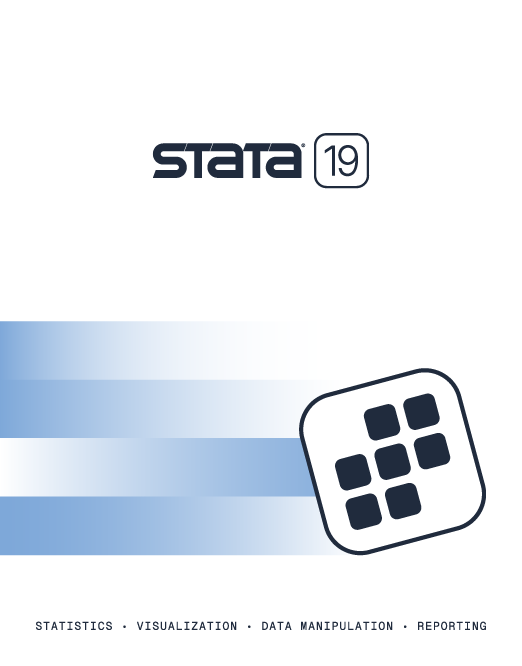
2025 Stata Conference • Nashville, TN • 31 July–01 August
Introduction to Econometrics, Fourth Edition |
||||||||||||||||||||||||||||||||
![]() Click to enlarge See the back cover |
![]()
As an Amazon Associate, StataCorp earns a small referral credit from
qualifying purchases made from affiliate links on our site.
eBook not available for this title
eBook not available for this title |
|
||||||||||||||||||||||||||||||
Comment from the Stata technical groupIntroduction to Econometrics, Fourth Edition, by James H. Stock and Mark W. Watson, provides an outstanding introduction to econometrics. They use the principle that "interesting applications must motivate the theory and the theory must match the applications" to write a rigorous text that makes you want to keep reading to find out how the story ends. Using an ingenious set of real-world questions and answers, they produced an excellent introduction to estimation, inference, and interpretation in econometrics. The text makes advanced statistical concepts easily understandable. For instance, the current econometric approach to analyzing linear models combines assumptions on the conditional moments of random variables and large-sample theory to derive estimators and their properties. This textbook provides an accessible introduction to this technique and its application to cross-sectional data, panel data, and time-series regression. The text provides an excellent introduction to causal inference and to understanding the role of regression as a tool for causal inference. The fourth edition provides an excellent introduction to prediction and to some key concepts and methods used in big-data analysis and machine learning. This edition distinguishes between econometrics for causal inference and econometrics for prediction early in the text. In the new chapter on prediction with many regressors and big data, the authors discuss some essential topics in prediction, including cross validation, ride regression, and the Lasso. The coverage and level of this text make it an excellent choice for undergraduate study, as a supplement to advanced courses, or as a refresher course for researchers that want a quick introduction to modern parametric econometrics. |
||||||||||||||||||||||||||||||||
Table of contentsView table of contents >> Preface
PART ONE Introduction and Review
CHAPTER 1 Economic Questions and Data
1.1 Economic Questions We Examine
Question 1: Does Reducing Class Size Improve Elementary School Education?
