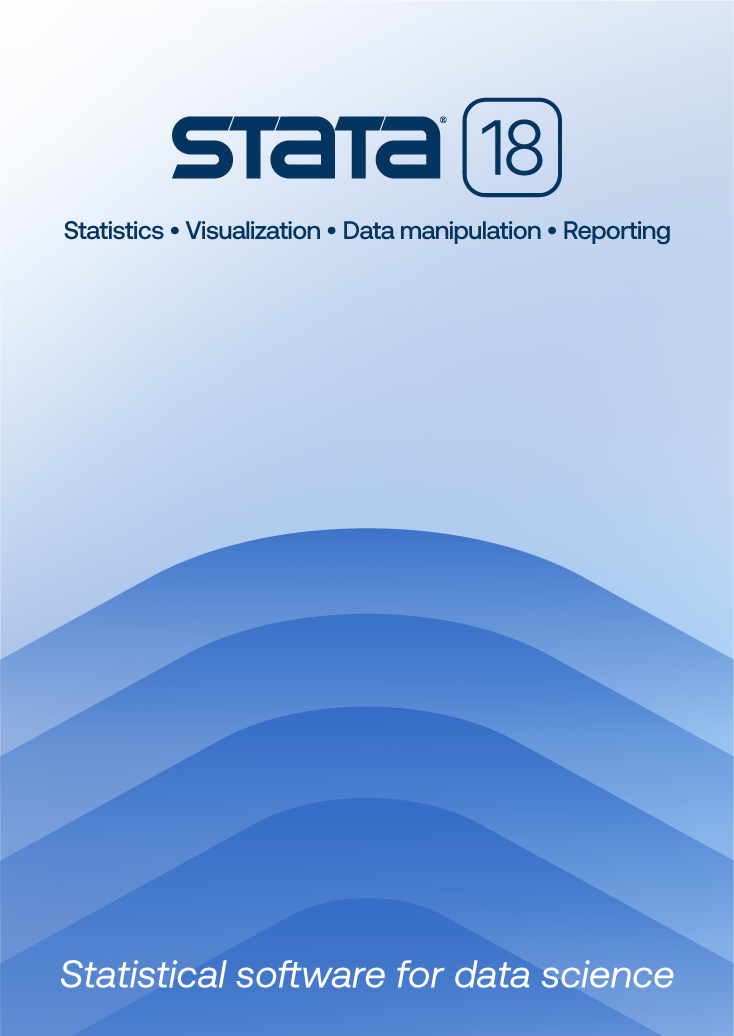
Introductory Econometrics: A Modern Approach, Seventh Edition |
||||||||||||||||||||||||||||||||
![]() Click to enlarge See the back cover |
![]()
As an Amazon Associate, StataCorp earns a small referral credit from
qualifying purchases made from affiliate links on our site.
eBook not available for this title
eBook not available for this title |
|
||||||||||||||||||||||||||||||
Comment from the Stata technical groupThe seventh edition of Jeffrey Wooldridge's textbook Introductory Econometrics: A Modern Approach lives up to its subtitle in its choice of topics and its treatment of standard material. Wooldridge recognizes that modern econometrics involves much more than ordinary least squares (OLS) with a few extensions to handle the special cases commonly encountered in econometric data. In addition to chapters on OLS, he includes chapters on current techniques of estimation and inference for time-series data, panel data, limited dependent variables, and sample selection. In his treatments of OLS and two-stage least squares, Wooldridge breaks new ground by concentrating on advanced statistical concepts instead of matrix algebra. A traditional approach to introductory econometrics would use advanced sections to explain matrix algebra and its applications in econometrics. In contrast, Wooldridge uses the advanced sections of his text to introduce recently developed statistical concepts and techniques. This approach leads to a text with greater breadth than is usual in books of this type. This book is equally useful for advanced undergraduate study, as the basis of a survey course at the graduate level, or as a conceptual supplement to advanced courses. The seventh edition introduces discrete covariates and the modern potential- outcome approach to causal inference earlier and more accessibly in the new sections 2.7, 3.7, and 4.7 and in the improved section 7.6. The new subsections 13.2(a) and 13.2(b) clearly explain the difference-in-differences approach to causal inference. The result is that an excellent introductory book has been made even better. |
||||||||||||||||||||||||||||||||
Table of contentsView table of contents >> Preface
About the Author
Chapter 1 The Nature of Econometrics and Economic Data
1-1 What Is Econometrics?
