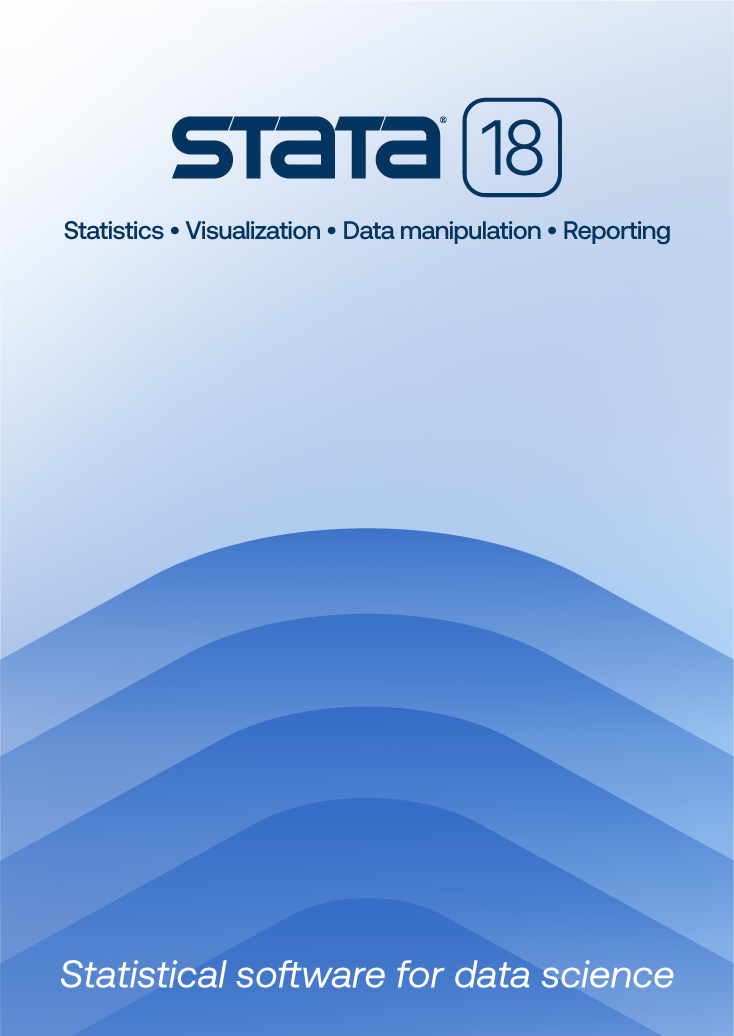
Principles of Econometrics, Fifth Edition |
||||||||||||||||||||||||||||||||
![]() Click to enlarge See the back cover |
![]()
As an Amazon Associate, StataCorp earns a small referral credit from
qualifying purchases made from affiliate links on our site.
eBook not available for this title
eBook not available for this title |
|
||||||||||||||||||||||||||||||
Comment from the Stata technical groupPrinciples of Econometrics, Fifth Edition, by R. Carter Hill, William E. Griffiths, and Guay C. Lim, is an introductory book for undergraduate econometrics. This book exemplifies learning by doing and gets the reader working through examples as fast as possible with a minimum of theory. Although Principles of Econometrics is designed to be the textbook in a principles of econometrics course, the style and coverage make it useful background reading for higher-level courses. The authors cover a broad area of econometrics. Appendixes quickly review the required mathematical, probability, and elementary-statistics tools, and a Probability Primer provides extra exercises. The first seven chapters cover estimation and inference in linear models without using matrix algebra. The next two chapters cover heteroskedasticity and stationary time series. Chapters 10 and 11 cover the method of moments approach to least-squares and instrumental-variables estimators and their application in simultaneous-equation models. Chapters 12, 13, and 14 provide nice introductions to the advanced time-series topics of nonstationarity, multiple time series, and time-varying volatility. Chapters 15 and 16 introduce two advanced topics in microeconometrics: panel-data models and models for qualitative and limited dependent variables. The fifth edition discusses the case of random covariates much earlier than the previous edition, which allows several of the first twelve chapters to be significantly streamlined. New in the fifth edition are discussions of the bootstrap in chapter 5 and a discussion of potential-outcome treatment effects in chapter 7. The numerous, nicely discussed examples in this book make the hands-on approach work well. The level of abstraction is held to a minimum, and instruction proceeds by interpreting examples. The many excellent exercises will help interested readers gain experience in and understanding about the methods discussed in the text. |