1.2 Causal Effects and Idealized ExperimentsQuestion 2: Is There Racial Discrimination in the Market for Home Loans? Question 3: How Much Do Cigarette Taxes Reduce Smoking? Question 4: By How Much Will U.S. GDP Grow Next Year? Quantitative Questions, Quantitative Answers
Estimation of Causal Effects
1.3 Data: Sources and TypesPrediction, Forecasting, and Causality
Experimental versus Observational Data
Cross-Sectional Data Time Series Data Panel Data CHAPTER 2 Review of Probability
2.1 Random Variables and Probability Distributions
Probabilities, the Sample Space, and Random Variables
2.2 Expected Values, Mean, and VarianceProbability Distribution of a Discrete Random Variable Probability Distribution of a Continuous Random Variable
The Expected Value of a Random Variable
2.3 Two Random VariablesThe Standard Deviation and Variance Mean and Variance of a Linear Function of a Random Variable Other Measures of the Shape of a Distribution Standardized Random Variables
Joint and Marginal Distributions
2.4 The Normal, Chi-Squared, Student t, and F DistributionsConditional Distributions Independence Covariance and Correlation The Mean and Variance of Sums of Random Variables
The Normal Distributions
2.5 Random Sampling and the Distribution of the Sample AverageThe Chi-Squared Distribution The Student t Distribution The F Distribution
Random Sampling
2.6 Large-Sample Approximations to the Sampling DistributionsThe Sampling Distribution of the Sample Average
The Law of Large Numbers and Consistency
The Central Limit Theorem APPENDIX 2.1 Derivation of Results in Key Concept 2.3 APPENDIX 2.2 The Conditional Mean as the Minimum Mean Squared Error Predictor CHAPTER 3 Review of Statistics
3.1 Estimation of the Population Mean
Estimators and Their Properties
3.2 Hypothesis Tests Concerning the Population MeanProperties of Ȳ The Importance of Random Sampling
Null and Alternative Hypotheses
3.3 Confidence Intervals for the Population MeanThe p-Value Calculating the p-Value When σϒ Is Known The Sample Variance, Sample Standard Deviation, and Standard Error Calculating the p-Value When σϒ Is Unknown The t-Statistic Hypothesis Testing with a Prespecified Significance Level One-Sided Alternatives 3.4 Comparing Means from Different Populations
Hypothesis Tests for the Difference Between Two Means
3.5 Differences-of-Means Estimation of Causal Effects Using Experimental DataConfidence Intervals for the Difference Between Two Population Means
The Causal Effect as a Difference of Conditional Expectations
3.6 Using the t-Statistic When the Sample Size Is SmallEstimation of the Causal Effect Using Differences of Means
The t-Statistic and the Student t Distribution
3.7 Scatterplots, the Sample Covariance, and the Sample CorrelationUse of the Student t Distribution in Practice
Scatterplots
Sample Covariance and Correlation APPENDIX 3.1 The U.S. Current Population Survey APPENDIX 3.2 Two Proofs That Ȳ Is the Least Squares Estimator of μϒ APPENDIX 3.3 A Proof That the Sample Variance is Consistent PART TWO Fundamentals of Regression Analysis
CHAPTER 4 Linear Regression with One Regressor
4.1 The Linear Regression Model
4.2 Estimating the Coefficients of the Linear Regression Model
The Ordinary Least Squares Estimator
4.3 Measures of Fit and Prediction AccuracyOLS Estimates of the Relationship Between Test Scores and the Student–Teacher Ratio Why Use the OLS Estimator?
The R2
4.4 The Least Squares AssumptionsThe Standard Error of the Regression Prediction Using OLS Application to the Test Score Data
Assumption 1: The Conditional Distribution of ui Given Xi Has a Mean of Zero
4.5 Sampling Distribution of the OLS EstimatorsAssumption 2: (Xi, Xi) i = 1,…, n, Are Independently and Identically Distributed Assumption 3: Large Outliers Are Unlikely Use of the Least Squares Assumptions 4.6 Conclusion
APPENDIX 4.1 The California Test Score Data Set
APPENDIX 4.2 Derivation of the OLS Estimators APPENDIX 4.3 Sampling Distribution of the OLS Estimator APPENDIX 4.4 The Least Squares Assumptions for Prediction CHAPTER 5 Regression with a Single Regressor: Hypothesis Tests and Confidence Intervals
5.1 Testing Hypotheses About One of the Regression Coefficients
Two-Sided Hypotheses Concerning Β1
5.2 Confidence Intervals for a Regression CoefficientOne-Sided Hypotheses Concerning Β1 Testing Hypotheses About the Intercept Β0 5.3 Regression When X is a Binary Variable
Interpretation of the Regression Coefficients
5.4 Heteroskedasticity and Homoskedasticity
What Are Heteroskedasticity and Homoskedasticity?