1-2 Steps in Empirical Economic Analysis 1-3 The Structure of Economic Data
1-3a Cross-Sectional Data
1-4 Causality, Ceteris Paribus, and Counterfactual Reasoning 1-3b Time Series Data 1-3c Pooled Cross Sections 1-3d Panel or Longitudinal Data 1-3e A Comment on Data Structures Summary Key Terms Problems Computer Exercises PART 1 Regression Analysis with Cross-Sectional Data
Chapter 2 The Simple Regression Model
2-1 Definition of the Simple Regression Model
2-2 Deriving the Ordinary Least Squares Estimates
2-2a A Note on Terminology
2-3 Properties of OLS on Any Sample of Data
2-3a Fitted Values and Residuals
2-4 Units of Measurement and Functional Form
2-3b Algebraic Properties of OLS Statistics 2-4c Goodness-of-Fit
2-4a The Effects of Changing Units of Measurement on OLS Statistics
2-5 Expected Values and Variances of the OLS Estimators
2-4b Incorporating Nonlinearities in Simple Regression 2-4c The Meaning of "Linear" Regression
2-5a Unbiasedness of OLS
2-6 Regression through the Origin and Regression on a Constant 2-5b Variances of the OLS Estimators 2-5c Estimating the Error Variance 2-7 Regression on a Binary Explanatory Variable
2-7a Counterfactual Outcomes, Causality, and Policy Analysis
Summary Key Terms Problems Computer Exercises Chapter 3 Multiple Regression Analysis: Estimation
3-1 Motivation for Multiple Regression
3-1a The Model with Two Independent Variables
3-2 Mechanics and Interpretation of Ordinary Least Squares
3-1b The Model with k Independent Variables
3-2a Obtaining the OLS Estimates
3-3 The Expected Value of the OLS Estimators
3-2b Interpreting the OLS Regression Equation 3-2c On the Meaning of "Holding Other Factors Fixed" in Multiple Regression 3-2d Changing More Than One Independent Variable Simultaneously 3-2e OLS Fitted Values and Residuals 3-2f A "Partialling Out" Interpretation of Multiple Regression 3-2g Comparison of Simple and Multiple Regression Estimates 3-2h Goodness-of-Fit 3-2i Regression through the Origin
3-3a Including Irrelevant Variables in a Regression Model
3-4 The Variance of the OLS Estimators
3-3b Omitted Variable Bias: The Simple Case 3-3c Omitted Variable Bias: More General Cases
3-4a The Components of the OLS Variances: Multicollinearity
3-5 Efficiency of OLS: The Gauss-Markov Theorem 3-4b Variances in Misspecified Models 3-4c Estimating σ2: Standard Errors of the OLS Estimators 3-6 Some Comments on the Language of Multiple Regression Analysis 3-7 Several Scenarios for Applying Multiple Regression
3-7a Prediction
3-7b Efficient Markets 3-7c Measuring the Tradeoff between Two Variables 3-7d Testing for Ceteris Paribus Group Differences 3-7e Potential Outcomes, Treatment Effects, and Policy Analysis Summary Key Terms Problems Computer Exercises Chapter 4 Multiple Regression Analysis: Inference
4-1 Sampling Distributions of the OLS Estimators
4-2 Testing Hypotheses about a Single Population Parameter: The t Test
4-2a Testing against One-Sided Alternatives
4-3 Confidence Intervals 4-2b Two-Sided Alternatives 4-2c Testing Other Hypotheses about βj 4-2d Computing p-Values for t Tests 4-2e A Reminder on the Language of Classical Hypothesis Testing 4-2f Economic, or Practical, versus Statistical Significance 4-4 Testing Hypotheses about a Single Linear Combination of the Parameters 4-5 Testing Multiple Linear Restrictions: The F Test
4-5a Testing Exclusion Restrictions
4-6 Reporting Regression Results 4-5b Relationship between F and t Statistics 4-5c The R-Squared Form of the F Statistic 4-5d Computing p-Values for F Tests 4-5e The F Statistic for Overall Significance of a Regression 4-5f Testing General Linear Restrictions 4-7 Revisiting Causal Effects and Policy Analysis Summary Key Terms Problems Computer Exercises Chapter 5 Multiple Regression Analysis: OLS Asymptotics
