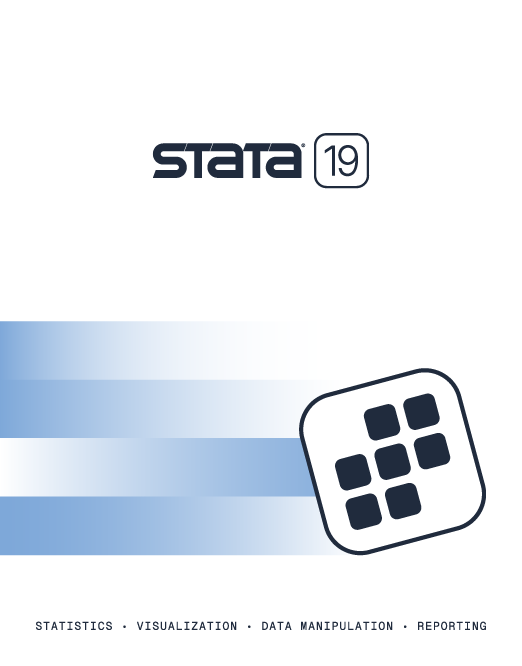
10:15–11:15 | Finite mixture models for linked survey and administrative data
Abstract:
Researchers use finite mixture
models to analyze linked survey and administrative data on
labor earnings (or similar variables), taking account of
various types of measurement error in each data source.
Different combinations of error-ridden and error-free
observations characterize latent classes. Latent class
probabilities depend on the probabilities of the different types
of error. We introduce a set of Stata commands to fit a general
class of finite mixture models to fit to linked
survey-administrative data. We also provide postestimation
commands for assessment of reliability, marginal effects, data
simulation, and prediction of hybrid earnings variables that
combine information from both data sources.
Contributor:
Fernando Rios-Avila
Bard College
Additional information:
Stephen P. Jenkins
The London School of Economics and Political Science
|
11:45–12:15 | A mixture of ordered probit models with endogenous switching between two latent classes
Abstract:
Ordinal responses can be generated, in a time-series context, by
different latent regimes or, in a cross-sectional context, by
different unobserved classes of population.
We introduce a new command, swopit, that fits a mixture of
ordered probit models with either exogenous or endogenous switching
between two latent classes (or regimes). Switching is endogenous
if the unobservables in the class-assignment model are
correlated with the unobservables in the outcome models. We
provide a battery of postestimation commands, assess by Monte
Carlo experiments the finite-sample performance of the maximum
likelihood estimator of the parameters, probabilities and their
standard errors (both the asymptotic and bootstrap ones), and
apply the new command to model the policy interest rates.
Contributors:
Jochem Huismans
Universiteit van Amsterdam
Andrei Sirchenko
Universiteit Maastricht
Additional information:
Jan Willem Nijenhuis
Universiteit Twente
|
12:15–12:45 | nwxtregress: Network regressions in Stata
Abstract:
Network analysis has become critical to the study of social sciences.
While several Stata programs are available for analyzing network
structures, programs that execute regression analysis with a
network structure are currently lacking. We fill this gap by
introducing the nwxtregress command. Building on spatial
econometric methods (LeSage and Pace 2009), nwxtregress uses
MCMC estimation to produce estimates of endogenous peer effects,
as well as own-node (direct) and cross-node (indirect) partial
effects, where nodes correspond to cross-sectional units of
observation, such as firms, and edges correspond to the
relations between nodes. Unlike existing spatial regression
commands (for example, spxtregress), nwxtregress is
designed to handle unbalanced panels of economic and social networks
as in Grieser et al. (2021). Networks can be directed or undirected
with weighted or unweighted edges, and they can be imported in a
list format that does not require a shapefile or a Stata spatial
weight matrix set by spmatrix. Finally, the command allows for
the inclusion or exclusion of contextual effects. To improve
speed, the command transforms the spatial weighting matrix into
a sparse matrix. Future work will be targeted toward improving
sparse matrix routines, as well as introducing a framework that
allows for multiple networks.
Contributors:
William Grieser
Texas Christian University
Morad Zekhnini
Michigan State University
Additional information:
Jan Ditzen
Free University of Bozen-Bolzano
|
1:45–2:15 | Visualizing categorical data with hammock plots
Abstract:
Visualizing data with more than two variables is not straightforward,
especially when some variables are categorical rather
than continuous.
My hammock plots are one option to visualize categorical data
and mixed categorical or continuous data. Hammock plots can be
viewed as a generalization of parallel coordinate plots where
the lines are replaced by rectangles that are proportional to
the number of observations they represent. Hammock plots also
incorporate optional univariate descriptors such as category
labels into the graph. I will introduce my Stata program for
hammock plots and give examples.
Additional information:
Matthias Schonlau
University of Waterloo
|
2:15–2:45 | Measuring associations and evaluating forecasts of categorical and discrete variables
Abstract:
This presentation introduces a new Stata command, classify,
that constructs a classification table and computes various
measures of association between two categorical variables, as
well as diagnostic scores of the
accuracy of probabilistic and deterministic forecasts of a
categorical (binary and multiclass ordinal or nominal) variable.
We compiled a comprehensive list of about 200 coefficients,
along with the synonymy and bibliography associated with them.
In addition to the general measures, the command also computes
the class-specific measures for each class as well as their macro
and weighted averages.
Contributors:
Jochem Huismans
Universiteit van Amsterdam
Andrei Sirchenko
Universiteit Maastricht
Additional information:
Jan Willem Nijenhuis
Universiteit Twente
|
3:15–3:45 | Difference-in-differences estimation using Stata
Abstract:
Difference-in-differences (DID) estimation has become a popular
tool in the context of treatment-effects estimation and program
evaluation.
In this presentation, I will show how to use Stata’s
didregress and xtdidregress commands to estimate
treatment effects with repeated cross-sectional as well as panel
data. I will also discuss a variety of methods for calculating
cluster–robust standard errors when the number of clusters is
small. Finally, I will show how to use diagnostic tools for
checking the parallel-trends assumption, which is an identifying
assumption of DID.
Additional information:
Joerg Luedicke
StataCorp
|
3:45–4:30 | Open panel discussion with Stata developers
Contribute to the Stata community by sharing your feedback with StataCorp's developers. From feature improvements to bug fixes and new ways to analyze data, we want to hear how Stata can be made better for our users.
|
Sven Spieß
9 June from 12:00 p.m. to 7:00 p.m. CEST
Reproducibility has always been a hallmark of Stata. The popular version control system, Git, offers useful additions to the versioning features implemented in Stata with regard to keeping track of revisions of individual (do-)files over the course of evolving research projects. The advantages are even more substantive in “distributed” projects where collaborators do n0t necessarily work on a common infrastructure. Leveraging Git in combination with the power of dynamic documents furthers your ability to easily present and disseminate your most recent findings.
In this workshop, you will first learn the basics of working with the free and open-source version control system Git in conjunction with Stata. After having Git up and running, you will dive into Stata’s facilities for creating dynamic documents to automatically reflect changes in our analyses and data.
Prerequisites:
Alexander Schmidt-Catran Goethe-Universität Frankfurt am Main |
Christian Czymara Goethe-Universität Frankfurt am Main |
Johannes Giesecke Humboldt-Universität zu Berlin |
Ulrich Kohler Universität Potsdam |
The logistics organizer for the 2022 German Stata Conference is DPC Software GmbH, the official distributor of Stata in Germany, the Netherlands, Austria, the Czech Republic, and Hungary.
View the proceedings of previous Stata Conferences and Users Group meetings.