5.5 The Theoretical Foundations of Ordinary Least SquaresMathematical Implications of Homoskedasticity What Does This Mean in Practice
Linear Conditionally Unbiased Estimators and the Gauss–Markov Theorem
5.6 Using the t-Statistic in Regression When the Sample Size Is SmallRegression Estimators Other Than OLS
The t-Statistic and the Student t Distribution
5.7 ConclusionUse of the Student t Distribution in Practice
APPENDIX 5.1 Formulas for OLS Standard Errors
APPENDIX 5.2 The Gauss–Markov Conditions and a Proof of the Gauss–Markov Theorem CHAPTER 6 Linear Regression with Multiple Regressors
6.1 Omitted Variable Bias
Definition of Omitted Variable Bias
6.2 The Multiple Regression ModelA Formula for Omitted Variable Bias Addressing Omitted Variable Bias by Dividing the Data into Groups
The Population Regression Line
6.3 The OLS Estimator in Multiple RegressionThe Population Multiple Regression Model
The OLS Estimator
6.4 Measures of Fit in Multiple RegressionApplication to Test Scores and the Student–Teacher Ratio
The Standard Error of the Regression (SER)
6.5 The Least Squares Assumptions for Causal Inference in Multiple RegressionThe R2 The Adjusted R2 Application to Test Scores
Assumption 1: The Conditional Distribution of ui
Given X1i ′,
X2i ′,…,
Xki Has a Mean of 0
6.6 The Distribution of OLS Estimators in Multiple RegressionAssumption 2: (X1i ′, X2i ′,…, Xki,Yi), i = 1,…,n, Are i.i.d. Assumption 3: Large Outliers Are Unlikely Assumption 4: No Perfect Multicollinearity 6.7 Multicollinearity
Examples of Perfect Multicollinearity
6.8 Control Variables and Conditional Mean IndependenceImperfect Multicollinearity 6.9 Conclusion
APPENDIX 6.1 Derivation of Equation (6.1)
APPENDIX 6.2 Distribution of the OLS Estimators When There Are Two Regressors and Homoskedastic Errors APPENDIX 6.3 The Frisch–Waugh Theorem APPENDIX 6.4 The Least Squares Assumptions for Prediction with Multiple Regressors APPENDIX 6.5 Distribution of OLS Estimators in Multiple Regression with Control Variables CHAPTER 7: Hypothesis Tests and Confidence Intervals in Multiple Regression
7.1 Hypothesis Tests and Confidence Intervals for a Single Coefficient
Standard Errors for the OLS Estimators
7.2 Tests of Joint HypothesesHypothesis Tests for a Single Coefficient Confidence Intervals for a Single Coefficient Application to Test Scores and the Student–Teacher Ratio
Testing Hypotheses on Two or More Coefficients
7.3 Testing Single Restrictions Involving Multiple CoefficientsThe F-Statistic Application to Test Scores and the Student–Teacher Ratio The Homoskedasticity-Only F-Statistic 7.4 Confidence Sets for Multiple Coefficients 7.5 Model Specification for Multiple Regression
Model Specification and Choosing Control Variables
7.6 Analysis of the Test Score Data SetInterpreting the R2 and the Adjusted R2 in Practice 7.7 Conclusion
APPENDIX 7.1 The Bonferroni Test of a Joint Hypothesis
CHAPTER 8 Nonlinear Regression Functions
8.1 A General Strategy for Modeling Nonlinear Regression Functions
Test Scores and District Income
8.2 Nonlinear Functions of a Single Independent VariableThe Effect on Y of a Change in X in Nonlinear Specifications A General Approach to Modeling Nonlinearities Using Multiple Regression
Polynomials
8.3 Interactions Between Independent VariablesLogarithms Polynomial and Logarithmic Models of Test Scores and District Income