||||||||||||||||||||||||||||||||
Table of contentsView table of contents >> Preface
List of Examples
1 An Introduction to Econometrics
1.1 Why Study Econometrics?
1.2 What Is Econometrics About?
1.2.1 Some Examples
1.3 The Econometric Model
1.3.1 Causality and Prediction
1.4 How Are Data Generated?
1.4.1 Experimental Data
1.5 Economic Data Types1.4.2 Quasi-Experimental Data 1.4.3 Nonexperimental Data
1.5.1 Time-Series Data
1.6 The Research Process1.5.2 Cross-Section Data 1.5.3 Panel or Longitudinal Data 1.7 Writing an Empirical Research Paper
1.7.1 Writing a Research Proposal
1.8 Sources of Economic Data1.7.2 A Format for Writing a Research Project
1.8.1 Links to Economic Data on the Internet
1.8.2 Interpreting Economic Data 1.8.3 Obtaining the Data Probability Primer
P.1 Random Variables
P.2 Probability Distributions P.3 Joint, Marginal, and Conditional Probabilities
P.3.1 Marginal Distributions
P.4 A Digression: Summation NotationP.3.2 Conditional Probability P.3.3 Statistical Independence P.5 Properties of Probability Distributions
P.5.1 Expected Value of a Random Variable
P.6 ConditioningP.5.2 Conditional Expectation P.5.3 Rules for Expected Values P.5.4 Variance of a Random Variable P.5.5 Expected Values of Several Random Variables P.5.6 Covariance Between Two Random Variables
P.6.1 Conditional Expectation
P.7 The Normal DistributionP.6.2 Conditional Variance P.6.3 Iterated Expectations P.6.4 Variance Decomposition P.6.5 Covariance Decomposition
P.7.1 The Bivariate Normal Distribution
P.8 Exercises
2 The Simple Linear Regression Model
2.1 An Economic Model
2.2 An Econometric Model
2.2.1 Data Generating Process
2.3 Estimating the Regression Parameters2.2.2 The Random Error and Strict Exogeneity 2.2.3 The Regression Function 2.2.4 Random Error Variation 2.2.5 Variation in x 2.2.6 Error Normality 2.2.7 Generalizing the Exogeneity Assumption 2.2.8 Error Correlation 2.2.9 Summarizing the Assumptions
2.3.1 The Least Squares Principle
2.4 Assessing the Least Squares Estimators2.3.2 Other Economic Models
2.4.1 The Estimator b2
2.5 The Gauss–Markov Theorem2.4.2 The Expected Values of b1 and b2 2.4.3 Sampling Variation 2.4.4 The Variances and Covariance of b1 and b2 2.6 The Probability Distributions of the Least Squares Estimators 2.7 Estimating the Variance of the Error Term
2.7.1 Estimating the Variances and Covariance of the Least Squares Estimators
2.8 Estimating Nonlinear Relationships2.7.2 Interpreting the Standard Errors
2.8.1 Quadratic Functions
2.9 Regression with Indicator Variables2.8.2 Using a Quadratic Model 2.8.3 A Log-Linear Function 2.8.4 Using a Log-Linear Model 2.8.5 Choosing a Functional Form 2.10 The Independent Variable
2.10.1 Random and Independent x
2.11 Exercises2.10.2 Random and Strictly Exogenous x 2.10.3 Random Sampling
2.11.1 Problems
Appendix 2A Derivation of the Least Squares Estimates2.11.2 Computer Exercises Appendix 2B Deviation from the Mean Form of b2 Appendix 2C b2 Is a Linear Estimator Appendix 2D Derivation of Theoretical Expression for b2 Appendix 2E Deriving the Conditional Variance of b2 Appendix 2F Proof of the Gauss–Markov Theorem Appendix 2G Proofs of Results Introduced in Section 2.10
2G.1 The Implications of Strict Exogeneity
Appendix 2H Monte Carlo Simulation2G.2 The Random and Independent x Case 2G.3 The Random and Strictly Exogenous x Case 2G.4 Random Sampling