5-1 Consistency
5-1a Deriving the Inconsistency in OLS
5-2 Asymptotic Normality and Large Sample Inference
5-2a Other Large Sample Tests: The Lagrange Multiplier Statistic
5-3 Asymptotic Efficiency of OLS Summary Key Terms Problems Computer Exercises Chapter 6 Multiple Regression Analysis: Further Issues
6-1 Effects of Data Scaling on OLS Statistics
6-1a Beta Coefficients
6-2 More on Functional Form
6-2a More on Using Logarithmic Functional Forms
6-3 More on Goodness-of-Fit and Selection of Regressors
6-2b Models with Quadratics 6-2c Models with Interaction Terms 6-3d Computing Average Partial Effects
6-3a Adjusted R-Squared
6-4 Prediction and Residual Analysis
6-3b Using Adjusted R-Squared to Choose between Nonnested Models 6-3c Controlling for Too Many Factors in Regression Analysis 6-3d Adding Regressors to Reduce the Error Variance
6-4a Confidence Intervals for Predictions
Summary 6-4b Residual Analysis 6-4c Predicting y When log(y) Is the Dependent Variable 6-4d Predicting y When the Dependent Variable Is log(y): Key Terms Problems Computer Exercises Chapter 7 Multiple Regression Analysis with Qualitative Information
7-1 Describing Qualitative Information
7-2 A Single Dummy Independent Variable
7-2a Interpreting Coefficients on Dummy Explanatory Variables When the Dependent Variable Is log(y)
7-3 Using Dummy Variables for Multiple Categories
7-3a Incorporating Ordinal Information by Using Dummy Variables
7-4 Interactions Involving Dummy Variables
7-4a Interactions among Dummy Variables
7-5 A Binary Dependent Variable: The Linear Probability Model 7-4b Allowing for Different Slopes 7-4c Testing for Differences in Regression Functions across Groups 7-6 More on Policy Analysis and Program Evaluation
7-6a Program Evaluation and Unrestricted Regression Adjustment
7-7 Interpreting Regression Results with Discrete Dependent Variables Summary Key Terms Problems Computer Exercises Chapter 8 Heteroskedasticity
8-1 Consequences of Heteroskedasticity for OLS
8-2 Heteroskedasticity-Robust Inference after OLS Estimation
8-2a Computing Heteroskedasticity-Robust LM Tests
8-3 Testing for Heteroskedasticity
8-3a The White Test for Heteroskedasticity
8-4 Weighted Least Squares Estimation
8-4a The Heteroskedasticity Is Known up to a Multiplicative Constant
8-5 The Linear Probability Model Revisited 8-4b The Heteroskedasticity Function Must Be Estimated: Feasible GLS 8-4c What If the Assumed Heteroskedasticity Function Is Wrong? 8-4d Prediction and Prediction Intervals with Heteroskedasticity Summary Key Terms Problems Computer Exercises Chapter 9 More on Specification and Data Issues
9-1 Functional Form Misspecification
9-1a RESET as a General Test for Functional Form Misspecification
9-2 Using Proxy Variables for Unobserved Explanatory Variables
9-1b Tests against Nonnested Alternatives
9-2a Using Lagged Dependent Variables as Proxy Variables
9-3 Models with Random Slopes 9-2b A Different Slant on Multiple Regression 9-2c Potential Outcomes and Proxy Variables 9-4 Properties of OLS under Measurement Error
9-4a Measurement Error in the Dependent Variable
9-5 Missing Data, Nonrandom Samples, and Outlying Observations
9-4b Measurement Error in an Explanatory Variable
9-5a Missing Data
9-6 Least Absolute Deviations Estimation 9-5b Nonrandom Samples 9-5c Outliers and Influential Observations Summary Key Terms Problems Computer Exercises Part 2 Regression Analysis with Time Series Data
Chapter 10 Basic Regression Analysis with Time Series Data
10-1 The Nature of Time Series Data