Interactions Between Two Binary Variables
8.4 Nonlinear Effects on Test Scores of the Student–Teacher RatioInteractions Between a Continuous and a Binary Variable Interactions Between Two Continuous Variables
Discussion of Regression Results
8.5 ConclusionSummary of Findings
APPENDIX 8.1 Regression Functions That Are Nonlinear in the Parameters
APPENDIX 8.2 Slopes and Elasticities for Nonlinear Regression Functions CHAPTER 9 Assessing Studies Based on Multiple Regression
9.1 Internal and External Validity
Threats to Internal Validity
9.2 Threats to Internal Validity of Multiple Regression AnalysisThreats to External Validity
Omitted Variable Bias
9.3 Internal and External Validity When the Regression Is Used for PredictionMisspecification of the Functional Form of the Regression Function Measurement Error and Errors-in-Variables Bias Missing Data and Sample Selection Simultaneous Causality Sources of Inconsistency of OLS Standard Errors 9.4 Example: Test Scores and Class Size
External Validity
9.5 ConclusionInternal Validity Discussion and Implications
APPENDIX 9.1 The Massachusetts Elementary School Testing Data
PART THREE Further Topics in Regression Analysis
CHAPTER 10 Regression with Panel Data
10.1 Panel Data
Example: Traffic Deaths and Alcohol Taxes
10.2 Panel Data with Two Time Periods: “Before and After” Comparisons10.3 Fixed Effects Regression
The Fixed Effects Regression Model
10.4 Regression with Time Fixed EffectsEstimation and Inference Application to Traffic Deaths
Time Effects Only
10.5 The Fixed Effects Regression Assumptions and Standard Errors for Fixed Effects RegressionBoth Entity and Time Fixed Effects
The Fixed Effects Regression Assumptions
10.6 Drunk Driving Laws and Traffic DeathsStandard Errors for Fixed Effects Regression 10.7 Conclusion
APPENDIX 10.1 The State Traffic Fatality Data Set
APPENDIX 10.2 Standard Errors for Fixed Effects Regression CHAPTER 11 Regression with a Binary Dependent Variable
11.1 Binary Dependent Variables and the Linear Probability Model
Binary Dependent Variables
11.2 Probit and Logit RegressionThe Linear Probability Model
Probit Regression
11.3 Estimation and Inference in the Logit and Probit ModelsLogit Regression Comparing the Linear Probability, Probit, and Logit Models
Nonlinear Least Squares Estimation
11.4 Application to the Boston HMDA DataMaximum Likelihood Estimation Measures of Fit 11.5 Conclusion
APPENDIX 11.1 The Boston HMDA Data Set
APPENDIX 11.2 Maximum Likelihood Estimation APPENDIX 11.3 Other Limited Dependent Variable Models CHAPTER 12 Instrumental Variables Regression
12.1 The IV Estimator with a Single Regressor and a Single Instrument
The IV Model and Assumptions
12.2 The General IV Regression ModelThe Two Stage Least Squares Estimator Why Does IV Regression Work? The Sampling Distribution of the TSLS Estimator Application to the Demand for Cigarettes
TSLS in the General IV Model
12.3 Checking Instrument ValidityInstrument Relevance and Exogeneity in the General IV Model The IV Regression Assumptions and Sampling Distribution of the TSLS Estimator Inference Using the TSLS Estimator Application to the Demand for Cigarettes
Assumption 1: Instrument Relevance
12.4 Application to the Demand for CigarettesAssumption 2: Instrument Exogeneity 12.5 Where Do Valid Instruments Come From?
