institutet
Machine learning using Stata/Python Abstract: We present two related Stata modules, r_ml_stata and c_ml_stata, for fitting popular machine learning (ML) methods in both regression and classification settings. Using the recent Stata/Python integration platform (sfi) of Stata 16, these commands provide hyperparameters' optimal tuning via K-fold cross-validation using greed search. More specifically, they make use of the Python Scikit-learn API to carry out both cross-validation and outcome/label prediction.
Additional information: Giovanni Cerulli
IRCrES-CNR
Beyond n-grams, tf-idf, and word indicators for text: Leveraging the Python API for vector embeddings Abstract: This talk will share strategies that Stata users can use to get more informative word, sentence, and document vector embeddings of text in their data. While indicator and bag-of-words strategies can be useful for some types of text analytics, they lack the richness of the semantic relationships between words that provide meaning and structure to language. Vector space embeddings attempt to preserve these relationships and in doing so can provide more robust numerical representations of text data that can be used for subsequent analysis. I will share strategies for using existing tools from the Python ecosystem with Stata to leverage the advances in NLP in your Stata workflow.
Additional information: William Buchanan
SAG Corporation
A Stata 17 implementation of the local ratio autonomy: Calling Python Abstract: In many countries around the world, the public sector is decentralized to improve efficiency in the provision of public services. Until the publication of the paper by Martínez-Vazquez, Vulovic, and Liu (2011), the level of decentralization was approximated through the local income ratio. It has been shown that this covariate is endogenous and that because of the unobservable heterogeneity, it can generate correlation. The local autonomy ratio proposed by these authors is an indicator weighted by the inverse of the distance between municipalities, which in turn is weighted by the sum of the inverse of the distance between all municipalities in the country. However, we propose a local autonomy ratio, conditioned by the distance and population thresholds between the country's municipalities. It is evident that multiple distance and population restrictions must be tested until the effect of this ratio is found to be significant, as a covariate in an econometric model. To reduce the computational cost-time of the estimation, we automated the calculation of the indicator, programming local ratio autonomy in Stata 16 but calling Python. We use Python version 3.9.
Additional information: Juan S. Morales-Castillo
University of Granada
|
11:10–12:00 | Open panel discussion with Stata developers |
12:00–1:40 | Session 8StataCorp presentation: Bayesian econometrics in Stata 17 Abstract: Stata 17 introduced Bayesian support for many time-series and panel-data commands. In this talk, I will discuss Bayesian vector autoregression models, Bayesian DSGE models, and Bayesian panel-data models. Bayesian estimation is well suited to these models because economic considerations often impose structure that is captured well by informative priors. I will describe the main features of these commands as well as Bayesian diagnostics, posterior hypothesis tests, predictions, impulse–response functions, and forecasts.
Additional information: David Schenck
StataCorp
Use of the bayesmh command in Stata to calculate excess relative and excess absolute risk for radiation health risk estimates Abstract: Excess relative risk (ERR) and excess absolute risk (EAR) are important metrics typically used in radiation epidemiology studies. Most studies of long-term radiation effects in Japanese atomic bomb survivors feature Poisson regression of grouped survival data. Risks are modeled on the excess risk scale using linear and log-linear functions of regression parameters, which are generally formulated to produce both ERR and EAR as output. Given the specific assumptions underlying these models, they are dubbed ERR and EAR models, respectively. Typically, these models are fit using the Epicure software that was specifically designed to fit these models, and they are difficult to reproduce in more accessible software. The flexibility of the bayesmh command can be utilized to fit these models within a Bayesian framework, which may increase accessibility in the broader statistical and epidemiological communities. In this presentation, I detail ERR and EAR model fitting and assumptions, and I give an example of how the models can be fit in Stata using Bayesian methods.
Additional information: Lori Chappell
KBR
Structural equation modeling the ICU patient microbiome and risk of bacteremia Abstract: Whether Candida interacts with Gram-positive bacteria to enhance their invasive potential from the microbiome leading to infection within intensive care unit (ICU) patients remains unclear. These infections arise from the colonizing flora, but quantifying microbial colonization in patients is not simple. Using published ICU infection prevention data, one can model the interaction between Candida and Gram-positive bacteria (at the level of the ICU) using generalized structural equation models (GSEM). In these models, colonization is a latent variable defined by the proportion of patients with respiratory tract or blood stream infections. The various ICU infection prevention interventions (as studied within more than 250 publications) variously impact colonization with Candida and Gram-positive bacteria, which are measured as latent variables within the GSEM. The models provide support to interactions occurring between Candida and Gram-positive bacteria, contributing to bacteremia and pneumonia with these microbes within ICU patients. Similar GSEM modeling likewise implicates interactions between Candida and Gram-negative bacteria contributing to bacteremia.
Additional information: James Hurley
University of Melbourne
|
1:50–3:20 | Session 9Joint estimation of employment and unemployment hazard rates with unobserved heterogeneity using the hshaz2s command Abstract: In this talk, I present hshaz2s, a new Stata command that fits two-states proportional-hazard rates models with unobserved heterogeneity specific to each of the two modeled states. hshaz2s uses the d2 ml method to provide the algebraic expressions of the first- and second-order derivatives of the log-likelihood function to achieve the model convergence faster, which is especially relevant for empirical researchers dealing with large longitudinal microdata sets. Results of fitting a discrete time duration model that jointly estimates the transition rates from employment and unemployment states on a sample of workers in the Spanish labor market are presented to show the main features of hshaz2s.