10-2 Examples of Time Series Regression Models
10-2a Static Models
10-3 Finite Sample Properties of OLS under Classical Assumptions
10-2b Finite Distributed Lag Models 10-2c A Convention about the Time Index
10-3a Unbiasedness of OLS
10-4 Functional Form, Dummy Variables, and Index Numbers 10-3b The Variances of the OLS Estimators and the Gauss-Markov Theorem 10-3c Inference under the Classical Linear Model Assumptions 10-5 Trends and Seasonality
10-5a Characterizing Trending Time Series
Summary 10-5b Using Trending Variables in Regression Analysis 10-5c A Detrending Interpretation of Regressions with a Time Trend 10-5d Computing R-Squared When the Dependent Variable Is Trending 10-5e Seasonality Key Terms Problems Computer Exercises Chapter 11 Further Issues in Using OLS with Time Series Data
11-1 Stationary and Weakly Dependent Time Series
11-1a Stationary and Nonstationary Time Series
11-2 Asymptotic Properties of OLS 11-1b Weakly Dependent Time Series 11-3 Using Highly Persistent Time Series in Regression Analysis
11-3a Highly Persistent Time Series
11-4 Dynamically Complete Models and the Absence of Serial Correlation 11-3b Transformations on Highly Persistent Time Series 11-3c Deciding Whether a Time Series Is I(1) 11-5 The Homoskedasticity Assumption for Time Series Models Summary Key Terms Problems Computer Exercises Chapter 12 Serial Correlation and Heteroskedasticity in Time Series Regressions
12-1 Properties of OLS with Serially Correlated Errors
12-1a Unbiasedness and Consistency
12-2 Serial Correlation-Robust Inference after OLS 12-1b Efficiency and Inference 12-1c Goodness-of-Fit 12-1d Serial Correlation in the Presence of Lagged Dependent Variables 12-3 Testing for Serial Correlation 12-3a A t Test for AR(1) Serial Correlation with Strictly Exogenous Regressors 12-3b The Durbin-Watson Test under Classical Assumptions 12-3c Testing for AR(1) Serial Correlation without Strictly Exogenous Regressors 12-3d Testing for Higher-Order Serial Correlation 12-4 Correcting for Serial Correlation with Strictly Exogenous Regressors
12-4a Obtaining the Best Linear Unbiased Estimator in the AR(1) Model
12-5 Differencing and Serial Correlation 12-4b Feasible GLS Estimation with AR(1) Errors 12-4c Comparing OLS and FGLS 12-4d Correcting for Higher-Order Serial Correlation 12-4e What if the Serial Correlation Models Is Wrong? 12-6 Heteroskedasticity in Time Series Regressions
12-6a Heteroskedasticity-Robust Statistics
Summary 12-6b Testing for Heteroskedasticity 12-6c Autoregressive Conditional Heteroskedasticity 12-6d Heteroskedasticity and Serial Correlation in Regression Models Key Terms Problems Computer Exercises Part 3 Advanced Topics
Chapter 13 Pooling Cross Sections across Time: Simple Panel Data Methods
13-1 Pooling Independent Cross Sections across Time
13-1a The Chow Test for Structural Change across Time
13-2 Policy Analysis with Pooled Cross Sections
13-2a Adding an Additional Control Group
13-3 Two-Period Panel Data Analysis
13-2b A General Framework for Policy Analysis with Pooled Cross Sections
13-3a Organizing Panel Data
13-4 Policy Analysis with Two-Period Panel Data 13-5 Differencing with More Than Two Time Periods
13-5a Potential Pitfalls in First Differencing Panel Data
Summary Key Terms Problems Computer Exercises Chapter 14 Advanced Panel Data Methods
14-1 Fixed Effects Estimation
14-1a The Dummy Variable Regression
14-2 Random Effects Models
14-1b Fixed Effects or First Differencing? 14-1c Fixed Effects with Unbalanced Panels
14-2a Random Effects of Pooled OLS?
14-3 The Correlated Random Effects Approach
14-2b Random Effects or Fixed Effects?