Three Examples
12.6 Conclusion
APPENDIX 12.1 The Cigarette Consumption Panel Data Set
APPENDIX 12.2 Derivation of the Formula for the TSLS Estimator in Equation (12.4) APPENDIX 12.3 Large-Sample Distribution of the TSLS Estimator APPENDIX 12.4 Large-Sample Distribution of the TSLS Estimator When the Instrument Is Not Valid APPENDIX 12.5 Instrumental Variables Analysis with Weak Instruments APPENDIX 12.6 TSLS with Control Variables CHAPTER 13 Experiments and Quasi-Experiments
13.1 Potential Outcomes, Causal Effects, and Idealized Experiments
Potential Outcomes and the Average Causal Effect
13.2 Threats to Validity of ExperimentsEconometric Methods for Analyzing Experimental Data
Threats to Internal Validity
13.3 Experimental Estimates of the Effect of Class Size ReductionsThreats to External Validity
Experimental Design
13.4 Quasi-ExperimentsAnalysis of the STAR Data Comparison of the Observational and Experimental Estimates of Class Size Effects
Examples
13.5 Potential Problems with Quasi-ExperimentsThe Differences-in-Differences Estimator Instrumental Variables Estimators Regression Discontinuity Estimators
Threats to Internal Validity
13.6 Experimental and Quasi-Experimental Estimates in Heterogeneous PopulationsThreats to External Validity
OLS with Heterogeneous Causal Effects
13.7 ConclusionIV Regression with Heterogeneous Causal Effects
APPENDIX 13.1 The Project STAR Data Set
APPENDIX 13.2 IV Estimation When the Causal Effect Varies Across Individuals APPENDIX 13.3 The Potential Outcomes Framework for Analyzing Data from Experiments CHAPTER 14 Prediction with Many Regressors and Big Data
14.1 What is “Big Data”?
14.2 The Many-Predictor Problem and OLS
The Mean Squared Prediction Error
14.3 Ridge Regression
The First Least Squares Assumption for Prediction The Predictive Regression Model with Standardized Regressors The MSPE of OLS and the Principle of Shrinkage Estimation of the MSPE
Shrinkage via Penalization and Ridge Regression
14.4 The LassoEstimation of the Ridge Shrinkage Parameter by Cross Validation Application to School Test Scores
Shrinkage Using the Lasso
14.5 Principal ComponentsApplication to School Test Scores
Principals Components with Two Variables
14.6 Predicting School Test Scores with Many PredictorsPrincipal Components with k Variables Application to School Test Scores 14.7 Conclusion
APPENDIX 14.1 The California School Test Score Data Set
APPENDIX 14.2 Derivation of Equation (14.4) for k =1 APPENDIX 14.3 The Ridge Regression Estimator When k =1 APPENDIX 14.4 The Lasso Estimator When k =1 APPENDIX 14.5 Computing Out-of-Sample Predictions in the Standardized Regression Model PART FOUR Regression Analysis of Economic Time Series Data
CHAPTER 15 Introduction to Time Series Regression and Forecasting
15.1 Introduction to Time Series Data and Serial Correlation
Real GDP in the United States
15.2 Stationarity and the Mean Squared Forecast ErrorLags, First Differences, Logarithms, and Growth Rates Autocorrelation Other Examples of Economic Time Series
Stationarity
15.3 AutoregressionsForecasts and Forecast Errors The Mean Squared Forecast Error
The First-Order Autoregressive Model
15.4 Time Series Regression with Additional Predictors and the Autoregressive Distributed Lag ModelThe pth-Order Autoregressive Model
Forecasting GDP Growth Using the Term Spread
15.5 Estimation of the MSFE and Forecast IntervalsThe Autoregressive Distributed Lag Model The Least Squares Assumptions for Forecasting with Multiple Predictors
Estimation of the MSFE
15.6 Estimating the Lag Length Selection Using Information CriteriaForecast Uncertainty and Forecast Intervals
Determining the Order of an Autoregression
15.7 Nonstationarity I: TrendsLag Length Selection in Time Series Regression with Multiple Predictors
What Is a Trend?
15.8 Nonstationarity II: BreaksProblems Caused by Stochastic Trends Detecting Stochastic Trends: Testing for a Unit AR Root Avoiding the Problems Caused by Stochastic Trends
What Is a Break?