2H.1 The Regression Function
2H.2 The Random Error 2H.3 Theoretically True Values 2H.4 Creating a Sample of Data 2H.5 Monte Carlo Objectives 2H.6 Monte Carlo Results 2H.7 Random-x Monte Carlo Results 3 Interval Estimation and Hypothesis Testing
3.1 Interval Estimation
3.1.1 The t-Distribution
3.2 Hypothesis Tests3.1.2 Obtaining Interval Estimates 3.1.3 The Sampling Context
3.2.1 The Null Hypothesis
3.3 Rejection Regions for Specific Alternatives3.2.2 The Alternative Hypothesis 3.2.3 The Test Statistic 3.2.4 The Rejection Region 3.2.5 A Conclusion
3.3.1 One-Tail Tests with Alternative “Greater Than” (>)
3.4 Examples of Hypothesis Tests3.3.2 One-Tail Tests with Alternative “Less Than” (<) 3.3.3 Two-Tail Tests with Alternative “Not Equal To” (≠) 3.5 The p-Value 3.6 Linear Combinations of Parameters
3.6.1 Testing a Linear Combination of Parameters
3.7 Exercises
3.7.1 Problems
Appendix 3A Derivation of the t-Distribution3.7.2 Computer Exercises Appendix 3B Distribution of the t-Statistic under H1 Appendix 3C Monte Carlo Simulation
3C.1 Sampling Properties of Interval Estimators
3C.2 Sampling Properties of Hypothesis Tests 3C.3 Choosing the Number of Monte Carlo Samples 3C.4 Random-x Monte Carlo Results 4 Prediction, Goodness-of-Fit, and Modeling Issues
4.1 Least Squares Prediction
4.2 Measuring Goodness-of-Fit
4.2.1 Correlation Analysis
4.3 Modeling Issues4.2.2 Correlation Analysis and R2
4.3.1 The Effects of Scaling the Data
4.4 Polynomial Models4.3.2 Choosing a Functional Form 4.3.3 A Linear-Log Food Expenditure Model 4.3.4 Using Diagnostic Residual Plots 4.3.5 Are the Regression Errors Normally Distributed? 4.3.6 Identifying Influential Observations
4.4.1 Quadratic and Cubic Equations
4.5 Log-Linear Models
4.5.1 Prediction in the Log-Linear Model
4.6 Log-Log Models4.5.2 A Generalized R2 Measure 4.5.3 Prediction Intervals in the Log-Linear Model 4.7 Exercises
4.7.1 Problems
Appendix 4A Development of a Prediction Interval4.7.2 Computer Exercises Appendix 4B The Sum of Squares Decomposition Appendix 4C Mean Squared Error: Estimation and Prediction 5 The Multiple Regression Model
5.1 Introduction
5.1.1 The Economic Model
5.2 Estimating the Parameters of the Multiple Regression Model5.1.2 The Econometric Model 5.1.3 The General Model 5.1.4 Assumptions of the Multiple Regression Model
5.2.1 Least Squares Estimation Procedure
5.3 Finite Sample Properties of the Least Squares Estimator5.2.2 Estimating the Error Variance σ2 5.2.3 Measuring Goodness-of-Fit 5.2.4 Frisch–Waugh–Lovell (FWL) Theorem
5.3.1 The Variances and Covariances of the Least Squares Estimators
5.4 Interval Estimation5.3.2 The Distribution of the Least Squares Estimators
5.4.1 Interval Estimation for a Single Coefficient
5.5 Hypothesis Testing5.4.2 Interval Estimation for a Linear Combination of Coefficients
5.5.1 Testing the Significance of a Single Coefficient
5.6 Nonlinear Relationships5.5.2 One-Tail Hypothesis Testing for a Single Coefficient 5.5.3 Hypothesis Testing for a Linear Combination of Coefficients 5.7 Large Sample Properties of the Least Squares Estimator
5.7.1 Consistency
5.8 Exercises5.7.2 Asymptotic Normality 5.7.3 Relaxing Assumptions 5.7.4 Inference for a Nonlinear Function of Coefficients
5.8.1 Problems