David Troncoso-Ponce
University of Seville
Canceled Abstract: The dynamics of markups both at the firm and at the economy-wide level has recently attracted renewed attention from scholars, institutions, and broader audiences. In this presentation, I review the main methods for markup estimation both at the micro and at the macro level, and I provide methodological insights as a guide for applied researchers. Finally, I present a new Stata module (markupest) that implements all methods and an addition to the user-contributed module prodest, aimed at estimating markups after the estimation of a production function. I show their main features and performance both on example firm-level production datasets and on national accounts data and further strengthen the results through a series of Monte Carlo simulations.
Gabriele Rovigatti
Bank of Italy
Measurement error and misclassification in linked earnings data: Estimation of the Kapteyn and Ypma model Abstract: Kapteyn and Ypma (KY; 2007, https://doi.org/10.1086/513298) is an influential study for the analysis of linked administrative and survey earnings data that was the first to allow for measurement errors in both sources of data. Allowing for measurement errors in administrative data, they find evidence that the oft-cited feature of mean-reversion errors in survey data virtually disappeared. In this talk, I introduce a new set of commands that facilitates the estimation of the KY measurement error model, expanding on the theoretical model proposed by KY, and incorporating insights from Meijer, Rohwedder, and Wansbeek (2012, https://doi.org/10.1198/jbes.2011.08166). These commands are ky_fit, a command that can be used to fit the KY model, including the proposed extensions; ky_estat, an add-on for estat that allows the user to obtain summary statistics of important features of the KY model, including measurements of data reliability; ky_p, an add-on for predict and margins that allows obtaining model predictions and marginal effects of the model; and ky_sim, a command that can simulate data based on the fitted models.
Additional information: Fernando Rios-Avila
Levy Economics Institute
|
3:30–5:00 | Session 10Drivers of COVID-19 outcomes: Evidence from a heterogeneous SAR panel-data model Abstract: In an extension of the standard spatial autoregressive (SAR) model, Aquaro, Bailey and Pesaran (ABP; 2021, https://doi.org/10.1002/jae.2792) introduced a SAR panel model that allows one to produce heterogeneous point estimates for each spatial unit. Their methodology has been implemented as the Stata routine hetsar (Belotti, 2021, Statistical Sofware Components S458926). As the COVID-19 pandemic has evolved in the U.S. since its first outbreak in February 2020 with following resurgences of multiple widespread and severe waves of the pandemic, the level of interactions between geographic units (for example, states and counties) has differed greatly over time in terms of the prevalence of the disease.Applying ABP’s HETSAR model to 2020 and 2021 COVID-19 data outcomes (confirmed case and death rates) at the state level, we extend our previous spatial econometric analysis (Baum and Henry, 2020, Boston College Working Papers in Economics 1009) on socioeconomic and demographic factors influencing the spatial spread of COVID-19 confirmed case and death rates in the U.S.
Additional information: Kit Baum
Boston College
Vaccination coverage quality indicators (VCQI): A flexible collection of Stata programs for standardized survey data analysis Abstract: Household surveys are a vital source of data on childhood vaccination in low- and middle-income countries. To evaluate effectiveness of government vaccination programs, the World Health Organization (WHO) has developed a set of outcome indicators that may be calculated from survey data. In this talk, I describe our collection of Stata programs—called Vaccination Coverage Quality Indicators (VCQI)—that WHO makes freely available so survey analysts can calculate those indicators in a consistent and transparent manner from surveys that can vary in many specific details. Although the collection consists of more than 300 ado-programs, the survey analyst interacts only with a single do-file—a "control program" that orchestrates calls to the other programs as needed. Only moderate Stata skills are required for an analyst to adapt and run VCQI control programs. This work has prompted several improvements in Stata’s survey estimation commands, and the collection includes useful extensions to Stata’s survey capabilities (for example, meaningful confidence intervals when 0% or 100% of respondents have the outcome). Most of VCQI’s programs are highly interdependent and focused on the specific topic of childhood vaccination, but several are generically useful for survey data analysis.
Additional information: Dale Rhoda
Biostat Global Consulting
Validating a user-developed bivariate pseudo-random vector generator Abstract: Testing based on simulated data is an important component of the design and assessment of a newly developed estimation method. Often, the relevant modeling context involves bivariate outcomes, for example, endogenous treatment-effect (ETE) models and nonlinear seemingly unrelated regressions (SUR). Stata offers reliable commands for univariate pseudo-random-number generators for a wide variety of probability distributions but, as is the case for all statistical software packages, does not provide similar commands for bivariate pseudodata simulation. This is of course reasonable, given the myriad of extant bivariate probability laws and the inherent technical challenges posed by the lack of a generic bivariate version of the inverse transform theorem. In such cases, it is left to the researcher to develop and implement the requisite bivariate data generator using Stata programming or Mata code. Reliability must be established before using such a user-developed simulator to generate data for assessing the feasibility, accuracy, and precision of a newly developed estimator. We propose a Mata-based approach for validating user-developed bivariate simulator reliability based on comparison of the cumulative bivariate relative frequencies for the generated data to the corresponding “true” bivariate cumulative distribution function values. Interesting illustrative examples in the ETE and SUR contexts are discussed.
Additional information: Joseph Terza
IUPUI
|
In light of this year's change to a virtual platform because of COVID-19, we are pleased to announce all proceeds from registrations for the 2021 Stata Conference were donated to Feeding America.
Registration is now closed.
Abt Associates
University of California, Berkeley
UCLA, ret.
Amazon