15.9 ConclusionTesting for Breaks Detecting Breals Using Pseudo Out-of-Sample Forecasting Avoiding the Problems Caused by Breaks
APPENDIX 15.1 Time Series Data Used in Chapter 14
APPENDIX 15.2 Stationarity in the AR(1) Model APPENDIX 15.3 Lag Operator Notation APPENDIX 15.4 ARMA Models APPENDIX 15.5 Consistency of the BIC Lag Length Estimator CHAPTER 16 Estimation of Dynamic Causal Effects
16.1 An Initial Taste of the Orange Juice Data
16.2 Dynamic Causal Effects
Causal Effects and Time Series Data
16.3 Estimation of Dynamic Causal Effects with Exogenous RegressorsTwo Types of Exogeneity
The Distributed Lag Model Assumptions
16.4 Heteroskedasticity- and Autocorrelation-Consistent Standard ErrorsAutocorrelated ut, Standard Errors, and Inference Dynamic Multipliers and Cumulative Dynamic Multipliers
Distribution of the OLS Estimator with Autocorrelated Errors
16.5 Estimation of Dynamic Causal Effects with Strictly Exogenous RegressorsHAC Standard Errors
The Distributed Lag Model with AR(1) Errors
16.6 Orange Juice Prices and Cold WeatherOLS Estimation of the ADL Model GLS Estimation 16.7 Is Exogeneity Plausible? Some Examples
U.S. Income and Australian Exports
16.8 ConclusionOil Prices and Inflation Monetary Policy and Inflation The Growth Rate of GDP and the Term Spread
APPENDIX 16.1 The Orange Juice Data Set
APPENDIX 16.2 The ADL Model and Generalized Least Squares in Lag Operator Notation CHAPTER 17 Additional Topics in Time Series Regression
17.1 Vector Autoregressions
The VAR Model
17.2 Multi-period ForecastsA VAR Model of the Growth Rate of GDP and the Term Spread
Iterated Multi-period Forecasts
17.3 Orders of Integration and the Nonnormality of Unit Root Test StatisticsDirect Multi-period Forecasts Which Method Should You Use?
Other Models of Trends and Orders of Integration
17.4 CointegrationWhy Do Unit Root Tests Have Nonnormal Distributions?
Cointegration and Error Correction
17.5 Volatility Clustering and Autoregressive Conditional HeteroskedasticityHow Can You Tell Whether Two Variables are Cointegrated? Estimation of Cointegrating Coefficients Extension to Multiple Cointegrated Variables
Volatility Clustering
17.6 Forecasting with Many Predictors Using Dynamic Factor Models and Principal ComponentsRealized Volatility Application to Stock Price Volatility
The Dynamic Factor Model
17.7 ConclusionThe DFM: Estimation and Forecasting Application to U.S. Macroeconomic Data
APPENDIX 17.1 The Quarterly U.S. Macro Data Set
PART FIVE Regression Analysis of Economic Time Series Data
CHAPTER 18 The Theory of Linear Regression with One Regressor
18.1 The Extended Least Squares Assumptions and the OLS Estimator
The Extended Least Squares Assumptions
18.2 Fundamentals of Asymptotic Distribution TheoryThe OLS Estimator
Convergence in Probability and the Law of Large Numbers
18.3 Asymptotic Distribution of the OLS Estimator and t-StatisticThe Central Limit Theorem and Convergence in Distribution Slutsky’s Theorem and the Continuous Mapping Theorem Application to the t-Statistic Based on the Sample Mean
Consistency and Asymptotic Normality of the OLS Estimators
18.4 Exact Sampling Distributions When the Errors Are Normally DistributedConsistency of Heteroskedasticity-Robust Standard Errors Asymptotic Normality of the Heteroskedasticity-Robust t-Statistic
Distribution of \(\hat{\beta}\)1 with Normal Errors
18.5 Weighted Least SquaresDistribution of the Homoskedasticity-Only t-Statistic
WLS with Known Heteroskedasticity
WLS with Heteroskedasticity of Known Functional Form Heteroskedasticity-Robust Standard Errors or WLS? APPENDIX 18.1 The Normal and Related Distributions and Moments of Continuous Random Variables APPENDIX 18.2 Two Inequalities CHAPTER 19 The Theory of Multiple Regression
19.1 The Linear Multiple Regression Model and OLS Estimator in Matrix Form
The Multiple Regression Model in Matrix Notation