Appendix 5A Derivation of Least Squares Estimators5.8.2 Computer Exercises Appendix 5B The Delta Method
5B.1 Nonlinear Function of a Single Parameter
Appendix 5C Monte Carlo Simulation5B.2 Nonlinear Function of Two Parameters
5C.1 Least Squares Estimation with Chi-Square Errors
Appendix 5D Bootstrapping5C.2 Monte Carlo Simulation of the Delta Method
5D.1 Resampling
5D.2 Bootstrap Bias Estimate 5D.3 Bootstrap Standard Error 5D.4 Bootstrap Percentile Interval Estimate 5D.5 Asymptotic Refinement 6 Further Inference in the Multiple Regression Model
6.1 Testing Joint Hypotheses: The F-test
6.1.1 Testing the Significance of the Model
6.2 The Use of Nonsample Information6.1.2 The Relationship Between t- and F-Tests 6.1.3 More General F-Tests 6.1.4 Using Computer Software 6.1.5 Large Sample Tests 6.3 Model Specification
6.3.1 Causality versus Prediction
6.4 Prediction6.3.2 Omitted Variables 6.3.3 Irrelevant Variables 6.3.4 Control Variables 6.3.5 Choosing a Model 6.3.6 RESET
6.4.1 Predictive Model Selection Criteria
6.5 Poor Data, Collinearity, and Insignificance
6.5.1 The Consequences of Collinearity
6.6 Nonlinear Least Squares6.5.2 Identifying and Mitigating Collinearity 6.5.3 Investigating Influential Observations 6.7 Exercises
6.7.1 Problems
Appendix 6A The Statistical Power of F-Tests6.7.2 Computer Exercises Appendix 6B Further Results from the FWL Theorem 7 Using Indicator Variables
7.1 Indicator Variables
7.1.1 Intercept Indicator Variables
7.2 Applying Indicator Variables7.1.2 Slope-Indicator Variables
7.2.1 Interactions Between Qualitative Factors
7.3 Log-Linear Models7.2.2 Qualitative Factors with Several Categories 7.2.3 Testing the Equivalence of Two Regressions 7.2.4 Controlling for Time
7.3.1 A Rough Calculation
7.4 The Linear Probability Model7.3.2 An Exact Calculation 7.5 Treatment Effects
7.5.1 The Difference Estimator
7.6 Treatment Effects and Causal Modeling7.5.2 Analysis of the Difference Estimator 7.5.3 The Differences-in-Differences Estimator
7.6.1 The Nature of Causal Effects
7.6.2 Treatment Effect Models 7.6.3 Decomposing the Treatment Effect 7.6.4 Introducing Control Variables 7.6.5 The Overlap Assumption 7.6.6 Regression Discontinuity Designs 7.7 Exercises
7.7.1 Problems
Appendix 7A Details of Log-Linear Model Interpretation7.7.2 Computer Exercises Appendix 7B Derivation of the Differences-in-Differences Estimator Appendix 7C The Overlap Assumption: Details 8 Heteroskedasticity
8.1 The Nature of Heteroskedasticity
8.2 Heteroskedasticity in the Multiple Regression Model
8.2.1 The Heteroskedastic Regression Model
8.3 Heteroskedasticity Robust Variance Estimator8.2.2 Heteroskedasticity Consequences for the OLS Estimator 8.4 Generalized Least Squares: Known Form of Variance
8.4.1 Transforming the Model: Proportional Heteroskedasticity
8.5 Generalized Least Squares: Unknown Form of Variance8.4.2 Weighted Least Squares: Proportional Heteroskedasticity
8.5.1 Estimating the Multiplicative Model
8.6 Detecting Heteroskedasticity
8.6.1 Residual Plots
8.7 Heteroskedasticity in the Linear Probability Model8.6.2 The Goldfeld–Quandt Test 8.6.3 A General Test for Conditional Heteroskedasticity 8.6.4 The White Test 8.6.5 Model Specification and Heteroskedasticity 8.8 Exercises
8.8.1 Problems
