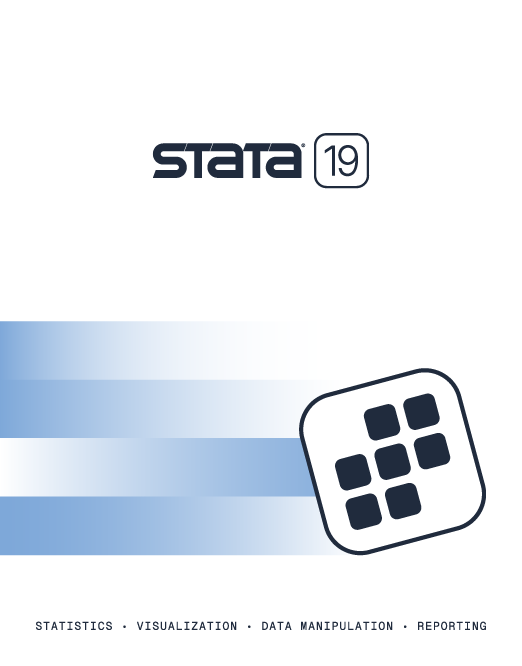
Last updated: 16 June 2014
University of Hamburg
Van-Melle-Park 6 ("Philosophenturm")
Hörsaal E (ground floor)
Hamburg, Germany
Additional materials:
de14_parner.pdf
Sequence analysis (SA) is a very different way of looking at categorical longitudinal data, such as life-course or labor-market histories (or any ordered categorical data, for that matter). Instead of focusing on transition rates (for example, via hazard rate, Markov, or panel models), SA takes individual time series and compares them as a whole. It has significant advantages at a descriptive and exploratory level and may help detect patterns that conventional methods overlook. As availability of longitudinal data increases, this becomes a significant advantage.
SA hinges on defining measures of similarity between sequences, typically to generate data-driven classifications, for example by cluster analysis. Most SA uses the optimal matching distance, but other measures are used. There is some controversy about the applicability of SA algorithms to social science data and about their parameterization. Comparison of different methods and parameterizations helps clarify the issues.
For a long time, TDA was the only package social scientists had access to for SA, but in more recent years, both Stata and R have had relevant functionality; in Stata’s case, this is provided by the SQ and SADI packages.
In this talk, I will discuss the current state of the SADI package. SADI differs from SQ that is based on a plugin; therefore, it is significantly faster: many of the distance measures are computationally intensive, and typically, _N*(_N-1)/2 comparisons will be made. It also provides additional distance measures, including dynamic hamming, time-warp edit distance, and a version of Elzinga’s number of matching subsequences measure. It includes tools for inspecting and graphing sequence data and for comparing distance measures and the resulting cluster analyses.
I will also briefly discuss the advantages and disadvantages of using plugins rather than Mata and comment about cross-compiling plugins under Linux.
Additional materials:
de14_halpin.pdf
Additional materials:
de14_drukker_gsem.pdf
Additional materials:
de14_kohler.pdf
Additional materials:
de14_erhardt_kuenster.pdf
During the past decades, count-data models (in particular, Poisson and negative-binomial-based regression models) have gained relevance in empirical social research. While identifying and interpreting main effects is relatively straightforward for this class of models, the integration of interactions between predictors proves to be complex. As a consequence of the exponential mean function implemented in count-data models (which restricts the possible range of the conditional expected count to nonnegative values), the coefficient of the product term variable (generated by the predictors constituting the interaction) does not—in contrast to the linear model—fully represent the underlying interaction effect. Further, the interaction effect is allowed to vary between individuals and can be divided into two components: a model-inherent interaction effect and a product-term-induced interaction effect.
We will derive the total interaction effect for the Poisson and negative binomial models by following a method developed by Norton and Ai (2003) for binary logit and probit models. Further, we will decompose the model-inherent and the product-term-induced interaction effect, discuss their substantive meaning, and provide delta-method standard errors for the respective effects. Finally, we will provide an approach for the estimation and graphical representation of these effects in Stata.
Reference
Additional materials:
de14_leitgoeb.pdf
Additional materials:
de14_drukker_teintro.pdf
Writing a document that contains statistical results in its narrative, including inline results, can take too much effort. Typically, users have a separate series of do-files whose results must then be pulled into the document. This is a very high-maintenance way to work in because updates to the data, changes to the do-files, updates to the statistical software, and, especially, updates to inline results all require work and careful checking of results.
Reproducible research greatly lessens document-maintenance chores by putting code and results directly into the document; this means that only one document is used; thus it remains consistent and is easily maintained.
In this presentation, I will show you how to put Stata code directly into a LaTeX or HTML document and run it through a preprocessor to create the document containing results. While this is useful for creating self-contained documents, it is very useful for creating periodic reports, class notes, solution sets, and other documents that get used over a long period of time.
Additional materials:
de14_rising.pdf
Additional materials:
de14_jann.pdf
Johannes Giesecke, University of Bamberg
[email protected]Ulrich Kohler, University of Potsdam
[email protected]Dirk Enzmann, University of Hamburg
[email protected]Kai-Uwe Schnapp, University of Hamburg
[email protected]
The conference is sponsored and organized by
Dittrich & Partner Consulting GmbH
(dpc-software.de), the distributor of Stata in
several countries, including Germany, the Netherlands, Austria, Czech
Republic, and Hungary.