14-3a Unbalanced Panels
14-4 General Policy Analysis with Panel Data
14-4a Advanced Considerations with Policy Analysis
14-5 Applying Panel Data Methods to Other Data Structures Summary Key Terms Problems Computer Exercises Chapter 15 Instrumental Variables Estimation and Two-Stage Least Squares
15-1 Motivation: Omitted Variables in a Simple Regression Model
15-1a Statistical Inference with the IV Estimator
15-2 IV Estimation of the Multiple Regression Model 15-1b Properties of IV with a Poor Instrumental Variable 15-1c Computing R-Squared after IV Estimation 15-3 Two-Stage Least Squares
15-3a A Single Endogenous Explanatory Variable
15-4 IV Solutions to Errors-in-Variables Problems 15-3b Multicollinearity and 2SLS 15-3c Detecting Weak Instruments 15-3d Multiple Endogenous Explanatory Variables 15-3e Testing Multiple Hypotheses after 2SLS Estimation 15-5 Testing for Endogeneity and Testing Overidentifying Restrictions
15-5a Testing for Endogeneity
15-6 2SLS with Heteroskedasticity 15-5b Testing Overidentification Restrictions 15-7 Applying 2SLS to Time Series Equations 15-8 Applying 2SLS to Pooled Cross Sections and Panel Data Summary Key Terms Problems Computer Exercises Chapter 16 Simultaneous Equations Models
16-1 The Nature of Simultaneous Equations Models
16-2 Simultaneity Bias in OLS 16-3 Identifying and Estimating a Structural Equation
16-3a Identification in a Two-Equation System
16-4 Systems with More Than Two Equations
16-3b Estimation by 2SLS
16-4a Identification in Systems with Three or More Equations
16-5 Simultaneous Equations Models with Time Series 16-4b Estimation 16-6 Simultaneous Equations Models with Panel Data Summary Key Terms Problems Computer Exercises Chapter 17 Limited Dependent Variable Models and Sample Selection Corrections
17-1 Logit and Probit Models for Binary Response
17-1a Specifying Logit and Probit Models
17-2 The Tobit Model for Corner Solution Responses
17-1b Maximum Likelihood Estimation of Logit and Probit Models 17-1c Testing Multiple Hypotheses 17-1d Interpreting the Logit and Probit Estimates
17-2a Interpreting the Tobit Estimates
17-3 The Poisson Regression Model 17-2b Specification Issues in Tobit Models 17-4 Censored and Truncated Regression Models
17-4a Censored Regression Models
17-5 Sample Selection Corrections
17-4b Truncated Regression Models
17-5a When Is OLS on the Selected Sample Consistent?
Summary 17-5b Incidental Truncation Key Terms Problems Computer Exercises Chapter 18 Advanced Time Series Topics
18-1 Infinite Distributed Lag Models
18-1a The Geometric (or Koyck) Distributed Lag Model
18-2 Testing for Unit Roots 18-1b Rational Distributed Lag Models 18-3 Spurious Regression 18-4 Cointegration and Error Correction Models
18-4a Cointegration
18-5 Forecasting
18-4b Error Correction Models
18-5a Types of Regression Models Used for Forecasting
Summary 18-5b One-Step-Ahead Forecasting 18-5c Comparing One-Step-Ahead Forecasts 18-5d Multiple-Step-Ahead Forecasts 18-5e Forecasting Trending, Seasonal, and Integrated Processes Key Terms Problems Computer Exercises Chapter 19 Carrying Out an Empirical Project
19-1 Posing a Question
19-2 Literature Review 19-3 Data Collection
19-3a Deciding on the Appropriate Data Set
19-4 Econometric Analysis 19-3b Entering and Storing Your Data 19-3c Inspecting, Cleaning, and Summarizing Your Data 19-5 Writing an Empirical Paper
19-5a Introduction
Summary 19-5b Conceptual (or Theoretical) Framework 19-5c Econometric Models and Estimation Methods 19-5d The Data 19-5e Results 19-5f Conclusions 19-5g Style Hints Key Terms Sample Empirical Projects List of Journals Data Sources MATH REFRESHER A Basic Mathematical Tools
A-1 The Summation Operator and Descriptive Statistics
A-2 Properties of Linear Functions A-3 Proportions and Percentages A-4 Some Special Functions and Their Properties
A-4a Quadratic Functions
A-5 Differential Calculus A-4b The Natural Logarithm A-4c The Exponential Function Summary Key Terms Problems MATH REFRESHER B Fundamentals of Probability
B-1 Random Variables and Their Probability Distributions
B-1a Discrete Random Variables
B-2 Joint Distributions, Conditional Distributions, and Independence
B-1b Continuous Random Variables
B-2a Joint Distributions and Independence
B-3 Features of Probability Distributions
B-2b Conditional Distributions
B-3a A Measure of Central Tendency: The Expected Value
B-4 Features of Joint and Conditional Distributions
B-3b Properties of Expected Values B-3c Another Measure of Central Tendency: The Median B-3d Measures of Variability: Variance and Standard Deviation B-3e Variance B-3f Standard Deviation B-3g Standardizing a Random Variable B-3h Skewness and Kurtosis