Appendix 8A Properties of the Least Squares Estimator8.8.2 Computer Exercises Appendix 8B Lagrange Multiplier Tests for Heteroskedasticity Appendix 8C Properties of the Least Squares Residuals
8C.1 Details of Multiplicative Heteroskedasticity Model
Appendix 8D Alternative Robust Sandwich EstimatorsAppendix 8E Monte Carlo Evidence: OLS, GLS, and FGLS 9 Regression with Time-Series Data: Stationary Variables
9.1 Introduction
9.1.1 Modeling Dynamic Relationships
9.2 Stationarity and Weak Dependence9.1.2 Autocorrelations 9.3 Forecasting
9.3.1 Forecast Intervals and Standard Errors
9.4 Testing for Serially Correlated Errors9.3.2 Assumptions for Forecasting 9.3.3 Selecting Lag Lengths 9.3.4 Testing for Granger Causality
9.4.1 Checking the Correlogram of the Least Squares Residuals
9.5 Time-Series Regressions for Policy Analysis9.4.2 Lagrange Multiplier Test 9.4.3 Durbin–Watson Test
9.5.1 Finite Distributed Lags
9.6 Exercises9.5.2 HAC Standard Errors 9.5.3 Estimation with AR(1) Errors 9.5.4 Infinite Distributed Lags
9.6.1 Problems
Appendix 9A The Durbin–Watson Test9.6.2 Computer Exercises
9A.1 The Durbin–Watson Bounds Test
Appendix 9B Properties of an AR(1) Error10 Endogenous Regressors and Moment-Based Estimation
10.1 Least Squares Estimation with Endogenous Regressors
10.1.1 Large Sample Properties of the OLS Estimator
10.2 Cases in Which x and e are Contemporaneously Correlated10.1.2 Why Least Squares Estimation Fails 10.1.3 Proving the Inconsistency of OLS
10.2.1 Measurement Error
10.3 Estimators Based on the Method of Moments10.2.2 Simultaneous Equations Bias 10.2.3 Lagged-Dependent Variable Models with Serial Correlation 10.2.4 Omitted Variables
10.3.1 Method of Moments Estimation of a Population Mean and Variance
10.4 Specification Tests10.3.2 Method of Moments Estimation in the Simple Regression Model 10.3.3 Instrumental Variables Estimation in the Simple Regression Model 10.3.4 The Importance of Using Strong Instruments 10.3.5 Proving the Consistency of the IV Estimator 10.3.6 IV Estimation Using Two-Stage Least Squares (2SLS) 10.3.7 Using Surplus Moment Conditions 10.3.8 Instrumental Variables Estimation in the Multiple Regression Model 10.3.9 Assessing Instrument Strength Using the First-Stage Model 10.3.10 Instrumental Variables Estimation in a General Model 10.3.11 Additional Issues When Using IV Estimation
10.4.1 The Hausman Test for Endogeneity
10.5 Exercises10.4.2 The Logic of the Hausman Test 10.4.3 Testing Instrument Validity
10.5.1 Problems
Appendix 10A Testing for Weak Instruments10.5.2 Computer Exercises
10A.1 A Test for Weak Identification
Appendix 10B Monte Carlo Simulation10A.2 Testing for Weak Identification: Conclusions
10B.1 Illustrations Using Simulated Data
10B.2 The Sampling Properties of IV/2SLS 11 Simultaneous Equations Models
11.1 A Supply and Demand Model
11.2 The Reduced-Form Equations 11.3 The Failure of Least Squares Estimation
11.3.1 Proving the Failure of OLS
11.4 The Identification Problem11.5 Two-Stage Least Squares Estimation
11.5.1 The General Two-Stage Least Squares Estimation Procedure
11.6 Exercises11.5.2 The Properties of the Two-Stage Least Squares Estimator
11.6.1 Problems
Appendix 11A 2SLS Alternatives11.6.2 Computer Exercises
11A.1 The k-Class of Estimators
11A.2 The LIML Estimator 11A.3 Monte Carlo Simulation Results 12 Regression with Time-Series Data: Nonstationary Variables