19.2 Asymptotic Distribution of the OLS Estimator and t-StatisticThe Extended Least Squares Assumptions The OLS Estimator
The Multivariate Central Limit Theorem
19.3 Tests of Joint HypothesesAsymptotic Normality of \(\hat{\beta}\) Heteroskedasticity-Robust Standard Errors Confidence Intervals for Predicted Effects Asymptotic Distribution of the t-Statistic
Joint Hypotheses in Matrix Notation
19.4 Distribution of Regression Statistics with Normal ErrorsAsymptotic Distribution of the F-Statistic Confidence Sets for Multiple Coefficients
Matrix Representations of OLS Regression Statistics
19.5 Efficiency of the OLS Estimator with Homoskedastic ErrorsDistribution of \(\hat{\beta}\) for Normal Errors Distribution of s2û Homoskedasticity-Only Standard Errors Distribution of the t-Statistic Distribution of the F-Statistic
The Gauss–Markov Conditions for Multiple Regression
19.6 Generalized Least SquaresLinear Conditionally Unbiased Estimators The Gauss–Markov Theorem for Multiple Regression
The GLS Assumptions
19.7 Instrumental Variables and Generalized Method of Moments EstimationGLS When Ω Is Known GLS When Ω Contains Unknown Parameters The Conditional Mean Zero Assumption and GLS
The IV Estimator in Matrix Form
Asymptotic Distribution of the TSLS Estimator Properties of TSLS When the Errors are Homoskedastic Generalized Method of Moments Estimation in Linear Models APPENDIX 19.1 Summary of Matrix Algebra APPENDIX 19.2 Multivariate Distributions APPENDIX 19.3 Derivation of the Asymptotic Distribution of \(\hat{\beta}\) APPENDIX 19.4 Derivations of Exact Distributions of OLS Test Statistics with Normal Errors APPENDIX 19.5 Proof of the Gauss–Markov Theorem for Multiple Regression APPENDIX 19.6 Proof of Selected Results for IV and GMM Estimation APPENDIX 19.7 Regression with Many Predictors: MSPE, Ridge Regression, and Principal Components Analysis Appendix
References
Glossary
Index
|
Learn
Free webinars
NetCourses
Classroom and web training
Organizational training
Video tutorials
Third-party courses
Web resources
Teaching with Stata
© Copyright 1996–2025 StataCorp LLC. All rights reserved.
×
We use cookies to ensure that we give you the best experience on our website—to enhance site navigation, to analyze usage, and to assist in our marketing efforts. By continuing to use our site, you consent to the storing of cookies on your device and agree to delivery of content, including web fonts and JavaScript, from third party web services.
Cookie Settings
Last updated: 16 November 2022
StataCorp LLC (StataCorp) strives to provide our users with exceptional products and services. To do so, we must collect personal information from you. This information is necessary to conduct business with our existing and potential customers. We collect and use this information only where we may legally do so. This policy explains what personal information we collect, how we use it, and what rights you have to that information.
These cookies are essential for our website to function and do not store any personally identifiable information. These cookies cannot be disabled.
This website uses cookies to provide you with a better user experience. A cookie is a small piece of data our website stores on a site visitor's hard drive and accesses each time you visit so we can improve your access to our site, better understand how you use our site, and serve you content that may be of interest to you. For instance, we store a cookie when you log in to our shopping cart so that we can maintain your shopping cart should you not complete checkout. These cookies do not directly store your personal information, but they do support the ability to uniquely identify your internet browser and device.
Please note: Clearing your browser cookies at any time will undo preferences saved here. The option selected here will apply only to the device you are currently using.