B-4a Measures of Association: Covariance and Correlation
B-5 The Normal and Related Distributions
B-4b Covariance B-4c Correlation Coefficient B-4d Variance of Sums of Random Variables B-4e Conditional Expectation B-4f Properties of Conditional Expectation B-4g Conditional Variance
B-5a The Normal Distribution
Summary B-5b The Standard Normal Distribution B-5c Additional Properties of the Normal Distribution B-5d The Chi-Square Distribution B-5e The t Distribution B-5f The F Distribution Key Terms Problems MATH REFRESHER C Fundamentals of Mathematical Statistics
C-1 Populations, Parameters, and Random Sampling
C-1a Sampling
C-2 Finite Sample Properties of Estimators
C-2a Estimators and Estimates
C-3 Asymptotic or Large Sample Properties of Estimators
C-2b Unbiasedness C-2c The Sampling Variance of Estimators C-2d Efficiency
C-3a Consistency
C-4 General Approaches to Parameter Estimation
C-3b Asymptotic Normality
C-4a Method of Moments
C-5 Interval Estimation and Confidence Intervals
C-4b Maximum Likelihood C-4c Least Squares
C-5a The Nature of Interval Estimation
C-6 Hypothesis Testing
C-5b Confidence Intervals for the Mean from a Normally Distributed Population C-5c A Simple Rule of Thumb for a 95% Confidence Interval C-5d Asymptotic Confidence Intervals for Nonnormal Populations
C-6a Fundamentals of Hypothesis Testing
C-7 Remarks on Notation C-6b Testing Hypotheses about the Mean in a Normal Population C-6c Asymptotic Tests for Nonnormal Populations C-6d Computing and Using p-Values C-6e The Relationship between Confidence Intervals and Hypothesis Testing C-6f Practical versus Statistical Significance Summary Key Terms Problems ADVANCED TREATMENT D Summary of Matrix Algebra
D-1 Basic Definitions
D-2 Matrix Operations
D-2a Matrix Addition
D-3 Linear Independence and Rank of a Matrix D-2b Scalar Multiplication D-2c Matrix Multiplication D-2d Transpose D-2e Partitioned Matrix Multiplication D-2f Trace D-2g Inverse D-4 Quadratic Forms and Positive Definite Matrices D-5 Idempotent Matrices D-6 Differentiation of Linear and Quadratic Forms D-7 Moments and Distributions of Random Vectors
D-7a Expected Value
Summary D-7b Variance-Covariance Matrix D-7c Multivariate Normal Distribution D-7d Chi-Square Distribution D-7e t Distribution D-7f F Distribution Key Terms Problems ADVANCED TREATMENT E The Linear Regression Model in Matrix Form
E-1 The Model and Ordinary Least Squares Estimation
E-1a The Frisch-Waugh Theorem
E-2 Finite Sample Properties of OLS E-3 Statistical Inference E-4 Some Asymptotic Analysis
E-4a Wald Statistics for Testing Multiple Hypotheses
Summary Key Terms Problems Answers to Going Further Questions Statistical Tables References Glossary Index |
Learn
Free webinars
NetCourses
Classroom and web training
Organizational training
Video tutorials
Third-party courses
Web resources
Teaching with Stata
© Copyright 1996–2025 StataCorp LLC. All rights reserved.
×
We use cookies to ensure that we give you the best experience on our website—to enhance site navigation, to analyze usage, and to assist in our marketing efforts. By continuing to use our site, you consent to the storing of cookies on your device and agree to delivery of content, including web fonts and JavaScript, from third party web services.
Cookie Settings
Last updated: 16 November 2022
StataCorp LLC (StataCorp) strives to provide our users with exceptional products and services. To do so, we must collect personal information from you. This information is necessary to conduct business with our existing and potential customers. We collect and use this information only where we may legally do so. This policy explains what personal information we collect, how we use it, and what rights you have to that information.
These cookies are essential for our website to function and do not store any personally identifiable information. These cookies cannot be disabled.
This website uses cookies to provide you with a better user experience. A cookie is a small piece of data our website stores on a site visitor's hard drive and accesses each time you visit so we can improve your access to our site, better understand how you use our site, and serve you content that may be of interest to you. For instance, we store a cookie when you log in to our shopping cart so that we can maintain your shopping cart should you not complete checkout. These cookies do not directly store your personal information, but they do support the ability to uniquely identify your internet browser and device.
Please note: Clearing your browser cookies at any time will undo preferences saved here. The option selected here will apply only to the device you are currently using.