12.1 Stationary and Nonstationary Variables
12.1.1 Trend Stationary Variables
12.2 Consequences of Stochastic Trends12.1.2 The First-Order Autoregressive Model 12.1.3 Random Walk Models 12.3 Unit Root Tests for Stationarity
12.3.1 Unit Roots
12.4 Cointegration12.3.2 Dickey–Fuller Tests 12.3.3 Dickey–Fuller Test with Intercept and No Trend 12.3.4 Dickey–Fuller Test with Intercept and Trend 12.3.5 Dickey–Fuller Test with No Intercept and No Trend 12.3.6 Order of Integration 12.3.7 Other Unit Root Tests
12.4.1 The Error Correction Model
12.5 Regression When There Is No Cointegration12.6 Summary 12.7 Exercises
12.7.1 Problems
12.7.2 Computer Exercises 13 Vector Error Correction and Vector Autoregressive Models
13.1 VEC and VAR Models
13.2 Estimating a Vector Error Correction Model 13.3 Estimating a VAR Model 13.4 Impulse Responses and Variance Decompositions
13.4.1 Impulse Response Functions
13.5 Exercises13.4.2 Forecast Error Variance Decompositions
13.5.1 Problems
Appendix 13A The Identification Problem13.5.2 Computer Exercises 14 Time-Varying Volatility and ARCH Models
14.1 The ARCH Model
14.2 Time-Varying Volatility 14.3 Testing, Estimating, and Forecasting 14.4 Extensions
14.4.1 The GARCH Model—Generalized ARCH
14.5 Exercises14.4.2 Allowing for an Asymmetric Effect 14.4.3 GARCH-in-Mean and Time-Varying Risk Premium 14.4.4 Other Developments
14.5.1 Problems
14.5.2 Computer Exercises 15 Panel Data Models
15.1 The Panel Data Regression Function
15.1.1 Further Discussion of Unobserved Heterogeneity
15.2 The Fixed Effects Estimator15.1.2 The Panel Data Regression Exogeneity Assumption 15.1.3 Using OLS to Estimate the Panel Data Regression
15.2.1 The Difference Estimator: T = 2
15.3 Panel Data Regression Error Assumptions15.2.2 The Within Estimator: T = 2 15.2.3 The Within Estimator: T > 2 15.2.4 The Least Squares Dummy Variable Model
15.3.1 OLS Estimation with Cluster-Robust Standard Errors
15.4 The Random Effects Estimator15.3.2 Fixed Effects Estimation with Cluster-Robust Standard Errors
15.4.1 Testing for Random Effects
15.5 Exercises15.4.2 A Hausman Test for Endogeneity in the Random Effects Model 15.4.3 A Regression-Based Hausman Test 15.4.4 The Hausman–Taylor Estimator 15.4.5 Summarizing Panel Data Assumptions 15.4.6 Summarizing and Extending Panel Data Model Estimation
15.5.1 Problems
Appendix 15A Cluster-Robust Standard Errors: Some Details15.5.2 Computer Exercises Appendix 15B Estimation of Error Components 16 Qualitative and Limited Dependent Variable Models
16.1 Introducing Models with Binary Dependent Variables
16.1.1 The Linear Probability Model
16.2 Modeling Binary Choices
16.2.1 The Probit Model for Binary Choice
16.3 Multinomial Logit16.2.2 Interpreting the Probit Model 16.2.3 Maximum Likelihood Estimation of the Probit Model 16.2.4 The Logit Model for Binary Choices 16.2.5 Wald Hypothesis Tests 16.2.6 Likelihood Ratio Hypothesis Tests 16.2.7 Robust Inference in Probit and Logit Models 16.2.8 Binary Choice Models with a Continuous Endogenous Variable 16.2.9 Binary Choice Models with a Binary Endogenous Variable 16.2.10 Binary Endogenous Explanatory Variables 16.2.11 Binary Choice Models and Panel Data
16.3.1 Multinomial Logit Choice Probabilities
16.4 Conditional Logit16.3.2 Maximum Likelihood Estimation 16.3.3 Multinomial Logit Postestimation Analysis
16.4.1 Conditional Logit Choice Probabilities
16.5 Ordered Choice Models16.4.2 Conditional Logit Postestimation Analysis
16.5.1 Ordinal Probit Choice Probabilities
16.6 Models for Count Data16.5.2 Ordered Probit Estimation and Interpretation
16.6.1 Maximum Likelihood Estimation of the Poisson Regression Model
16.7 Limited Dependent Variables16.6.2 Interpreting the Poisson Regression Model
16.7.1 Maximum Likelihood Estimation of the Simple Linear Regression Model
16.8 Exercises16.7.2 Truncated Regression 16.7.3 Censored Samples and Regression 16.7.4 Tobit Model Interpretation 16.7.5 Sample Selection
16.8.1 Problems
Appendix 16A Probit Marginal Effects: Details16.8.2 Computer Exercises
16A.1 Standard Error of Marginal Effect at a Given Point
Appendix 16B Random Utility Models16A.2 Standard Error of Average Marginal Effect
16B.1 Binary Choice Model
Appendix 16C Using Latent Variables16B.2 Probit or Logit?
16C.1 Tobit (Tobit Type I)
Appendix 16D A Tobit Monte Carlo Experiment16C.2 Heckit (Tobit Type II) Appendix A Mathematical Tools
A.1 Some Basics
A.1.1 Numbers
A.2 Linear RelationshipsA.1.2 Exponents A.1.3 Scientific Notation A.1.4 Logarithms and the Number e A.1.5 Decimals and Percentages A.1.6 Logarithms and Percentages
A.2.1 Slopes and Derivatives
A.3 Nonlinear RelationshipsA.2.2 Elasticity
A.3.1 Rules for Derivatives
A.4 IntegralsA.3.2 Elasticity of a Nonlinear Relationship A.3.3 Second Derivatives A.3.4 Maxima and Minima A.3.5 Partial Derivatives A.3.6 Maxima and Minima of Bivariate Functions
A.4.1 Computing the Area Under a Curve
A.5 ExercisesAppendix B Probability Concepts
B.1 Discrete Random Variables
B.1.1 Expected Value of a Discrete Random Variable
B.2 Working with Continuous Random VariablesB.1.2 Variance of a Discrete Random Variable B.1.3 Joint, Marginal, and Conditional Distributions B.1.4 Expectations Involving Several Random Variables B.1.5 Covariance and Correlation B.1.6 Conditional Expectations B.1.7 Iterated Expectations B.1.8 Variance Decomposition B.1.9 Covariance Decomposition
B.2.1 Probability Calculations
B.3 Some Important Probability DistributionsB.2.2 Properties of Continuous Random Variables B.2.3 Joint, Marginal, and Conditional Probability Distributions B.2.4 Using Iterated Expectations with Continuous Random Variables B.2.5 Distributions of Functions of Random Variables B.2.6 Truncated Random Variables
B.3.1 The Bernoulli Distribution
B.4 Random NumbersB.3.2 The Binomial Distribution B.3.3 The Poisson Distribution B.3.4 The Uniform Distribution B.3.5 The Normal Distribution B.3.6 The Chi-Square Distribution B.3.7 The t-distribution B.3.8 The F-distribution B.3.9 The Log-Normal Distribution
B.4.1 Uniform Random Numbers
B.5 ExercisesAppendix C Review of Statistical Inference
C.1 A Sample of Data
C.2 An Econometric Model C.3 Estimating the Mean of a Population
C.3.1 The Expected Value of Ȳ
C.4 Estimating the Population Variance and Other MomentsC.3.2 The Variance of Ȳ C.3.3 The Sampling Distribution of Ȳ C.3.4 The Central Limit Theorem C.3.5 Best Linear Unbiased Estimation
C.4.1 Estimating the Population Variance
C.5 Interval EstimationC.4.2 Estimating Higher Moments
C.5.1 Interval Estimation: σ2 Known
C.6 Hypothesis Tests About a Population MeanC.5.2 Interval Estimation: σ2 Unknown
C.6.1 Components of Hypothesis Tests
C.7 Some Other Useful TestsC.6.2 One-Tail Tests with Alternative “Greater Than” (>) C.6.3 One-Tail Tests with Alternative “Less Than” (<) C.6.4 Two-Tail Tests with Alternative “Not Equal To” (≠) C.6.5 The p-Value C.6.6 A Comment on Stating Null and Alternative Hypotheses C.6.7 Type I and Type II Errors C.6.8 A Relationship Between Hypothesis Testing and Confidence Intervals
C.7.1 Testing the Population Variance
C.8 Introduction to Maximum Likelihood EstimationC.7.2 Testing the Equality of Two Population Means C.7.3 Testing the Ratio of Two Population Variances C.7.4 Testing the Normality of a Population
C.8.1 Inference with Maximum Likelihood Estimators
C.9 Algebraic SupplementsC.8.2 The Variance of the Maximum Likelihood Estimator C.8.3 The Distribution of the Sample Proportion C.8.4 Asymptotic Test Procedures
C.9.1 Derivation of Least Squares Estimator
C.10 Kernel Density EstimatorC.9.2 Best Linear Unbiased Estimation C.11 Exercises
C.11.1 Problems
C.11.2 Computer Exercises Appendix D Statistical Tables
Table D.1 Cumulative Probabilities for the Standard Normal Distribution Φ(z) = P(Z ≤ z)
Table D.2 Percentiles of the t-distribution Table D.3 Percentiles of the Chi-square Distribution Table D.4 95th Percentile for the F-distribution Table D.5 99th Percentile for the F-distribution Table D.6 Standard Normal pdf Values Φ(z) Index
|
Learn
Free webinars
NetCourses
Classroom and web training
Organizational training
Video tutorials
Third-party courses
Web resources
Teaching with Stata
© Copyright 1996–2024 StataCorp LLC. All rights reserved.
×
We use cookies to ensure that we give you the best experience on our website—to enhance site navigation, to analyze usage, and to assist in our marketing efforts. By continuing to use our site, you consent to the storing of cookies on your device and agree to delivery of content, including web fonts and JavaScript, from third party web services.
Cookie Settings
Last updated: 16 November 2022
StataCorp LLC (StataCorp) strives to provide our users with exceptional products and services. To do so, we must collect personal information from you. This information is necessary to conduct business with our existing and potential customers. We collect and use this information only where we may legally do so. This policy explains what personal information we collect, how we use it, and what rights you have to that information.
These cookies are essential for our website to function and do not store any personally identifiable information. These cookies cannot be disabled.
This website uses cookies to provide you with a better user experience. A cookie is a small piece of data our website stores on a site visitor's hard drive and accesses each time you visit so we can improve your access to our site, better understand how you use our site, and serve you content that may be of interest to you. For instance, we store a cookie when you log in to our shopping cart so that we can maintain your shopping cart should you not complete checkout. These cookies do not directly store your personal information, but they do support the ability to uniquely identify your internet browser and device.
Please note: Clearing your browser cookies at any time will undo preferences saved here. The option selected here will apply only to the device you are currently using.